A New Quantum-Inspired Salp Swarm Optimization Algorithm for Dynamic Optimization Problem
2022 IEEE 19th India Council International Conference (INDICON)(2022)
摘要
Salp Swarm Optimization (SSO) is designed to solve various stationary optimization problems, including feature selection, neural network learning, and the controller placement problem. Several real-world applications are dynamic optimization problems (DOPs) that cannot be solved similarly to stationary optimization problems. While the SSO approach has been applied to solve many real-world optimization problems, this approach still has difficulty in identifying the optima in the dynamic and uncertain environment. It is mainly because of its original design and formulation, a consistent movement towards global optimum, and diversity losses during the dynamic optimization process. This article attempts to overcome this problem for the first time by integrating the principles of quantum computing in SSO’s equations for dynamic optimization problems. We tried to hybridize two novel paradigms for addressing issues in dynamic and uncertain environments, Viz., Salp Swarm Optimization (SSO), and the principles of quantum mechanics. An intelligent shifting operator is designed to conform to the evolutional individuals for the current environmental state. Principles of quantum computing and a newly designed operator improve SSO’s diversity and search capabilities by finding an optimal compromise between exploration and exploitation. The outcome of the proposed algorithm is evaluated on the Six Generalized Dynamic Benchmarking (GDBG) problems and compared with the results of several cutting-edge algorithms. The experimental results indicate that the performance of the Quantum-inspired SSO (QSSO) algorithm outperforms as compared to other algorithms in this domain.
更多查看译文
关键词
salp swarm optimization algorithm,dynamic optimization problem,quantum-inspired
AI 理解论文
溯源树
样例
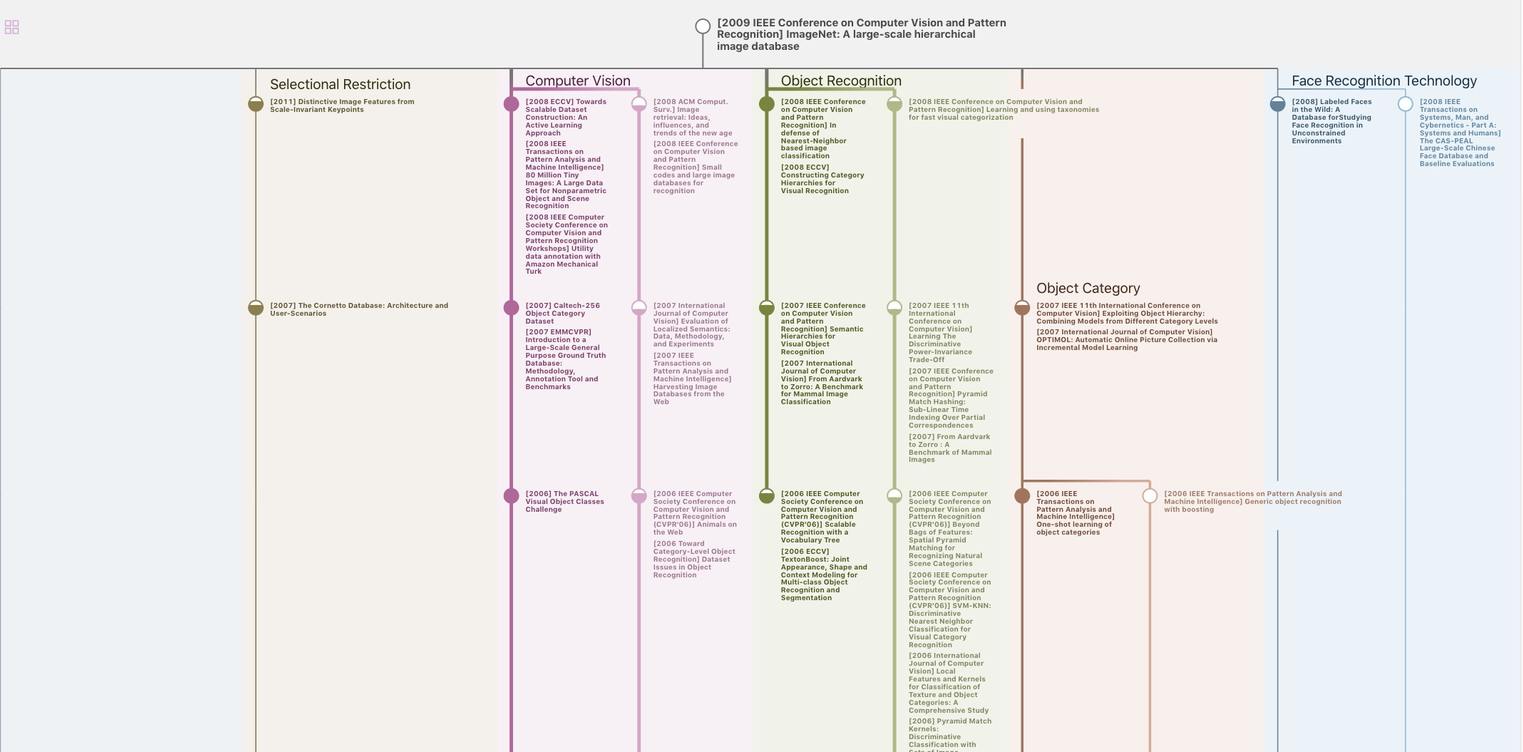
生成溯源树,研究论文发展脉络
Chat Paper
正在生成论文摘要