User Selection and Power Allocation Scheme With SINR-Based Deep Learning for Downlink NOMA
IEEE Transactions on Vehicular Technology(2023)
摘要
Innon-orthogonal multiple access (NOMA) systems, transmit power allocation plays a crucial role in maximizing the sum rate, while satisfying quality of service requirements of users. In prior study, an interior point method (IPM) has been presented as an iterative algorithm for power allocation, which can maximize the sum rate and minimize the outage rate. However, such iterative algorithms typically suffer from low computational efficiency and excessively high latency. To overcome these limitations, machine learning-based power allocation schemes have been widely studied. Along the same line, in this paper, focusing on unsupervised learning to improve the computational complexity for power allocation as well as user selection, we propose a deep neural network (DNN)-based joint user selection and power allocation technique for the cellular downlink multiple-input single-output(MISO) NOMA systems, where the transmit antenna selection and multi-cell cooperation are considered. Especially, an optimization problem for user selection and power allocation is formulated to maximize the sum rate under the constraint of minimum data-rate requirement. We propose a new loss function and training model for user selection and power allocation to solve the optimization problem, where the training and testing models are based on the signal-to-interference-plus-noise-ratio (SINR) information instead of channel state information, which can significantly reduce the input data size in DNN. Through simulations, we show that our proposed scheme provides comparable performances in terms of sum rate and outage rate to the optimal solutions, while requiring lower computational complexity compared to the optimal ones.
更多查看译文
关键词
Non-orthogonal multiple access,deep learning,user selection,power allocation,sum rate,inter-cell interference
AI 理解论文
溯源树
样例
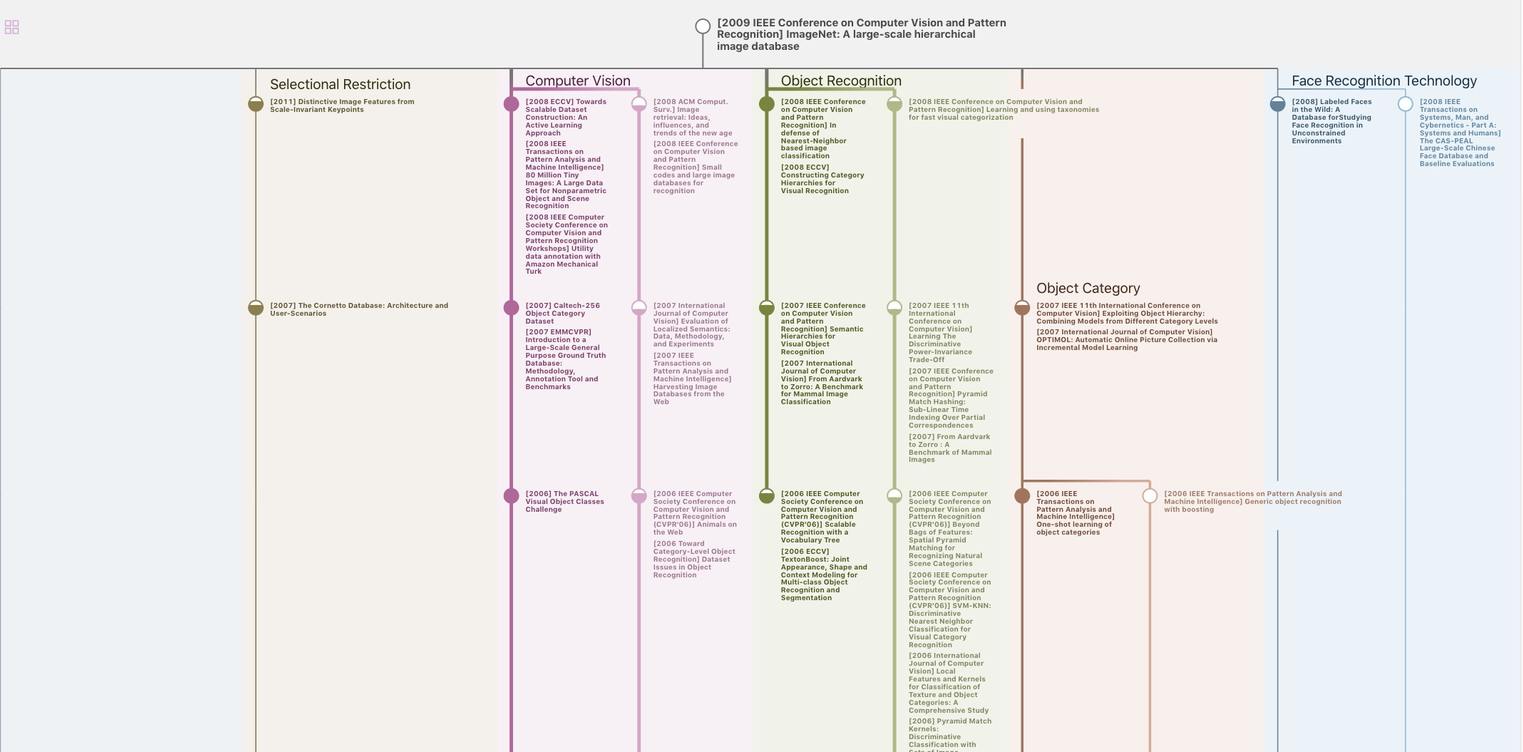
生成溯源树,研究论文发展脉络
Chat Paper
正在生成论文摘要