PASPP Medical Transformer for Medical Image Segmentation
Proceedings of International Conference on Data Science and ApplicationsLecture Notes in Networks and Systems(2023)
摘要
Medical Transformer (MedT) has recently attracted much attention in medical segmentation as it could perform global context of the image and can work well even with small datasets. However, there are some limitations of MedT such as the big disparity between the information of the encoder and the decoder, the low resolution of input images to effectively execute, and the lack of ability to recognize contextual information in multiple scales. To address such issues, in this study, we propose an architecture that employs progressive atrous spatial pyramid pooling (PASPP) to the MedT architecture, and pointwise atrous convolution layers instead of AvgPooling layers in MedT to make robust pooling operations. In addition, we also change the convolution stem of MedT to help the model to accept a higher resolution of input with the same computational complexity. The proposed model is evaluated on two medical image segmentation datasets including the Glas and Data science bowls 2018. Experiment results show that the proposed approach outperforms other state of the arts.
更多查看译文
关键词
image segmentation,transformer
AI 理解论文
溯源树
样例
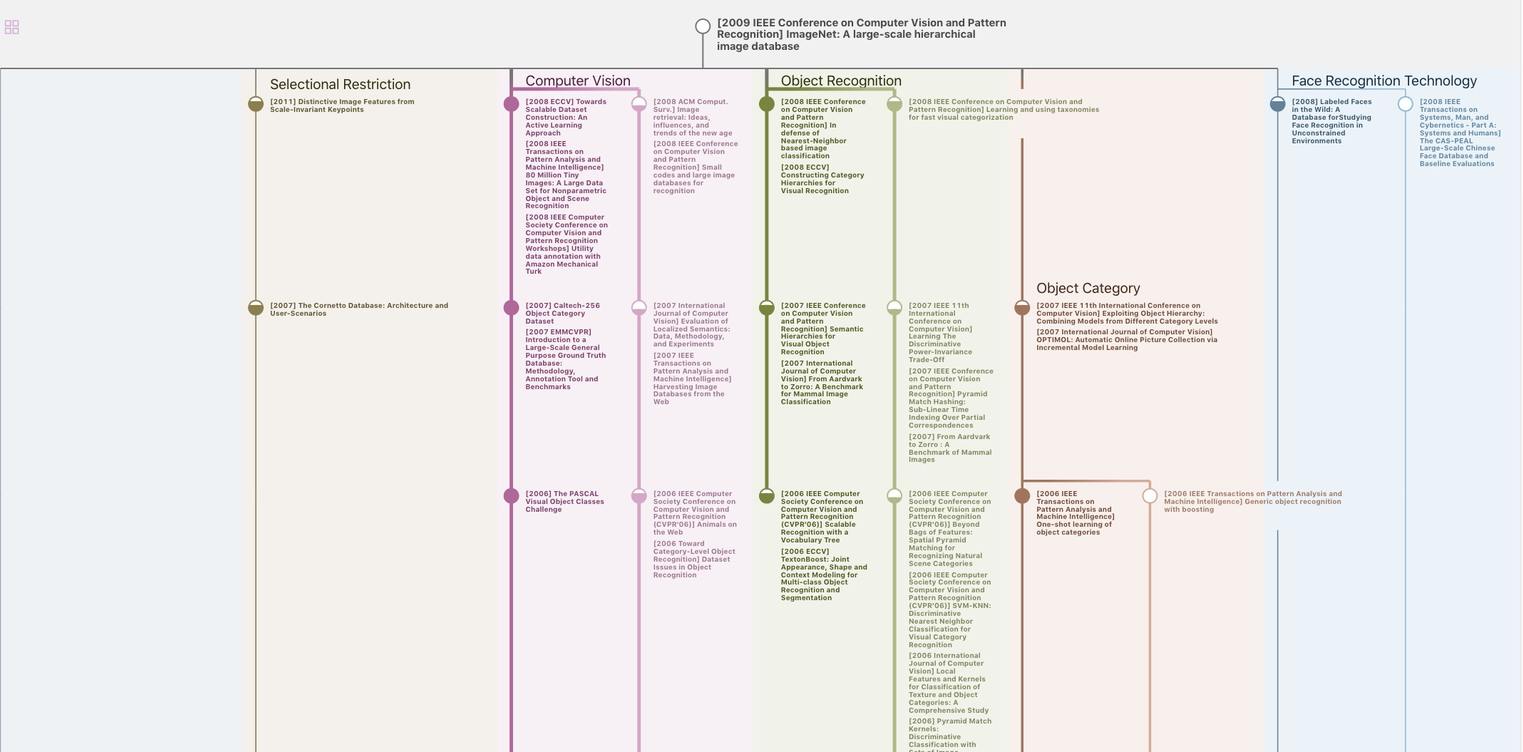
生成溯源树,研究论文发展脉络
Chat Paper
正在生成论文摘要