Hierarchical block aggregation network for long-tailed visual recognition
SSRN Electronic Journal(2023)
摘要
It is usually supposed that training database is manually balanced in traditional visual recognition tasks. However, in nature, data tends to follow long-tailed distributions. In recent years, many plug-and-play methods based on data augmentation or representation learning have been proposed to tackle the long-tailed visual recognition task. Although these methods are effective, we find that when different plug-and-play methods are applied to the same long-tail recognition model, they sometimes fail to promote each other. The reason for this phenomenon may lie in the fact that the overall performance of the model is constrained by the insufficient capability of a traditional feature extractor. Motivated by this fact, we first propose Hierarchical Block Aggregation Network (HBAN), a network structure with stronger feature extraction capability. Then, we design a Quantity-Aware Balanced (QAB) loss and a decoupled training paradigm to optimize HBAN. The effectiveness of HBAN is demonstrated by extensive experiments. In particular, HBAN achieves significant improvements over our baseline on three benchmark datasets, and outperforms the state-of-the-art methods on CIFAR 100-LT.& COPY; 2023 Elsevier B.V. All rights reserved.
更多查看译文
关键词
Long-tailed visual recognition,Hierarchical block aggregation network,Decoupled training paradigm
AI 理解论文
溯源树
样例
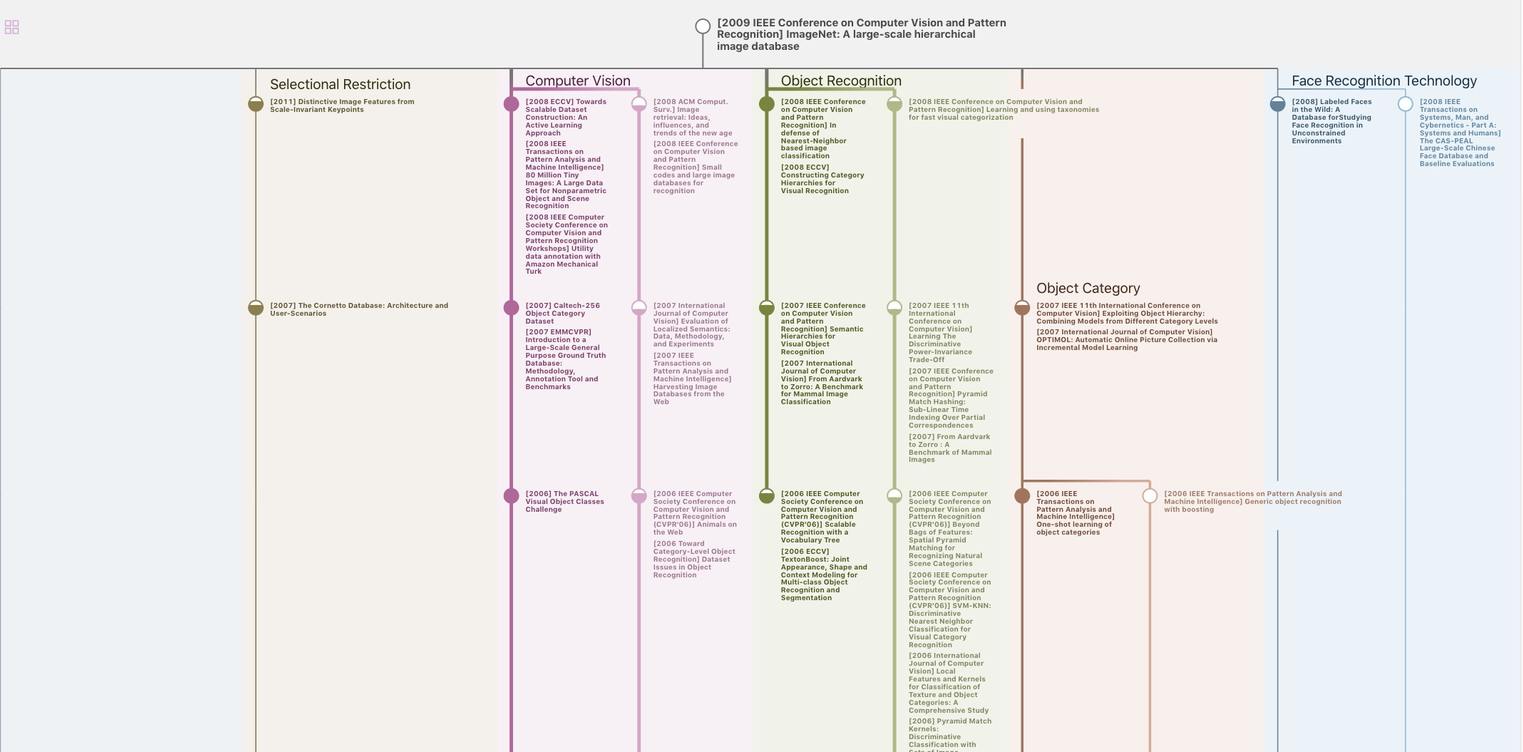
生成溯源树,研究论文发展脉络
Chat Paper
正在生成论文摘要