Improving Performance, Reliability, and Feasibility in Multimodal Multitask Traffic Classification with XAI
IEEE Transactions on Network and Service Management(2023)
摘要
The promise of Deep Learning (DL) in solving hard problems such as network Traffic Classification (TC) is being held back by the severe lack of transparency and explainability of this kind of approaches. To cope with this strongly felt issue, the field of eXplainable Artificial Intelligence (XAI) has been recently founded, and is providing effective techniques and approaches. Accordingly, in this work we investigate interpretability via XAI-based techniques to understand and improve the behavior of state-of-the-art multimodal and multitask DL traffic classifiers. Using a publicly available security-related dataset (ISCX VPN-nonVPN), we explore and exploit XAI techniques to characterize the considered classifiers providing global interpretations (rather than sample-based ones), and define a novel classifier, Distiller-Evolved, optimized along three objectives: performance, reliability, feasibility. The proposed methodology proves as highly appealing, allowing to much simplify the architecture to get faster training time and shorter classification time, as fewer packets must be collected. This is at the expenses of negligible (or even positive) impact on classification performance, while understanding and controlling the interplay between inputs, model complexity, performance, and reliability.
更多查看译文
关键词
Deep learning,encrypted traffic,explainable artificial intelligence,multimodal learning,multitask learning,traffic classification
AI 理解论文
溯源树
样例
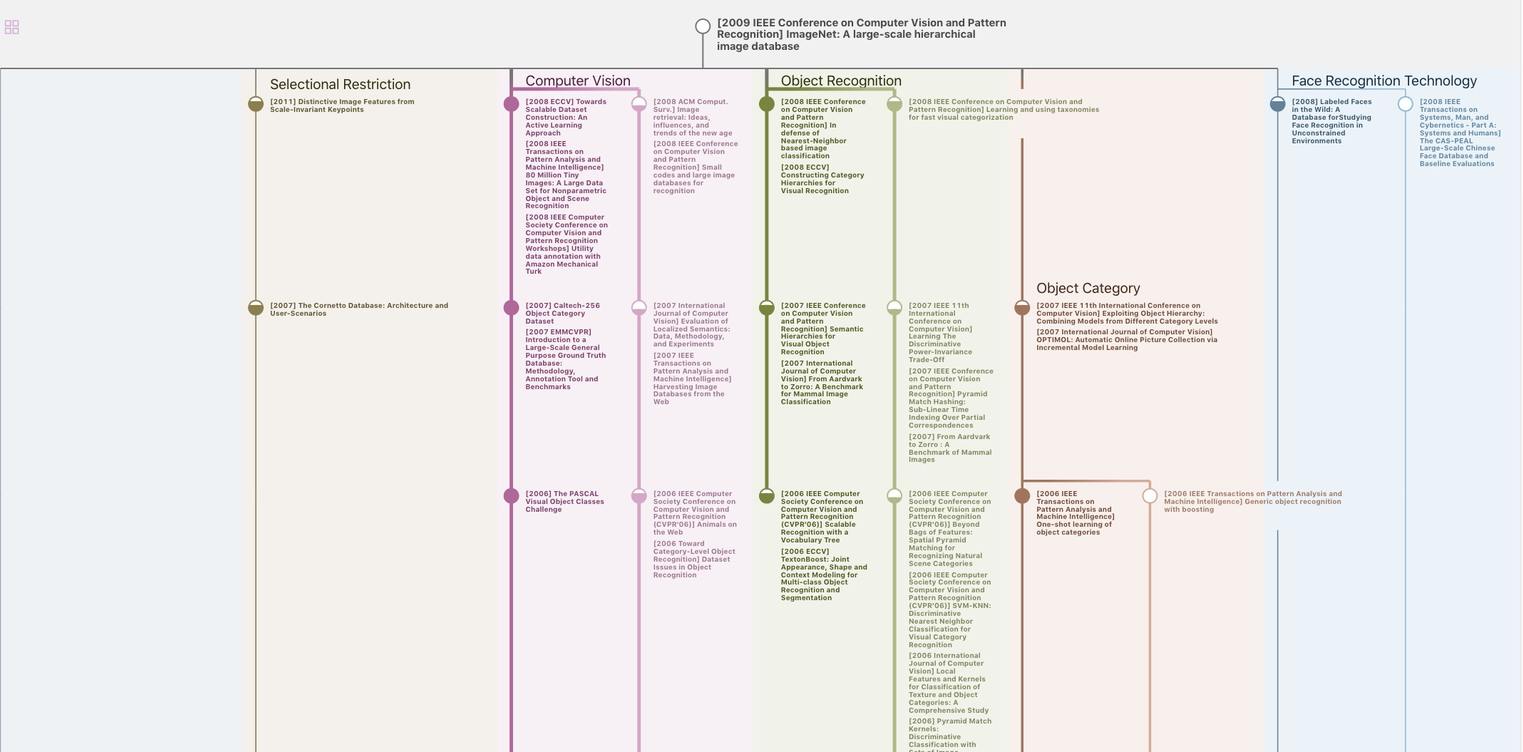
生成溯源树,研究论文发展脉络
Chat Paper
正在生成论文摘要