Hierarchical-Based Semantic Segmentation of 3D Point Cloud Using Deep Learning
EAI-Springer Innovations in Communication and Computing(2023)
摘要
AbstractIn this chapter, we present a new hierarchical deep learning-based approach for semantic segmentation of 3D point cloud. Our method involves nearest neighbor search for local feature extraction followed by an auxiliary pretrained network for classification. The proposed method constructs an octree representation of given point cloud and then performs a box search for finding neighborhood points, which are used in local feature extraction. The problem of segmentation is redefined to a per point classification task, and segmentation labels of every point is evaluated independently. This architecture can be fed with any number of points in input point cloud as opposed to state-of-the-art existing architectures, which demands fixed size input. Usefulness of the proposed approach is substantiated by supportive qualitative and quantitative results on shapenet dataset. Our method can produce effective segmentation even for shapes different from the ones used while training.Keywords3D point cloud processingSemantic segmentationOctreeDeep learningNearest neighborhood search
更多查看译文
AI 理解论文
溯源树
样例
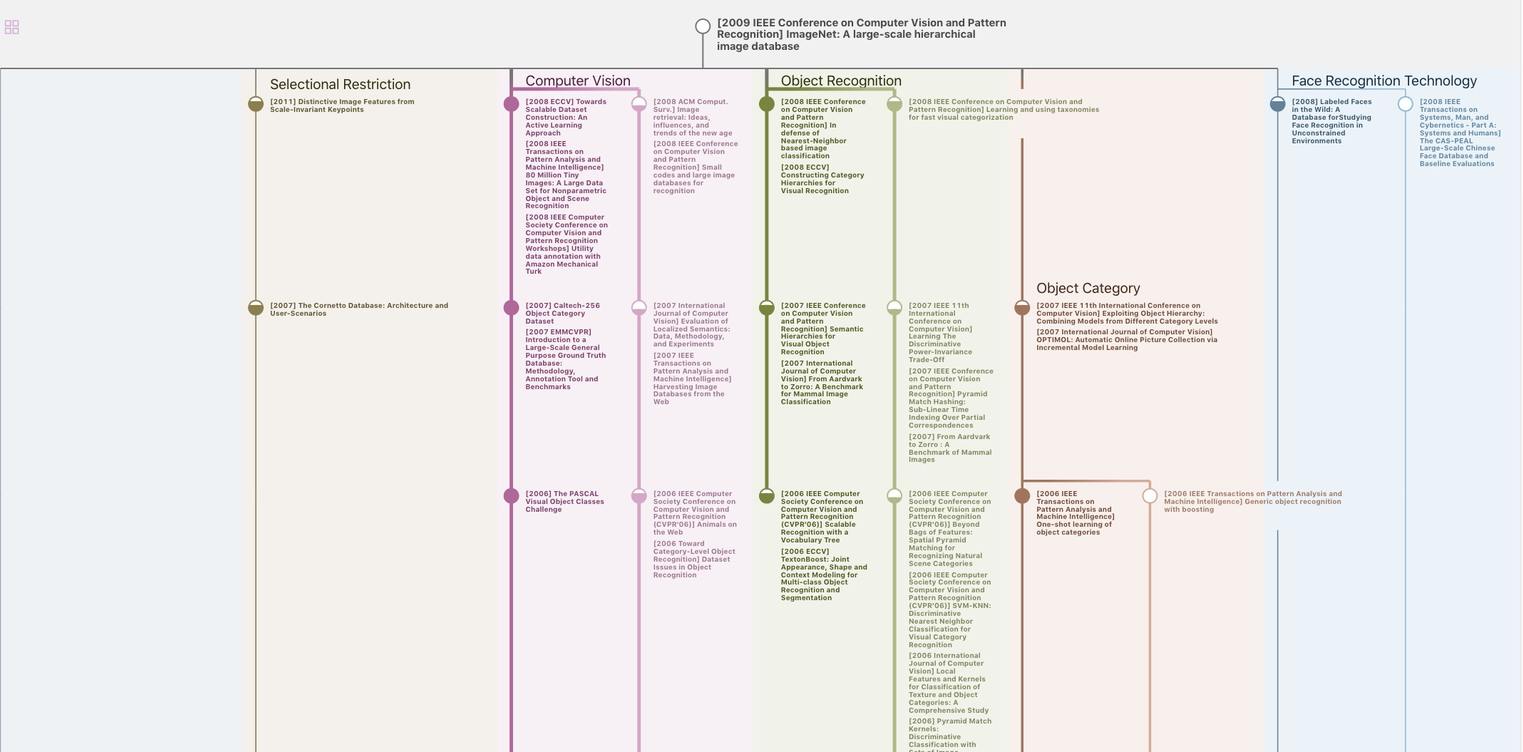
生成溯源树,研究论文发展脉络
Chat Paper
正在生成论文摘要