Interactive nonlocal joint learning network for red, green, blue plus depth salient object detection
Journal of Electronic Imaging(2022)
摘要
Research into red, green, blue plus depth salient object detection (SOD) has identified the challenging problem of how to exploit raw depth features and fuse cross-modal (CM) information. To solve this problem, we propose an interactive nonlocal joint learning (INL-JL) network for quality RGB-D SOD. INL-JL benefits from three key components. First, we carry out joint learning to extract common features from RGB and depth images. Second, we adopt simple yet effective CM fusion blocks in lower levels while leveraging the proposed INL blocks in higher levels, aiming to purify the depth features and to make CM fusion more efficient. Third, we utilize a dense multiscale transfer strategy to infer saliency maps. INL-JL advances the state-of-the-art methods on five public datasets, demonstrating its power to promote the quality of RGB-D SOD. (c) 2022 SPIE and IS&T
更多查看译文
关键词
salient object detection, red, green, blue plus depth data, interactive nonlocal joint learning, cross-modal fusion
AI 理解论文
溯源树
样例
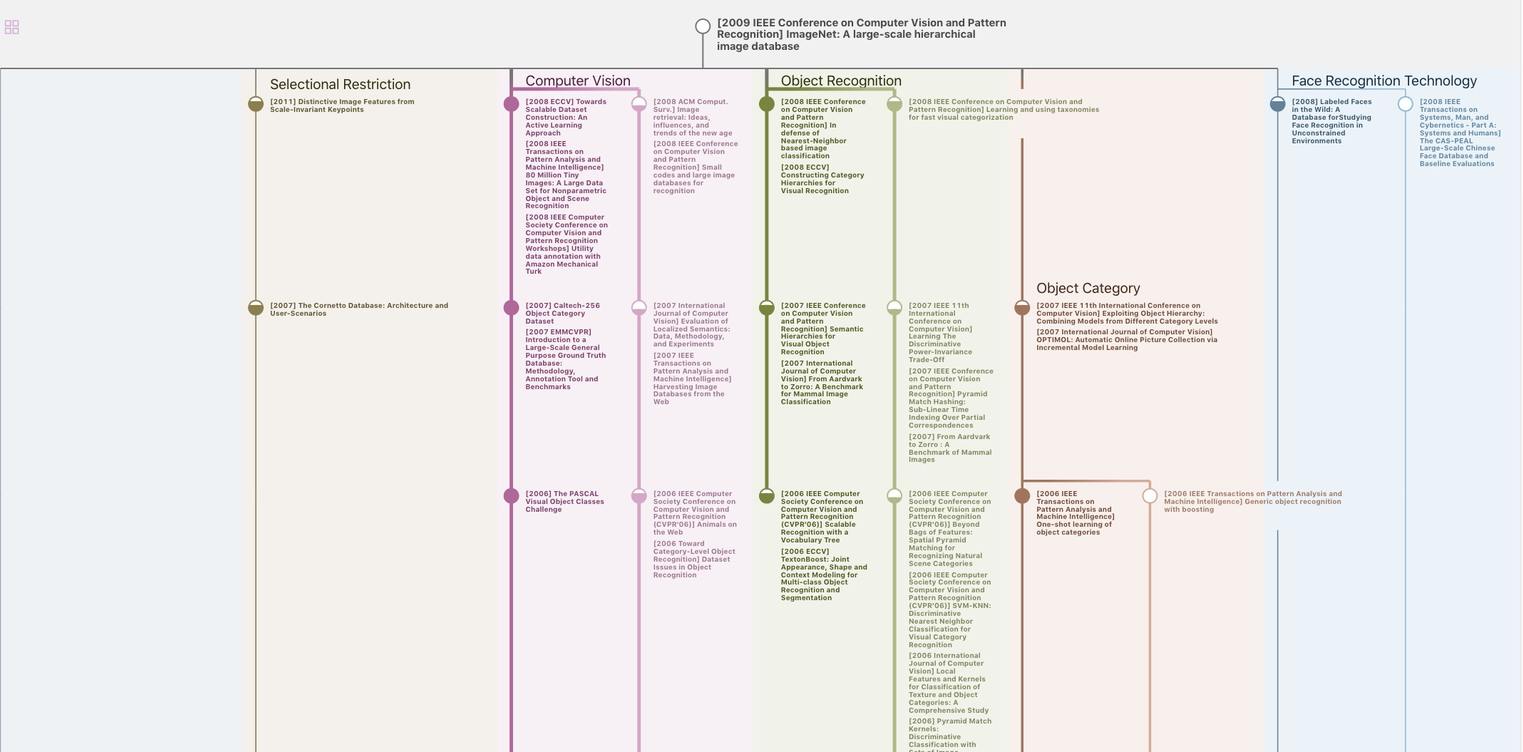
生成溯源树,研究论文发展脉络
Chat Paper
正在生成论文摘要