Towards flood warnings everywhere - data-driven rainfall-runoff modeling at global scale
crossref(2023)
摘要
<p>The goal of Google’s Flood Forecasting Initiative is to provide timely and actionable flood warnings to everyone, globally. Until recently, Google provided operational flood warnings only for specific partner countries, namely India, Bangladesh, Sri Lanka, Colombia, and Brazil. In 2021 our flood alerting system sent out around 115 million flood notifications, reaching over 23 million people in the affected local areas. In all of the regions mentioned above, our operational model relies on partnerships with local governments to provide real-time measurements of observed discharge or water level (Nevo et al. 2021). However, relying on real-time measurement data makes it harder to scale to new regions as a) this data does not exist everywhere, and b) even if it exists, it requires significant per-country time and resource investment.</p> <p>Building on research results from the last few years (e.g., Kratzert et al. 2019a, Kratzert et al. 2019b, Klotz et al. 2021), we built a global rainfall-runoff model that does not rely on real-time measurements in the operational context but only uses globally available forcing data and globally available catchment attributes. It can therefore be deployed everywhere, including in ungauged basins. Following Kratzert et al. (2019a), our rainfall-runoff model is based on the Long Short-Term Memory network (LSTM) and is trained on thousands of hydrologically diverse basins from all around the world. To forecast river discharge for any given river on Earth, the model uses time series data from various meteorological forcing products (IMERG, CPC, ERA5-Land, ECMWF’s IFS), as well as static catchment characteristics.</p> <p>This new model allows us to scale to new regions more quickly. As of January 2023, we now provide operational flood warnings to hundreds of sites across 48 countries worldwide, with hundreds of more sites being rolled out in the coming months. Besides our previous channels of communicating flood warnings (e.g. Google Search, Google Maps, Google Alerts, and direct communications with NGOs and governments), we also released FloodHub (g.co/floodhub), a new interactive portal that allows for easy access to all operational forecasts.</p> <p>Here, we present more information about the modeling methodology shifts, the challenges we faced and finally showcase the latest advancements made.</p> <p> </p> <p>References:</p> <p>Klotz, D., et al. (2022). Uncertainty estimation with deep learning for rainfall–runoff modeling. <em>Hydrology and Earth System Sciences</em>, <em>26</em>(6), 1673-1693.</p> <p>Kratzert, F., et al. (2019a). Towards learning universal, regional, and local hydrological behaviors via machine learning applied to large-sample datasets. <em>Hydrology and Earth System Sciences</em>, <em>23</em>(12), 5089-5110.</p> <p>Kratzert, F., et al. (2019b). Toward improved predictions in ungauged basins: Exploiting the power of machine learning. <em>Water Resources Research</em>, <em>55</em>(12), 11344-11354.</p> <p>Nevo, S., et al., (2021). Flood forecasting with machine learning models in an operational framework. <em>Hydrology and Earth System Sciences Discussions</em>, pp.1-31.</p>
更多查看译文
AI 理解论文
溯源树
样例
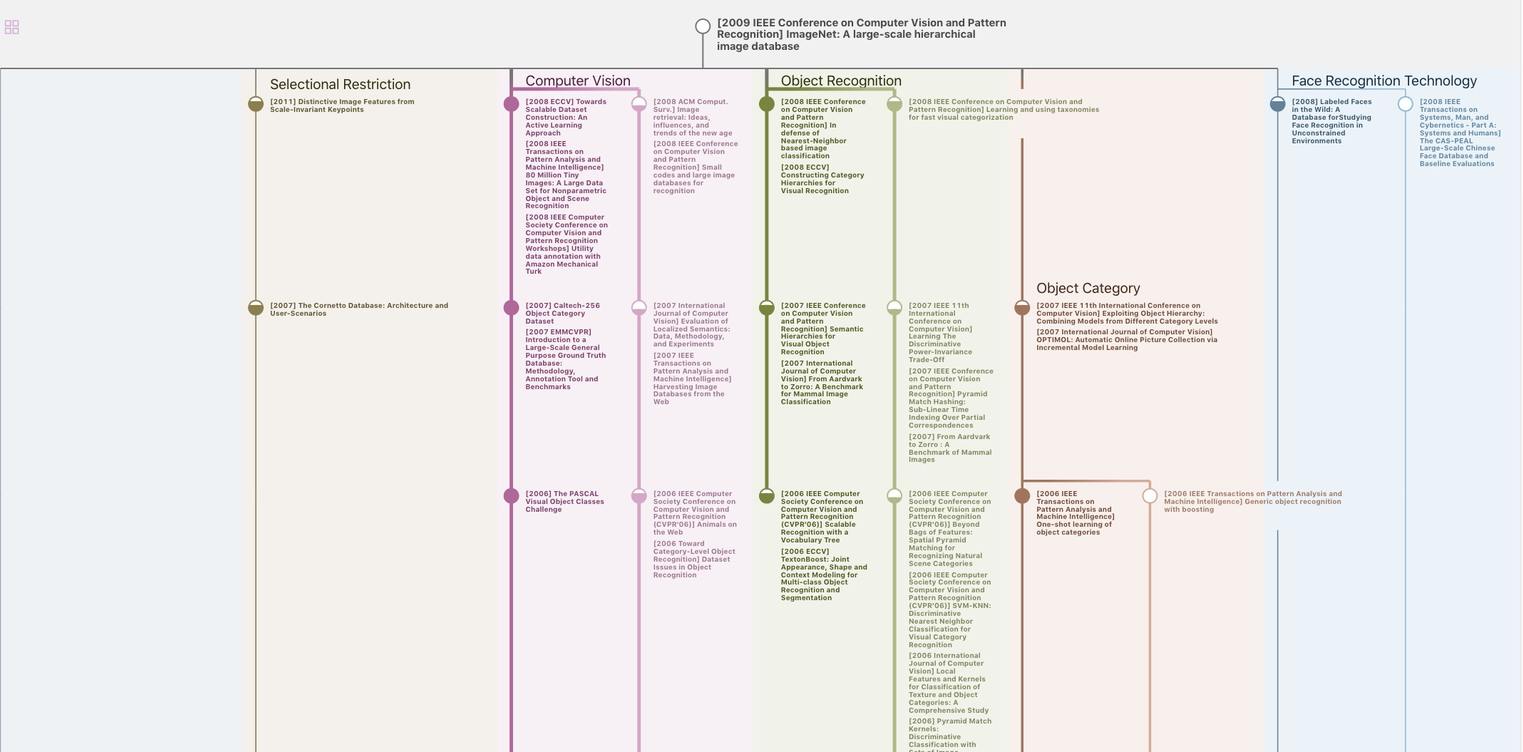
生成溯源树,研究论文发展脉络
Chat Paper
正在生成论文摘要