MTL-Leak: Privacy Risk Assessment in Multi-Task Learning.
IEEE Trans. Dependable Secur. Comput.(2024)
摘要
Multi-task learning (MTL) supports simultaneous training over multiple related tasks and learns the shared representation. While improving the generalization ability of training on a single task, MTL has higher privacy risk than traditional single-task learning because more sensitive information is extracted and learned in a correlated manner. Unfortunately, very few works have attempted to address the privacy risks posed by MTL. In this article, we first investigate such risk by designing model extraction attack (MEA) and membership inference attack (MIA) in MTL. Then we evaluate the privacy risks on six MTL model architectures and two popular MTL datasets, whose results show that both the number of tasks and the complexity of training data play an important role in the attack performance. Our investigation shows that MTL is more vulnerable than traditional single-task learning under both attacks.
更多查看译文
关键词
Multi-task learning,model extraction attacks,membership inference attacks,privacy threaten
AI 理解论文
溯源树
样例
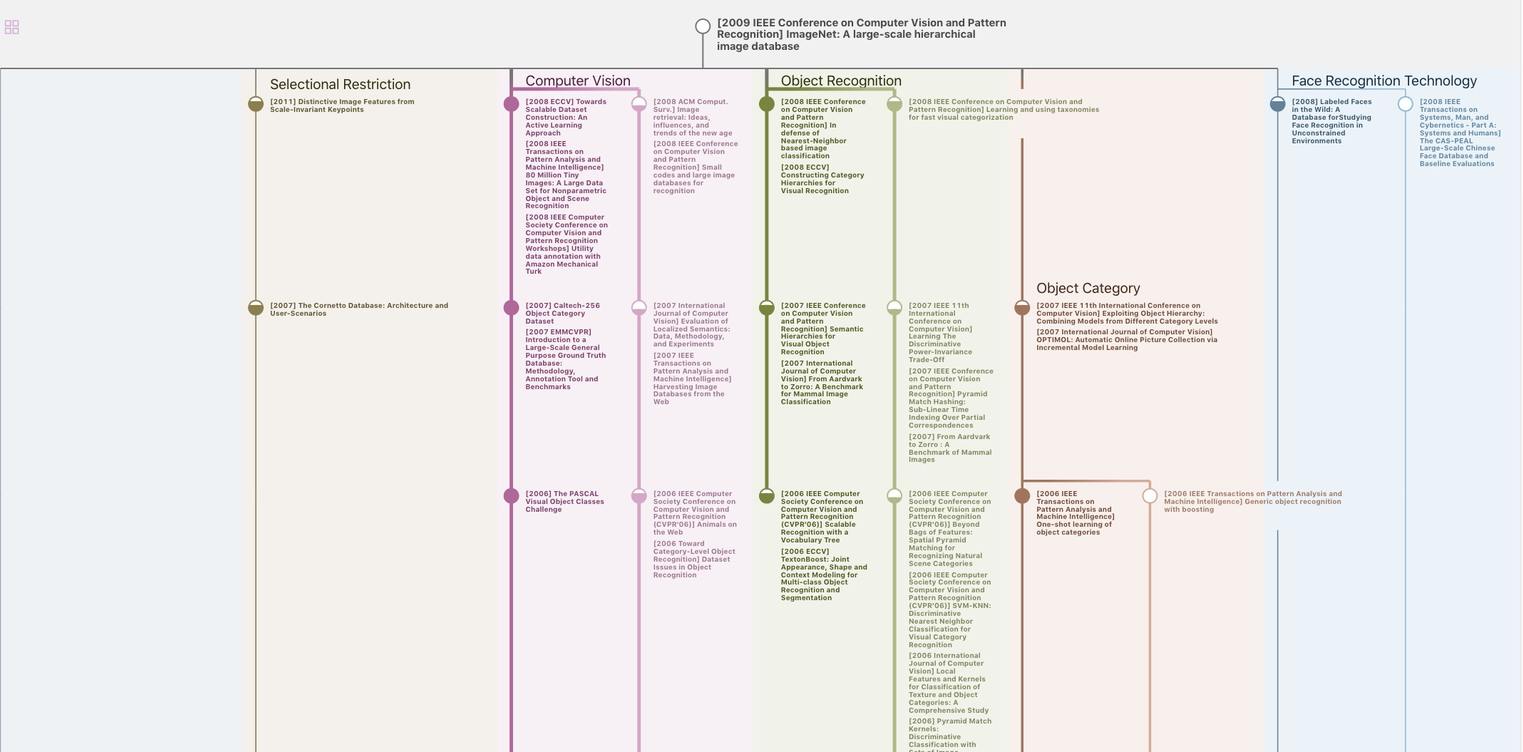
生成溯源树,研究论文发展脉络
Chat Paper
正在生成论文摘要