A Low-cost and Configurable Hardware Architecture of Sparse 1-D CNN for ECG Classification
2022 IEEE 16th International Conference on Solid-State & Integrated Circuit Technology (ICSICT)(2022)
摘要
Pruning techniques have been widely used to compress large scale CNN models, which is friendly to the resource limited electrocardiogram (ECG) classification application. However, the random distribution of non-zero weights make the parallel calculation in hardware less efficient. In this work, a low-cost hardware architecture especially for sparse 1-D CNN is presented. The configurable PE array which contains three kinds of primitive PE structures is proposed to address the workload imbalance issue by forming multiple data path combination. Implemented on Xilinx Zynq ZC706 FPGA platform, this work achieves an accuracy of 99.17% on five types of ECG beats real-time classification with a 60% sparsity 1-D CNN, consuming only 1995 LUT, 3011 FF and 12 DSP.
更多查看译文
关键词
sparse,configurable hardware architecture,low-cost
AI 理解论文
溯源树
样例
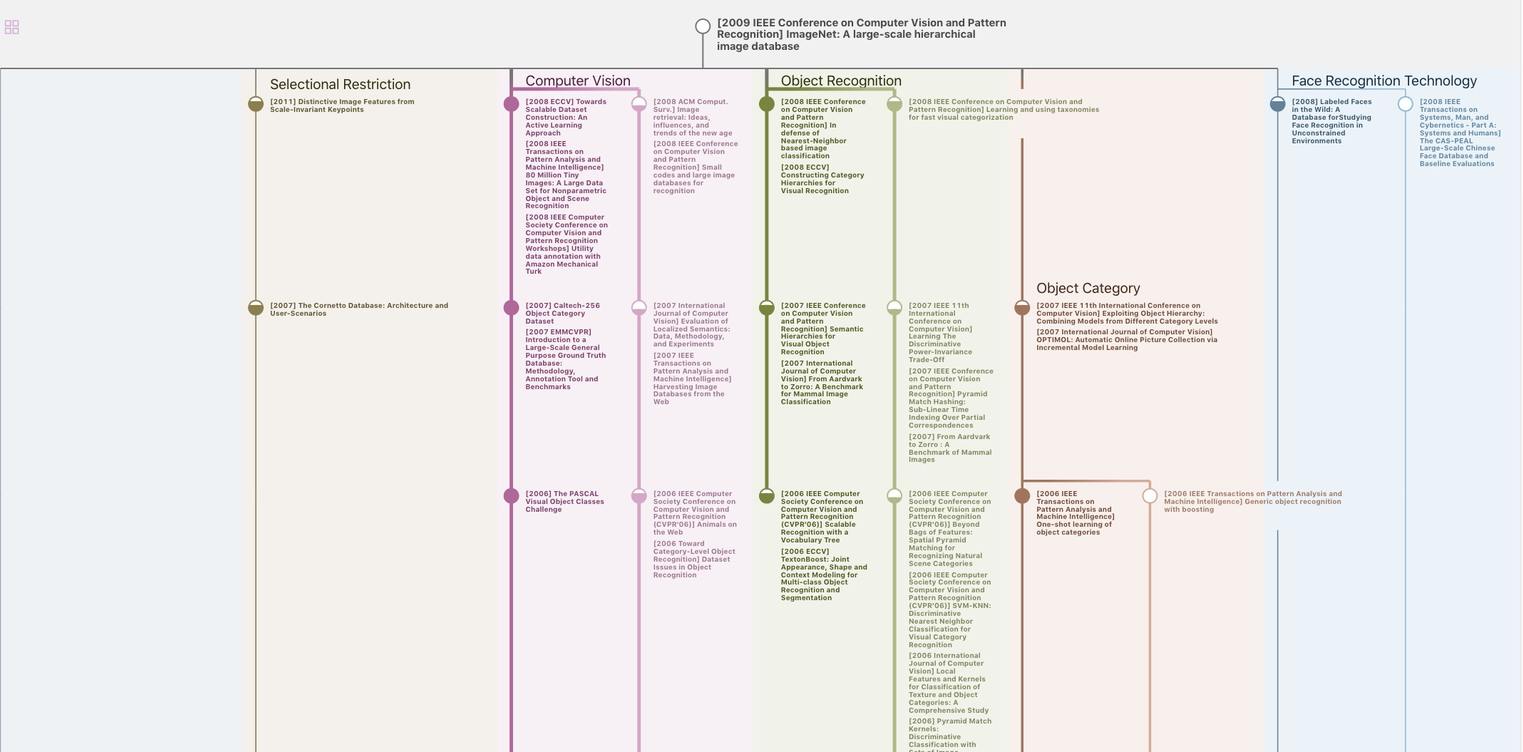
生成溯源树,研究论文发展脉络
Chat Paper
正在生成论文摘要