Acoustic-based automatic speech intelligibility scoring using deep neural networks
The Journal of the Acoustical Society of America(2022)
摘要
Human-generated measures of speech intelligibility are time-intensive methods for assessing the intelligibility of speech. The purpose of the present study is to automate the assessment of speech intelligibility by developing a deep neural network that estimates a standardized intelligibility score based on acoustic input. We extracted Mel-frequency cepstral coefficients from the UW/NU IEEE sentence corpus which had been manipulated with three signal-to-noise ratios (−2, 0, 2 dB). We obtained listener transcriptions from the UAW speech intelligibility dataset and calculated the Levenshtein distance between the transcriptions and the speaker's prompt. The neural network was trained to predict the Levenshtein distance given MFCC representations of sentences. We use tenfold cross-validation to verify the accuracy of the model and investigate the correlation of the model predictions with the average human responses. We also compare our model’s accuracy with the Levenshtein distance generated by transcriptions produced by the DeepSpeech ASR model. This study investigates the reliability of deep neural networks as an alternative to human-based inference in quantifying the intelligibility of speech. We discuss the advantages and disadvantages of the different approaches to assessing speech intelligibility.
更多查看译文
关键词
automatic speech intelligibility scoring,neural networks,acoustic-based
AI 理解论文
溯源树
样例
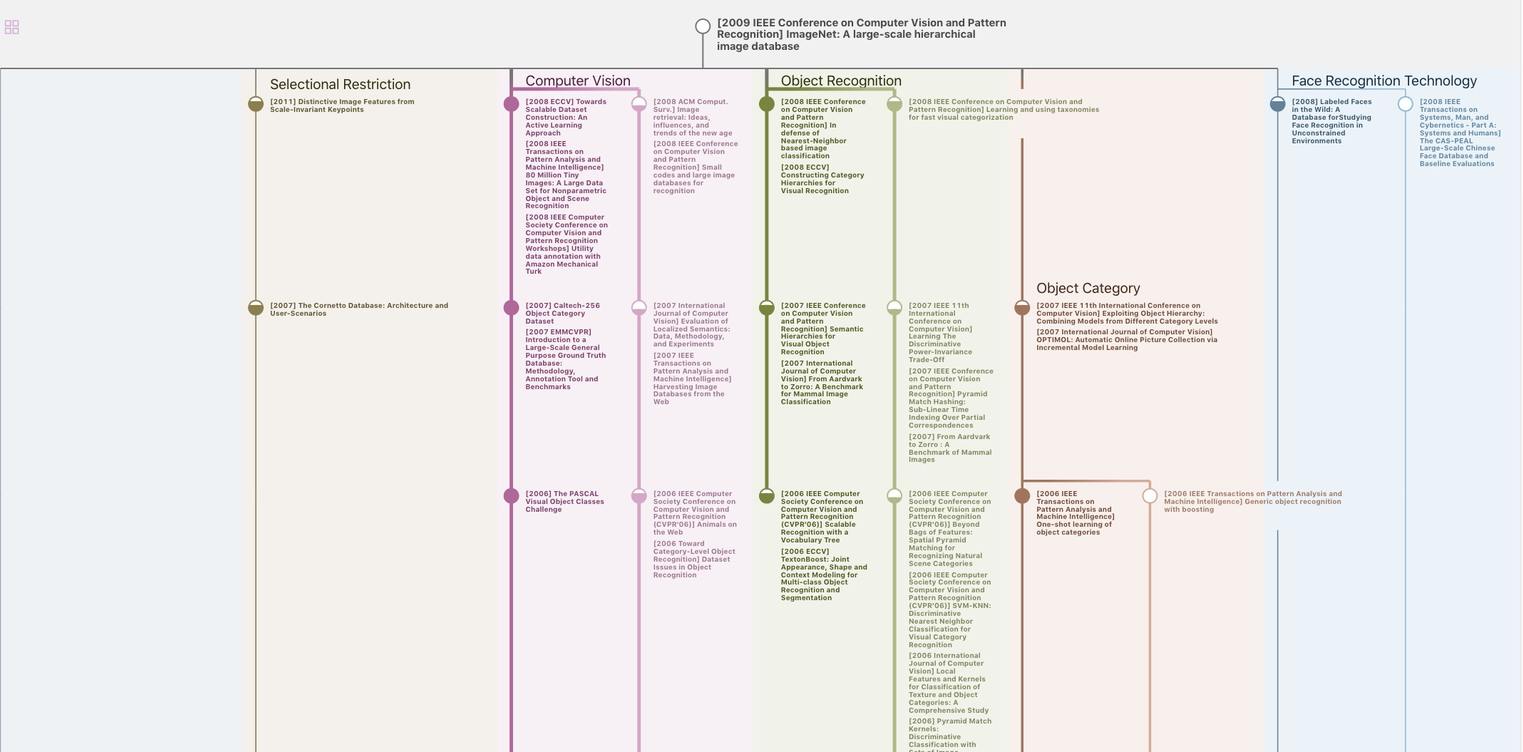
生成溯源树,研究论文发展脉络
Chat Paper
正在生成论文摘要