MAT: An improved Swin Transformer model for counting cultured fish
Research Square (Research Square)(2023)
摘要
Abstract The accurate and automatic counting of cultured fish becomes increasingly important in achieving efficient production management with the expansion of the scale of aquaculture. This study proposed a cultured fish counting model (MAT) based on Multi-column dilated convolution, Attention mechanism and swin Transformer to address the problem of low counting accuracy brought on by high stocking density and complicated environment. The MAT model contains two components: the feature extraction module and the attention module. The feature extraction module is a pyramid-like structured network based on the Swin Transformer block which can integrate feature information from various scales and use patch expanding to accomplish upsampling. The attention module consists of three parallel sets of dilated convolutional blocks in different size and a residual attention structure which are used to extract more critical information from images and generate high-quality density maps. The experimental results showed that the counting accuracy of MAT on the cultured fish dataset was 97.57%, and its mean absolute error (MAE) and the root mean square error (RMSE) were 2.09 and 2.38, respectively. Compared to MCNN and CSRnet, the counting accuracy of the MAT model was improved by 4.48% and 1.22%; MAE lowered by 63.5% and 32.8%; RMSE decreased by 63.5% and 32.8%. Additionally, the density maps generated by MAT were more refined. The proposed model was thus proved to have a high counting accuracy and produced high-quality density maps which can effectively estimate the spatial distribution, regional state and specific location of the fish population.
更多查看译文
关键词
improved swin transformer model,cultured fish
AI 理解论文
溯源树
样例
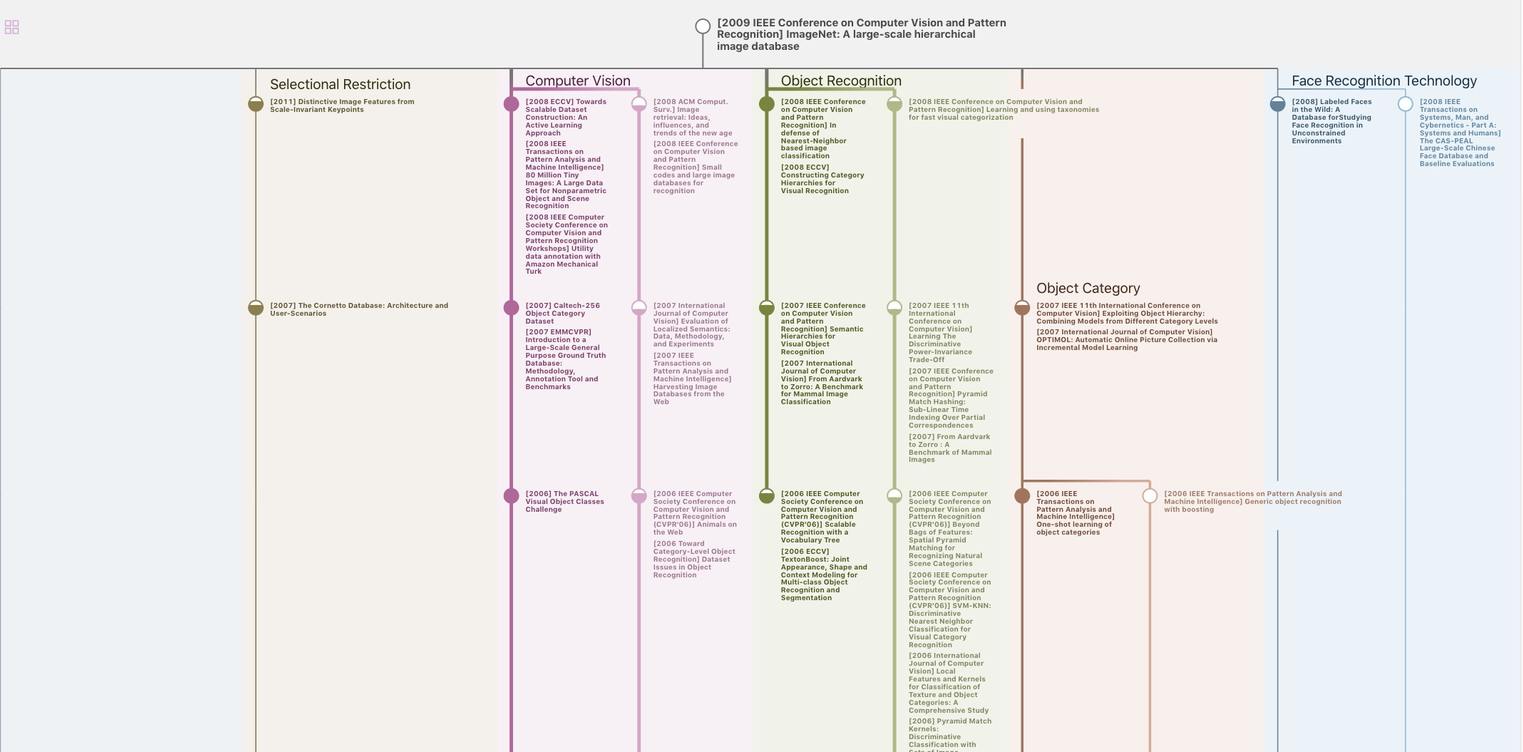
生成溯源树,研究论文发展脉络
Chat Paper
正在生成论文摘要