Dynamic learning rates for continual unsupervised learning
Integrated Computer-Aided Engineering(2023)
摘要
The dilemma between stability and plasticity is crucial in machine learning, especially when non-stationary input distributions are considered. This issue can be addressed by continual learning in order to alleviate catastrophic forgetting. This strategy has been previously proposed for supervised and reinforcement learning models. However, little attention has been devoted to unsupervised learning. This work presents a dynamic learning rate framework for unsupervised neural networks that can handle non-stationary distributions. In order for the model to adapt to the input as it changes its characteristics, a varying learning rate that does not merely depend on the training step but on the reconstruction error has been proposed. In the experiments, different configurations for classical competitive neural networks, self-organizing maps and growing neural gas with either per-neuron or per-network dynamic learning rate have been tested. Experimental results on document clustering tasks demonstrate the suitability of the proposal for real-world problems.
更多查看译文
关键词
dynamic learning rates
AI 理解论文
溯源树
样例
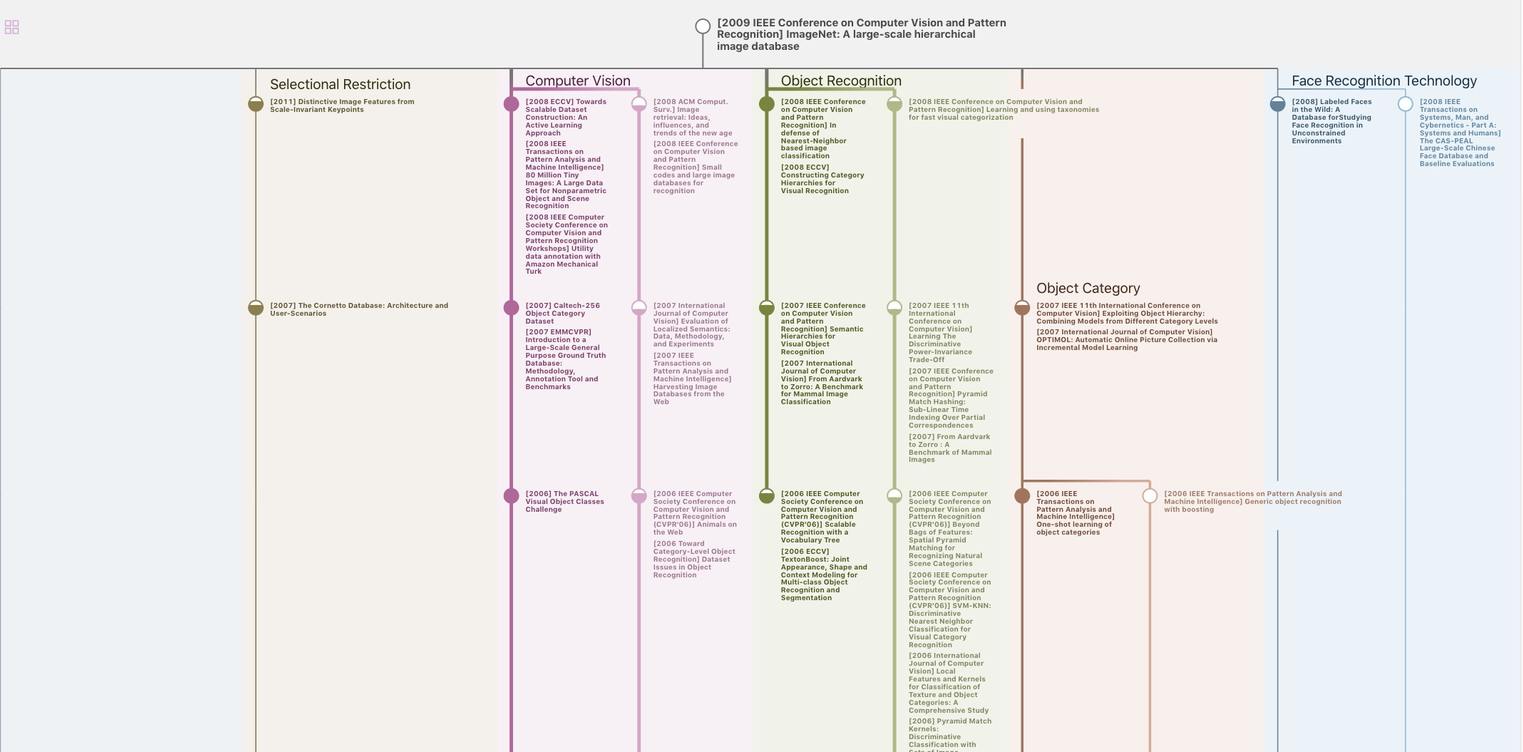
生成溯源树,研究论文发展脉络
Chat Paper
正在生成论文摘要