Identifying tracer and pollutant sources in drainage networks from point observations using an efficient convex unmixing scheme
crossref(2023)
摘要
<p>Water and sediment transport minerals, micro-plastics, heavy-metals, pathogens, DNA, RNA, and emerging contaminants through river networks. We would like to use point observations of these concentrations to determine where and how much of these are entering the network. However, downstream samples are mixtures of all the potential upstream sources. Separating out the contribution of an individual source requires "unmixing" the network's waters or sediments.</p> <p>Here, we describe a very efficient approach to perform such an unmixing, identifying the contribution from each nested sub-catchment in a drainage basin. First, we abstract the sub-catchments defined by our sampling sites into a directed acyclic graph. Each node (sub-catchment) in the graph is defined as having an upstream area, which we know, and a tracer source concentration, whose value we want to find. If we assume that when two rivers meet their tracers' fluxes are combined conservatively then downstream concentrations are the mixture of all upstream concentrations, weighted by upstream area.</p> <p>To solve for the source concentration of each sub-catchment we define a convex optimisation problem, minimising the relative difference in the predicted and observed tracer concentration at each sample site. Due to its convexity, this optimisation problem can be solved in less than a second for networks of a 100 nodes. Uncertainties can be estimated using a Monte-Carlo style approach. We have made an open-source, Python implementation of this algorithm available on GitHub. This implementation requires as input (1) a spreadsheet containing sample site locations and observed tracer concentrations and (2) a D8 flow-direction raster map. This is a powerful approach for locating and quantifying the sources of conservatively mixed tracers or pollutants in drainage networks.</p>
更多查看译文
AI 理解论文
溯源树
样例
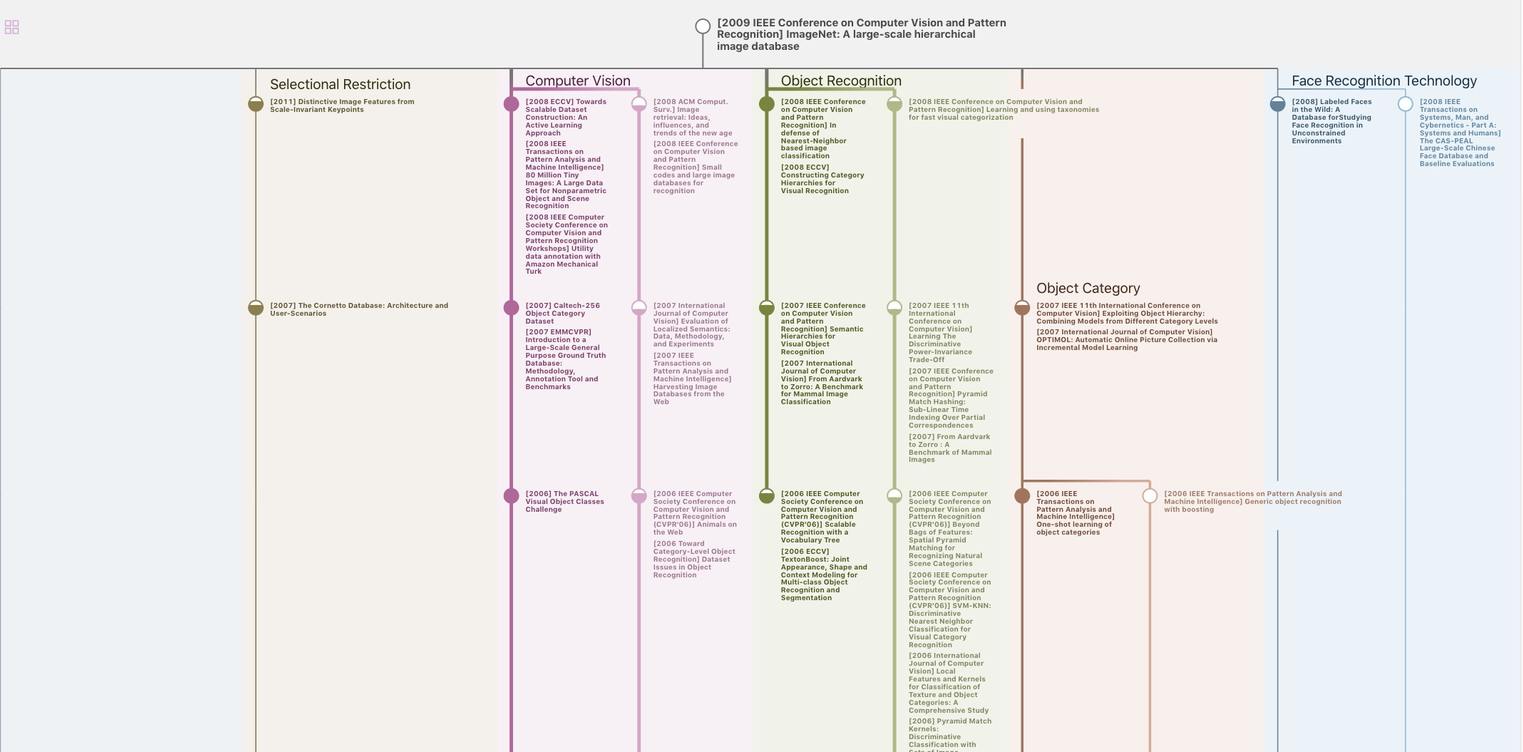
生成溯源树,研究论文发展脉络
Chat Paper
正在生成论文摘要