An Explainable AI Model for ICU Admission Prediction of COVID-19 Patients
INTERNATIONAL JOURNAL ON ARTIFICIAL INTELLIGENCE TOOLS(2023)
摘要
COVID-19 has overwhelmed hospitals all around the world, because of the very high number of patients requiring intubation. Predicting and anticipating which patients will be intubated can help hospitals better allocate their resources. For this, we created an explainable AI model that receives some medical data for each patient and returns a prediction on whether they will be intubated later on, while also explaining the argumentation process that led to that conclusion. This model can help medical professionals better manage the ICU beds and the patients between hospitals and warn them on the symptoms and signs to look for in patients worsening. To obtain the model, we first trained various ML algorithms on a dataset of COVID ICU admissions. Then, using the most accurate of the models, we obtained the sum of the most important rules, using R's inTrees framework. With the rules obtained, we produced a program in Gorgias written in Prolog. We added some more rules and changed the ordering to come up with a program that requires some patient vitals and blood results as inputs and then returns the prediction along with its reasoning.
更多查看译文
关键词
Explainable AI,machine learning,COVID-19,Gorgias
AI 理解论文
溯源树
样例
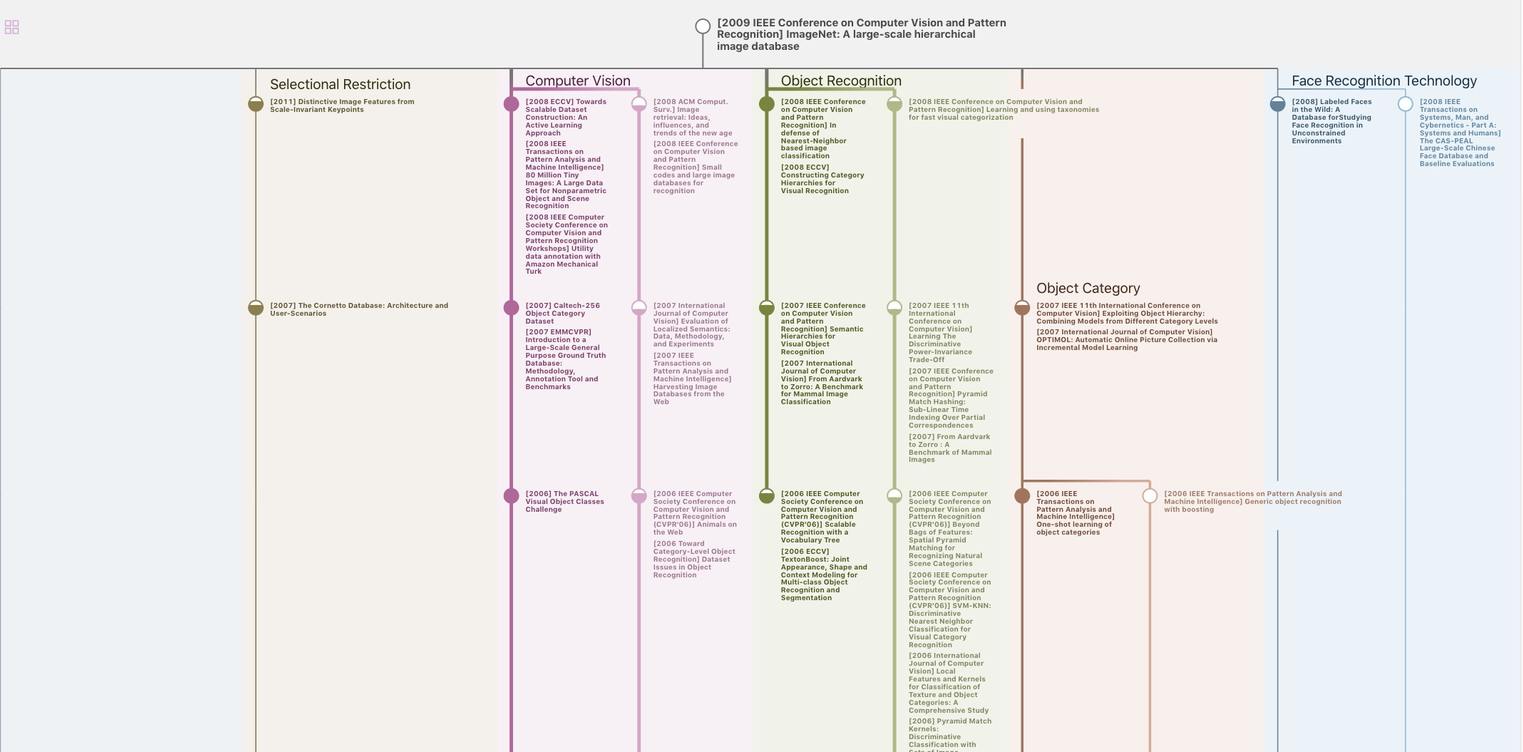
生成溯源树,研究论文发展脉络
Chat Paper
正在生成论文摘要