CLOOME: contrastive learning unlocks bioimaging databases for queries with chemical structures.
Nature communications(2023)
摘要
The field of bioimage analysis is currently impacted by a profound transformation, driven by the advancements in imaging technologies and artificial intelligence. The emergence of multi-modal AI systems could allow extracting and utilizing knowledge from bioimaging databases based on information from other data modalities. We leverage the multi-modal contrastive learning paradigm, which enables the embedding of both bioimages and chemical structures into a unified space by means of bioimage and molecular structure encoders. This common embedding space unlocks the possibility of querying bioimaging databases with chemical structures that induce different phenotypic effects. Concretely, in this work we show that a retrieval system based on multi-modal contrastive learning is capable of identifying the correct bioimage corresponding to a given chemical structure from a database of ~2000 candidate images with a top-1 accuracy >70 times higher than a random baseline. Additionally, the bioimage encoder demonstrates remarkable transferability to various further prediction tasks within the domain of drug discovery, such as activity prediction, molecule classification, and mechanism of action identification. Thus, our approach not only addresses the current limitations of bioimaging databases but also paves the way towards foundation models for microscopy images.
更多查看译文
关键词
databases,queries,chemical structures,learning
AI 理解论文
溯源树
样例
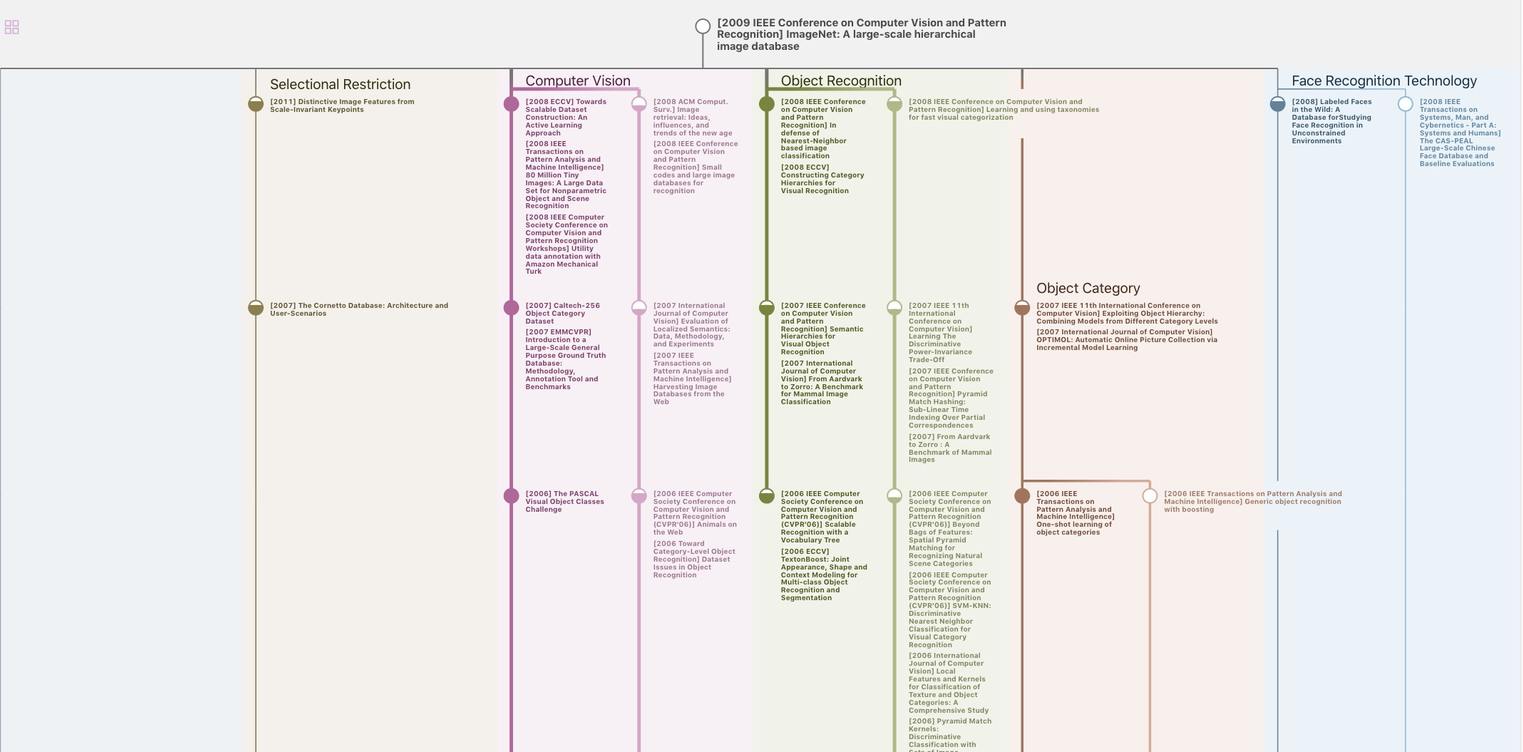
生成溯源树,研究论文发展脉络
Chat Paper
正在生成论文摘要