Semi-Supervised Event Extraction Incorporated With Topic Event Frame
Journal of Database Management(2023)
摘要
Supervised Meta-event extraction suffers from two limitations: (1) The extracted meta-events only contain local semantic information and do not present the core content of the text; (2) model performance is easily degraded because of labeled samples with insufficient number and poor quality. To overcome these limitations, this study presents an approach called frame-incorporated semi-supervised topic event extraction (FISTEE), which aims to extract topic events containing global semantic information. Inspired by the frame-based knowledge representation, a topic event frame is developed to integrate multiple meta-events into a topic event. Combined with the tri-training algorithm, a strategy for selecting unlabeled samples is designed to expand the training sets, and labeling models based on conditional random field (CRF) are constructed to label meta-events. The experimental results show that the event extraction performance of FISTEE is better than supervised learning-based approaches. Furthermore, the extracted topic events can present the core content of the text.
更多查看译文
关键词
CRF, Meta-event Extraction, Semantic Information, Semi-supervised Learning, Sequence Labeling, Topic Event Extraction, Topic Event Frame, Tri-training
AI 理解论文
溯源树
样例
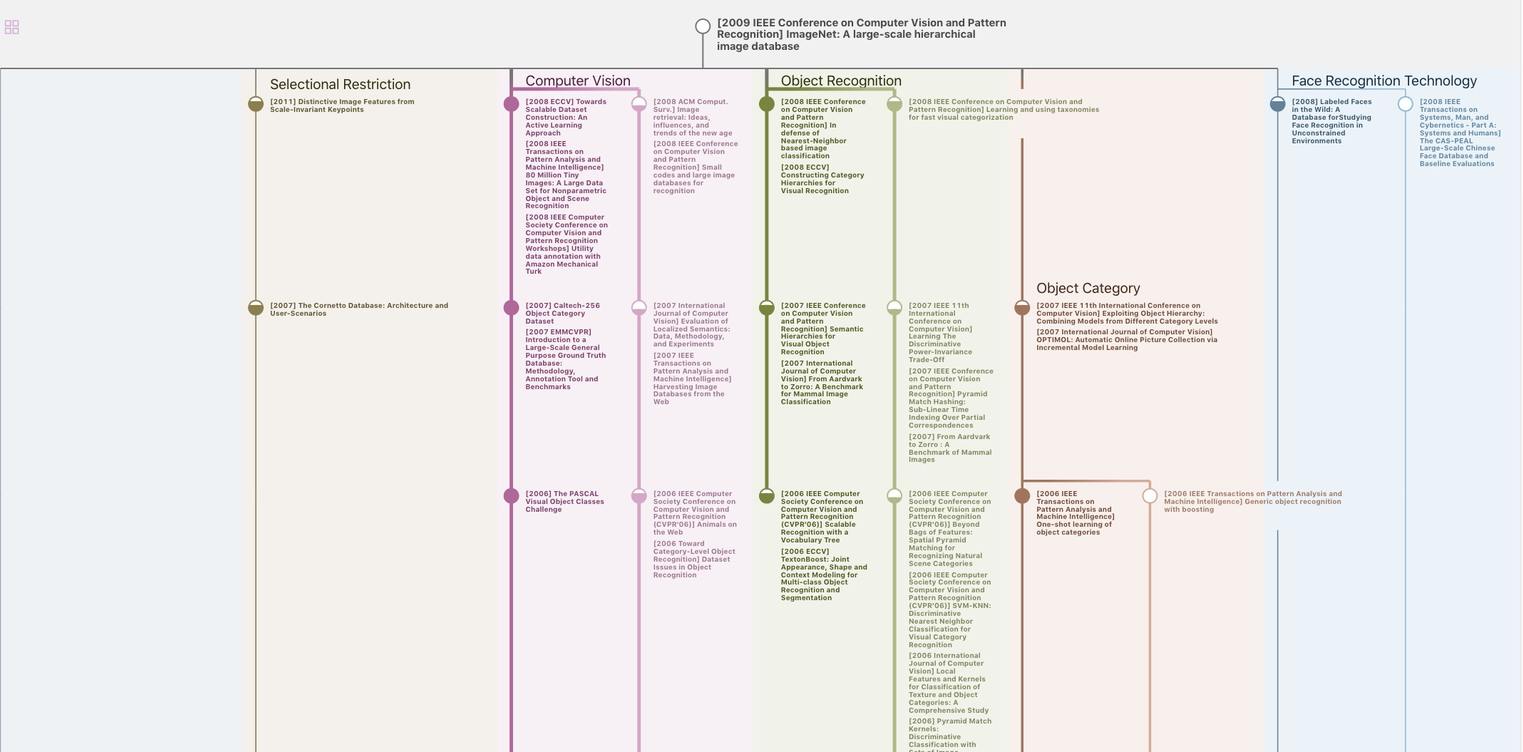
生成溯源树,研究论文发展脉络
Chat Paper
正在生成论文摘要