Explainable and Generalizable Blind Image Quality Assessment via Semantic Attribute Reasoning
IEEE TRANSACTIONS ON MULTIMEDIA(2023)
摘要
Blind image quality assessment (BIQA) that can directly evaluate image quality without perfect-quality reference has been a long-standing research topic. Although the existing BIQA models have achieved very encouraging performance, the lack of explainability and generalization ability limits their real-world applications to a great extent. People usually assess image quality according to semantic attributes, e.g., brightness, color, contrast, noise and sharpness. Furthermore, judgment on image quality is also impacted by the scene presented in the image. Therefore, the inherent relationship between semantic attributes and scenes is crucial for image quality assessment, which has rarely been explored yet. With this motivation, this paper presents a Semantic Attribute Reasoning based image QUality Evaluator (SARQUE). Specifically, we propose a two-stream network to predict semantic attributes and scene categories from distorted images. To investigate the inherent relationship between the semantic attributes and scene category, a semantic reasoning module is further proposed based on the graph convolution network (GCN), producing the final quality score. Extensive experiments conducted on five in-the-wild image quality databases demonstrate the superiority of the proposed SARQUE model over the state-of-the-arts. Furthermore, the proposed model features better explainability and generalization ability due to the use of semantic attributes.
更多查看译文
关键词
Blind image quality assessment,explainability,generalization ability,semantic attribute,graph convolution network
AI 理解论文
溯源树
样例
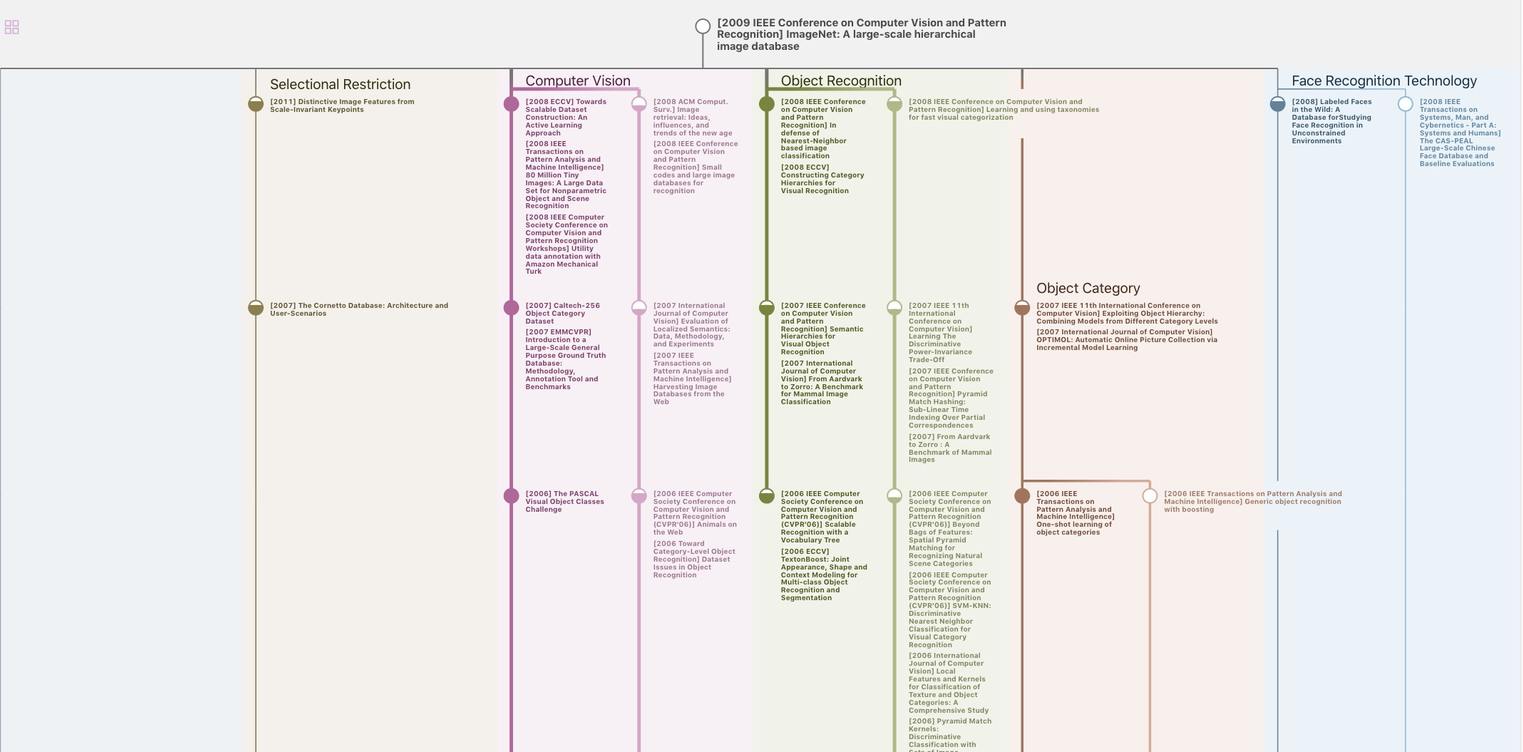
生成溯源树,研究论文发展脉络
Chat Paper
正在生成论文摘要