Chinese Clinical Named Entity Recognition in Electronic Medical Records: Using Multimodal Features With the Help of RoBERTa-wwm and CNN (Preprint)
crossref(2022)
摘要
BACKGROUND Clinical electronic medical records (EMRs) contain important medical information expressing patients' anatomy, symptoms, examinations, diagnoses, and medications. Large-scale mining of rich medical information from massive amounts of electronic medical record data will have significant reference value for medical research. With the complexity of Chinese grammar and the blurred boundaries of Chinese words, Chinese Clinical Named Entity Recognition (CNER) remains a significant challenge. The follow-up tasks, like medical entity structuring, medical entity standardization, medical entity relationship extraction, and medical knowledge graph construction, largely depend on the medical named entity recognition effects. A promising CNER result would provide reliable support for building domain knowledge graphs, knowledge bases, and knowledge retrieval systems. Further, it would provide research ideas for scientists, medical decision-making references for doctors, and even guide patients on disease and health management. Therefore, it is essential to provide great CNER results. OBJECTIVE This paper aims to propose a Chinese CNER method to learn semantic-enriched representations for comprehensively enhancing machines to understand deep semantic information of EMRs by using multimodal features, which makes medical information more readable and more understandable. METHODS Firstly, we used RoBERTa-wwm with dynamic fusion and Chinese character features, including Five-stroke code, Zheng code, Phonological code, and Stroke code, extracted by one-dimensional convolutional neural networks (CNN) to obtain Chinese character semantic features. Subsequently, we converted Chinese characters into square images to obtain Chinese character image features by using two-dimensional CNN. Finally, we input multimodal features into Bidirectional Long Short-Term Memory with Conditional Random Fields (BiLSTM-CRF) to achieve Chinese CNER. Our model's effectiveness is compared with the baseline and existing research models, and the features involved in the model are ablated and analyzed to verify the model's effectiveness. RESULTS We collected 1,397 CCKS-2019 EMRs containing 23,655 entities in six categories, and 2,007 Self-annotated EMRs containing 118,643 entities in seven categories. The experiments showed that our model outperformed the comparison experiments, with F1 values of 89.28% and 84.61% on the Yidu-S4K dataset and the Self-annotated dataset, respectively. The results of the ablation analysis demonstrated that each feature and method we used could improve the entity recognition ability. CONCLUSIONS Our proposed CNER method would mine the richer deep semantic information in EMRs by multimodal embedding using RoBERTa-wwm and CNN, enhancing the semantic recognition of characters at different granularity levels, and improving the generalization capability of the method by achieving information complementarity among different modalities, thus making machine semantically understand EMRs and improving the CNER task accuracy.
更多查看译文
AI 理解论文
溯源树
样例
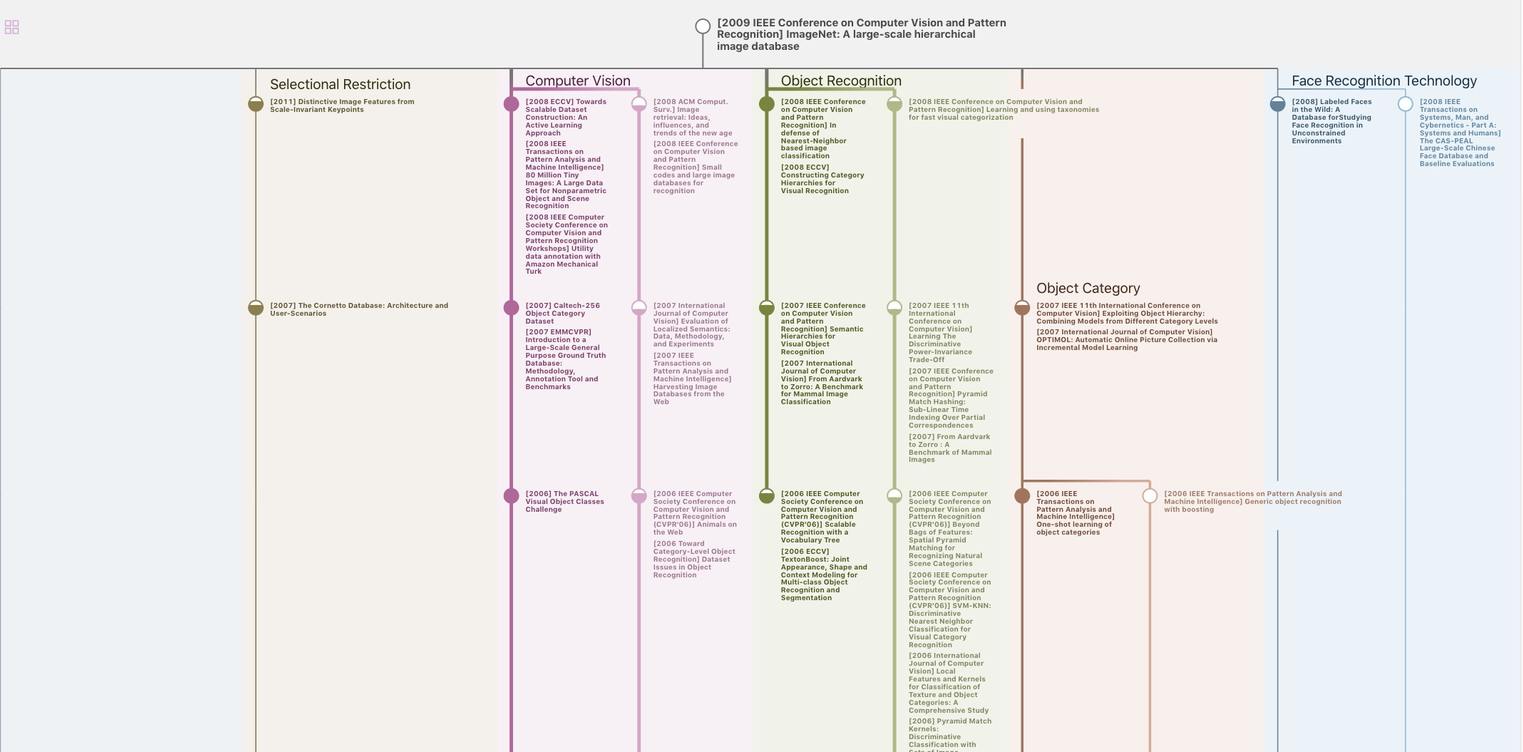
生成溯源树,研究论文发展脉络
Chat Paper
正在生成论文摘要