ADCL: Toward an Adaptive Network Intrusion Detection System Using Collaborative Learning in IoT Networks
IEEE Internet of Things Journal(2023)
摘要
With the widespread of cyber attacks, network intrusion detection system (NIDS) is becoming an important and essential tool to protect Internet of Things (IoT) environments. However, it is well known that the NIDS performance depends heavily on the effectiveness of the detection model, which can be influenced significantly by the learning mechanism and the available training data. Many existing studies try to mitigate the above challenges, but few of them consider the adaptability and the cost of deploying an NIDS, the integrity of the learning process, the capacity of model based on concrete traffic samples at the same time. To fill this gap and improve the detection performance, we propose a collaborative learning-based detection framework called ADCL, which can mitigate the limitations on the knowledge of a single model by leveraging multiple models trained in similar environments and detecting intrusions in a collaborative manner. Our evaluation results indicate that ADCL can provide better performance compared with a single model on detecting various attacks in IoT networks. Specifically, ADCL improves F-score by up to 80% for adaptability, 42% in mitigating the reliance on learning integrity, 85% for model capacity. Furthermore, the detection results of ADCL guide those single models to update and increase the F-score by 15%.
更多查看译文
关键词
Collaborative learning,Internet of Things (IoT),intrusion detection,multiple model,system adaptability
AI 理解论文
溯源树
样例
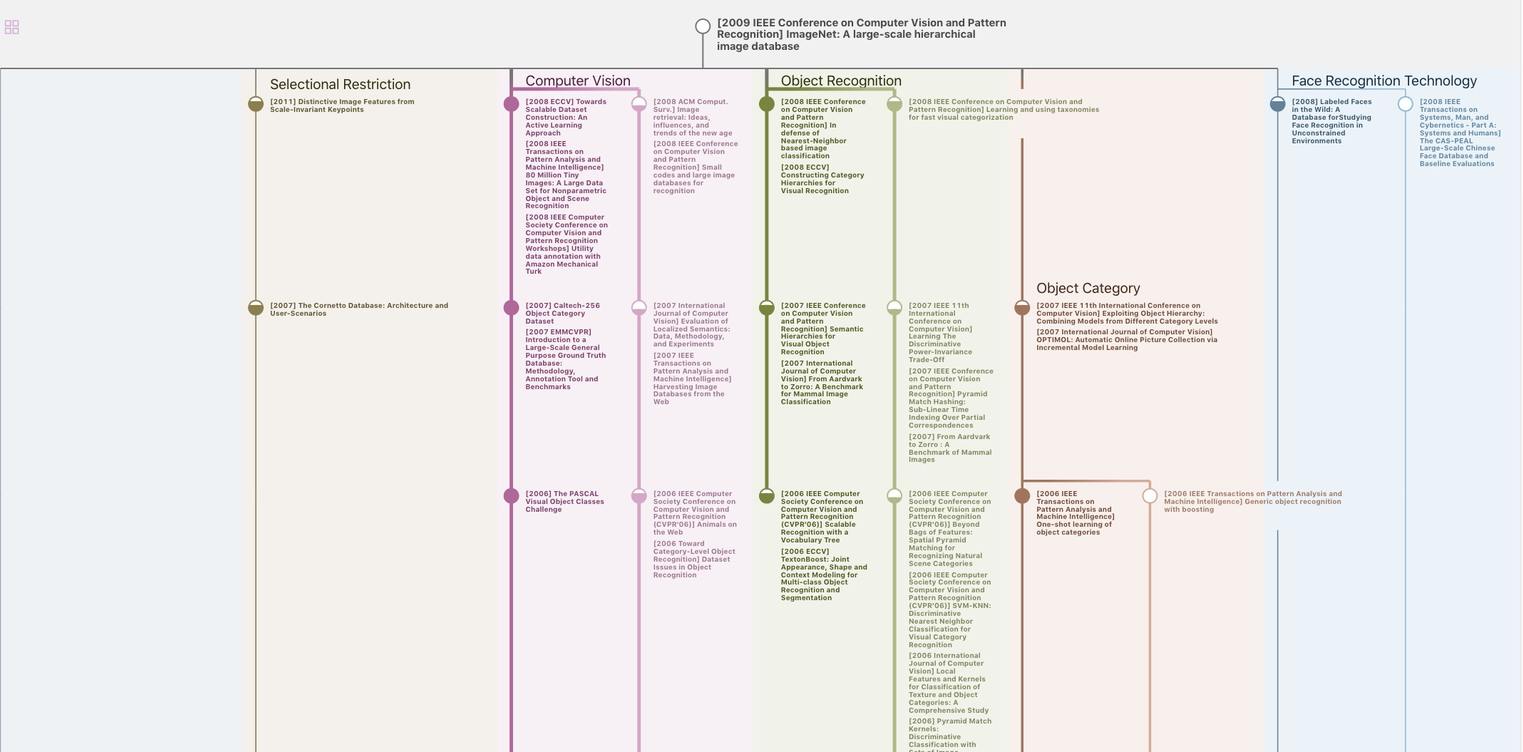
生成溯源树,研究论文发展脉络
Chat Paper
正在生成论文摘要