Data-driven discovery of organic electronic materials enabled by hybrid top-down/bottom-up design
crossref(2022)
摘要
The high-throughput molecular exploration and screening of organic electronic materials often starts with either a 'top-down' mining of existing repositories, or the 'bottom-up' assembly of fragments based on predetermined rules and known synthetic templates. In both instances, the datasets used are often produced on a case-by-case basis, and require the high-quality computation of electronic properties and extensive user input: curation in the top-down approach, and the construction of a fragment library and introduction of rules for linking them in the bottom-up approach. Both approaches are time-consuming and require significant computational resources. Here, we generate a top-down set of 117K synthesized molecules containing their optimized structures, associated electronic and topological properties and chemical composition, and use these structures as a vast library of molecular building blocks for bottom-up fragment-based materials design. A tool is developed to automate the coupling of these building block units based on their available C(sp2/sp)-H bonds, thus providing a fundamental link between the two philosophies of dataset construction. Statistical models are trained on this dataset and a subset of the resulting hybrid top-down/bottom-up compounds, which enable on-the-fly prediction of key ground state (frontier molecular orbital gaps) and excited state (S1 and T1 energies) properties from molecular geometries with high accuracy across all known p-block organic compound space. With access to ab initio-quality optical properties in hand, it is possible to apply this bottom-up pipeline using existing compounds as molecular building blocks to any materials design campaign. To illustrate this, we construct and screen over a million molecular candidates for efficient intramolecular singlet fission, the leading candidates of which provide insight into the structural features that may promote this multiexciton-generating process.
更多查看译文
AI 理解论文
溯源树
样例
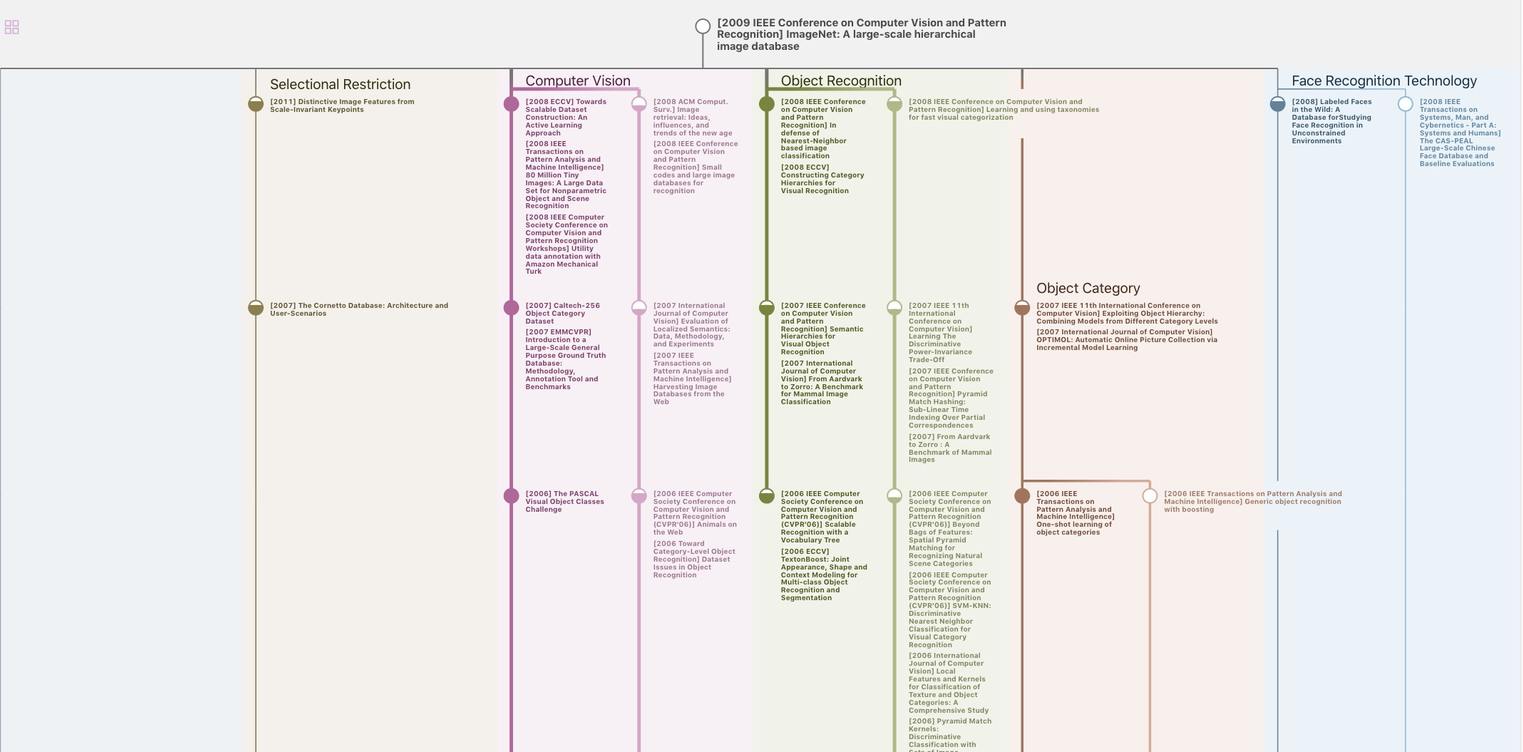
生成溯源树,研究论文发展脉络
Chat Paper
正在生成论文摘要