On the boundary problems in diagnostic classification models
Behaviormetrika(2022)
摘要
In diagnostic classification models, parameter estimation sometimes provides estimates that stick to the boundaries of the parameter space, which is called the boundary problem and may lead to extreme values of standard errors. However, the relationship between the boundary problem and irregular standard errors has not been analytically explored. In addition, prior research has not shown how maximum-a-posteriori estimates avoid the boundary problem and affect the standard errors of estimates. To analyze these relationships, the expectation–maximization algorithm for maximum-a-posteriori estimates and a complete data Fisher information matrix are explicitly derived for a mixture formulation of saturated diagnostic classification models. Theoretical considerations show that the emptiness of attribute mastery patterns causes both the boundary problem and the inaccurate standard error estimates. Furthermore, unfortunate boundary problem without emptiness causes shorter standard errors. A simulation study shows that the maximum-a-posteriori method prevents boundary problems. Moreover, this method with monotonicity constraint estimation improves standard error estimates more than unconstrained maximum likelihood estimates do.
更多查看译文
关键词
Diagnostic classification models,Maximum a posteriori estimation,Expectation maximization algorithm,Standard error
AI 理解论文
溯源树
样例
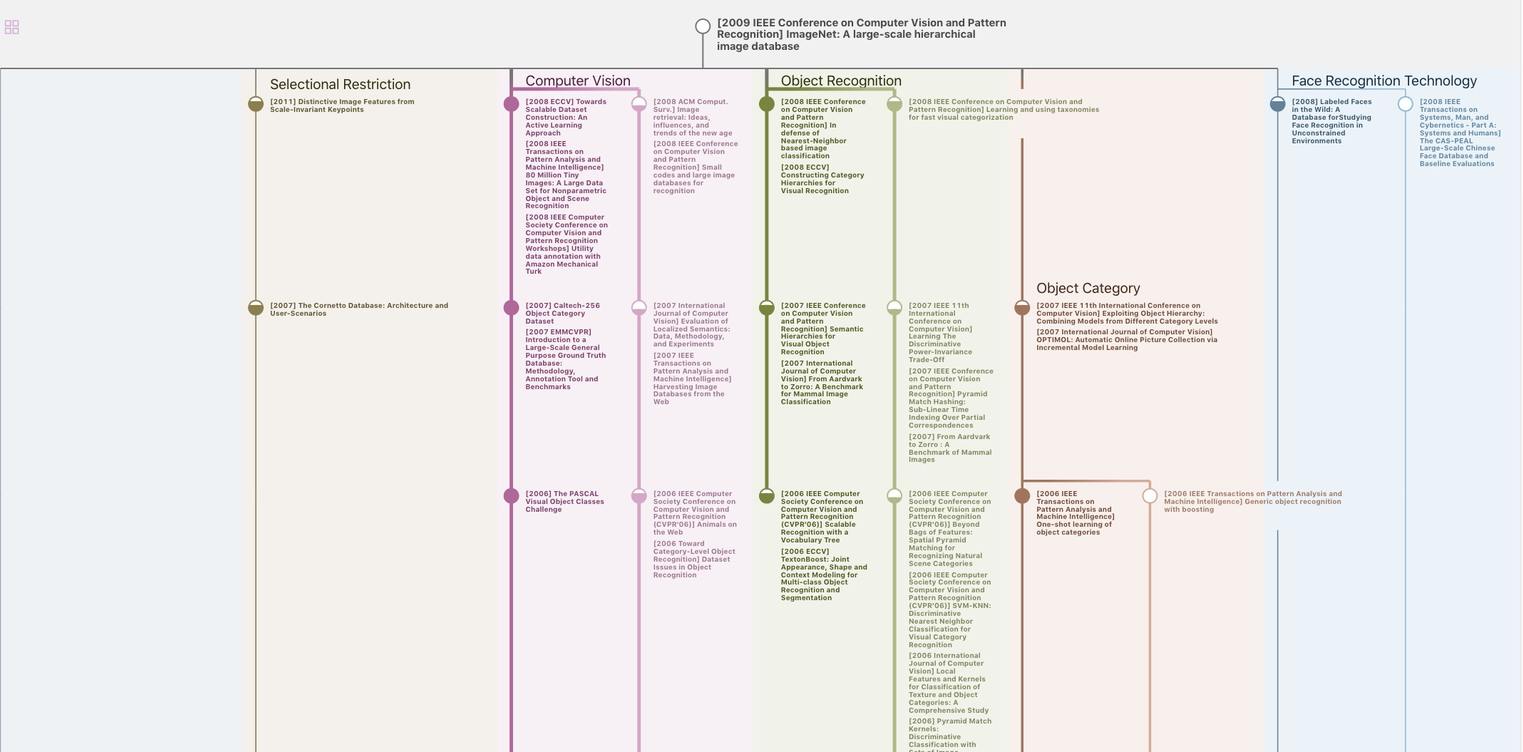
生成溯源树,研究论文发展脉络
Chat Paper
正在生成论文摘要