Deep reinforcement learning-based framework for the design of broadband acoustic metamaterials
The Journal of the Acoustical Society of America(2022)
摘要
This talk presents a general framework for discovering optimal designs of metamaterials with deep reinforcement learning (RL). In our previous research [1], we applied RL to acoustic cloak design and optimized the design parameters of a planar configuration of up to 12 cylindrical scatterers to minimize the scattering of an incident acoustic wave. The designs produced by RL algorithms are comparable and in some cases superior to those produced by fmincon solver. However, the challenge of finding an efficient approach for inverse design, is still in its emergent stage, requiring further modifications of these models and significant computational resources to increase the complexity of a design. This creates a significant challenge when attempting to enable model scaling since training times surge to unfeasible levels. We will discuss methods to counteract this challenge and present an RL-based framework for discovering optimal designs of meta-devices. We expanded our research [1] to large numbers of scatterers, and new designs with variable cylinder radii, position, and material. We noticed a dramatic improvement in the training times of RL agents due to the speed increase provided by the Julia programming language which accelerates episode runtimes 10-times compared to our previous models in python [1]. [1] T. Shah et al., “Reinforcement learning applied to metamaterial design,” J. Acoust. Soc. Am. 150(1), 321–338 (2021).
更多查看译文
关键词
broadband acoustic metamaterials,learning-based
AI 理解论文
溯源树
样例
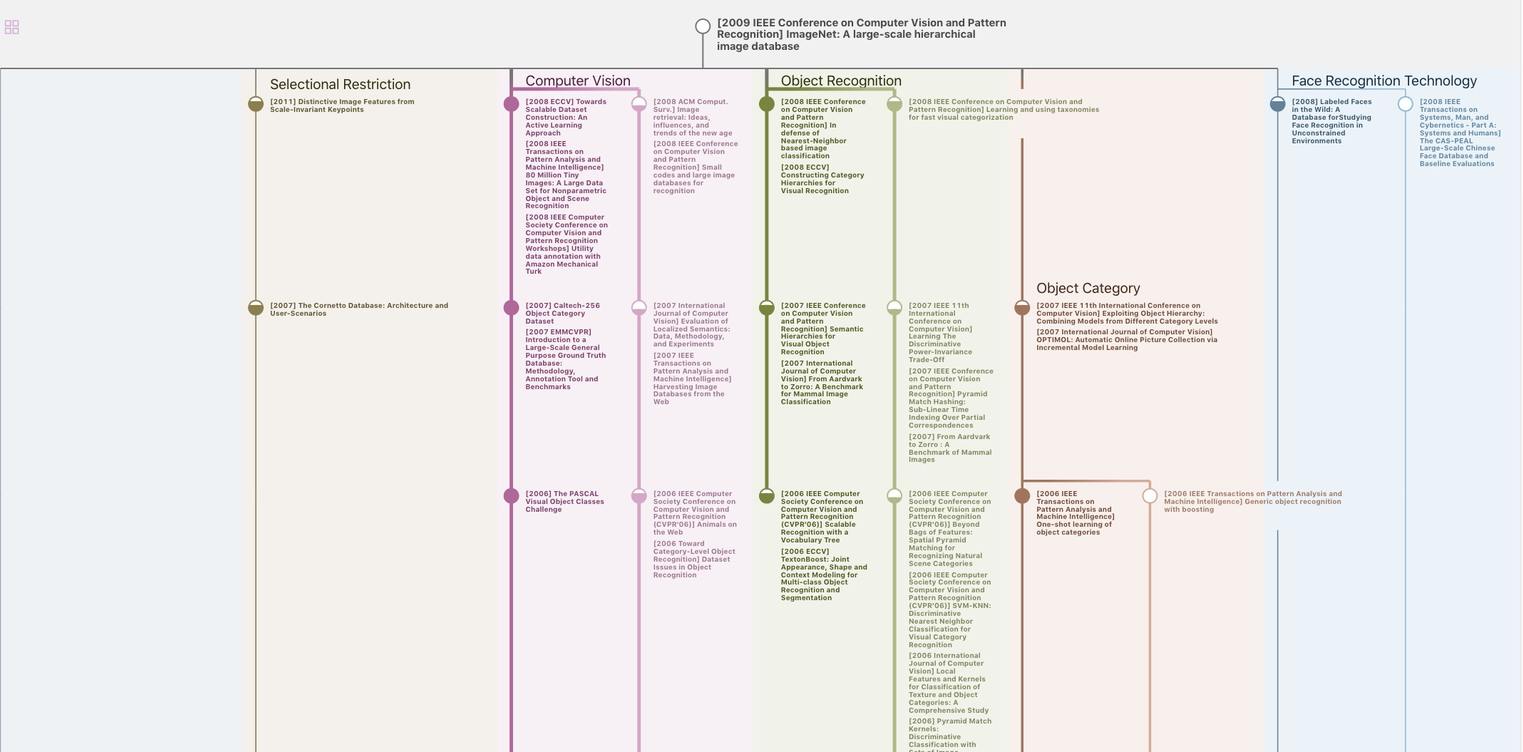
生成溯源树,研究论文发展脉络
Chat Paper
正在生成论文摘要