Dual-Branch TransV-Net for 3-D Echocardiography Segmentation
IEEE TRANSACTIONS ON INDUSTRIAL INFORMATICS(2023)
摘要
Segmentation of left and right ventricles from 3D echocardiographic images is premised and key for quantitative analysis of cardiac function, which is important for pediatric cardiac diagnosis. Compared with 2D echocardiography, 3D echocardiography can fully represent ventricular structure without geometric inference. However, it usually takes experts several hours to obtain a 3D segmentation mask of the left and right ventricles. Therefore, a fast and automatic segmentation method is highly desired. Unfortunately, 3D echocardiography suffers from low contrast, unclear left and right ventricles borders, and blind zone. Existing segmentation methods usually have poor performance at ventricular boundaries. To deal with these problems, we propose a novel dual-branch TransV-Net (DBTV). DBTV comprises two parallel, interleaved, and relatively independent V-shaped encoder-decoder branches. The main branch acts on the original data to extract image features, and the auxiliary branch acts on edge maps to extract the additional edge features. To suppress noise and enhance the edge information, extra concatenations are added to bridge the features from the main and auxiliary branches. To reduce object missing caused by blind zone, a 3D transformer-based module is proposed in the bottom layer of the dual-branch structure to extract the global contexts. We do experiments on a self-collected dataset with 120 3D echocardiographic images from 60 cardiac sequences, and the dice score of 0.913 and 0.880 are obtained in the left and right ventricle segments, respectively. Each inference takes about two-thirds of a second for a single 3D frame.
更多查看译文
关键词
3-D echocardiography (3DE),deep-learning,dual-branch network,left and right ventricle segmentation,medical image segmentation,self-attention
AI 理解论文
溯源树
样例
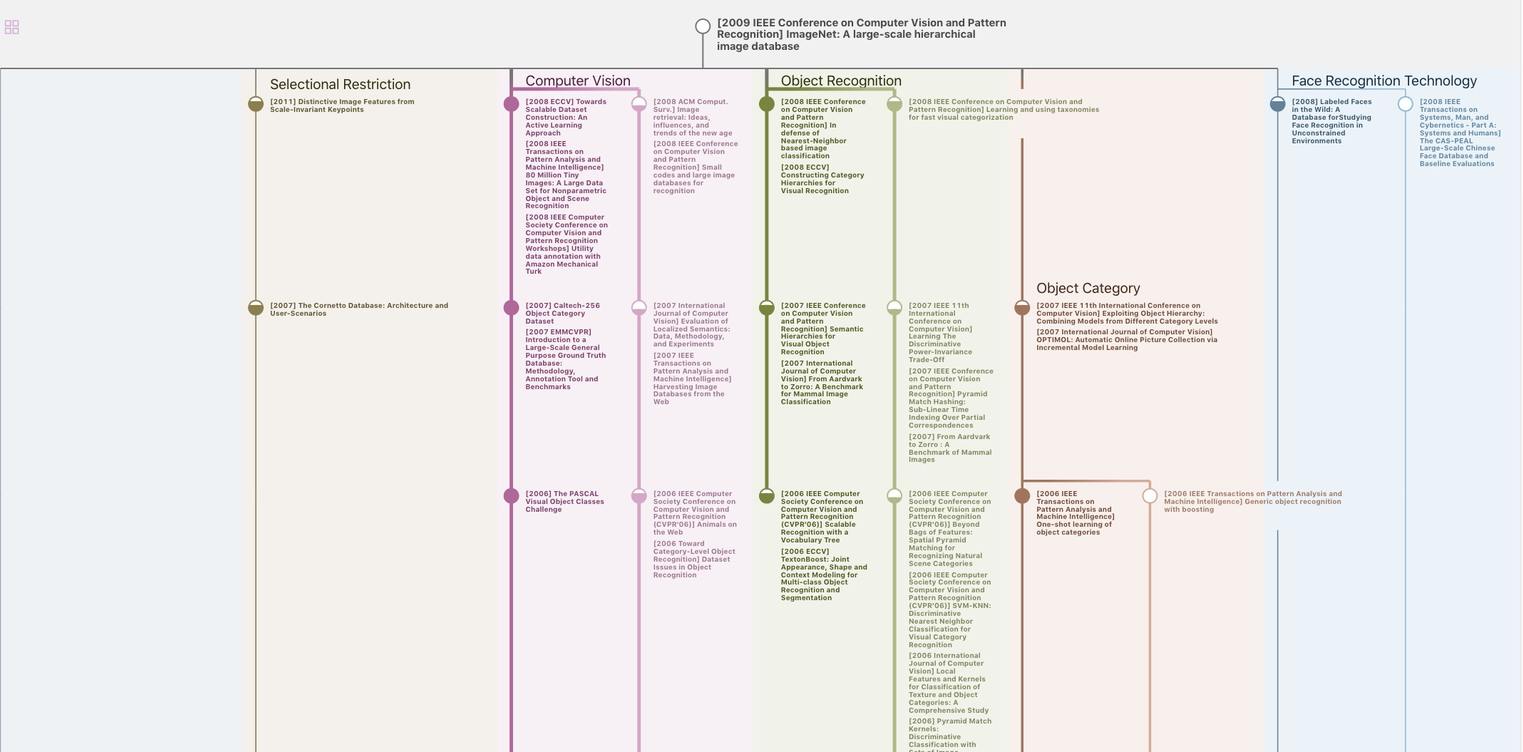
生成溯源树,研究论文发展脉络
Chat Paper
正在生成论文摘要