RINet: Relative Importance-Aware Network for Fixation Prediction
IEEE TRANSACTIONS ON MULTIMEDIA(2023)
摘要
Fixation prediction aims to simulate human visual selection mechanism and estimate the visual saliency degree of regions in a scene. In semantically rich scenes, there are generally multiple salient regions. This condition requires a fixation prediction model to understand the relative importance relationship of multiple salient regions, that is, to identify which region is more important. In practice, existing fixation prediction models implicitly explore the relative importance relationship in the end-to-end training process while they do not work well. In this article, we propose a novel Relative Importance-aware Network (RINet) to explicitly explore the modeling of relative importance in fixation prediction. RINet perceives multi-scale local and global relative importance through the Hierarchical Relative Importance Enhancement (HRIE) module. Within a single scale subspace, on the one hand, HRIE module regards the similarity matrix as the local relative importance map to weight the input feature. On the other hand, HRIE module integrates a set of local relative importance maps into one map, defined as the global relative importance map, to grasp global relative importance. Moreover, we propose a Complexity-Relevant Focal (CRF) loss for network training. As such, we can progressively emphasize learning difficult samples for better handling the complicated scenarios, further improving the performance. The ablation studies confirm the contributions of key components of our RINet, and extensive experiments on five datasets demonstrate our RINet is superior to 28 relevant state-of-the-art models.
更多查看译文
关键词
Fixation prediction,relative importance,self-attention mechanism,complexity-relevant focal loss
AI 理解论文
溯源树
样例
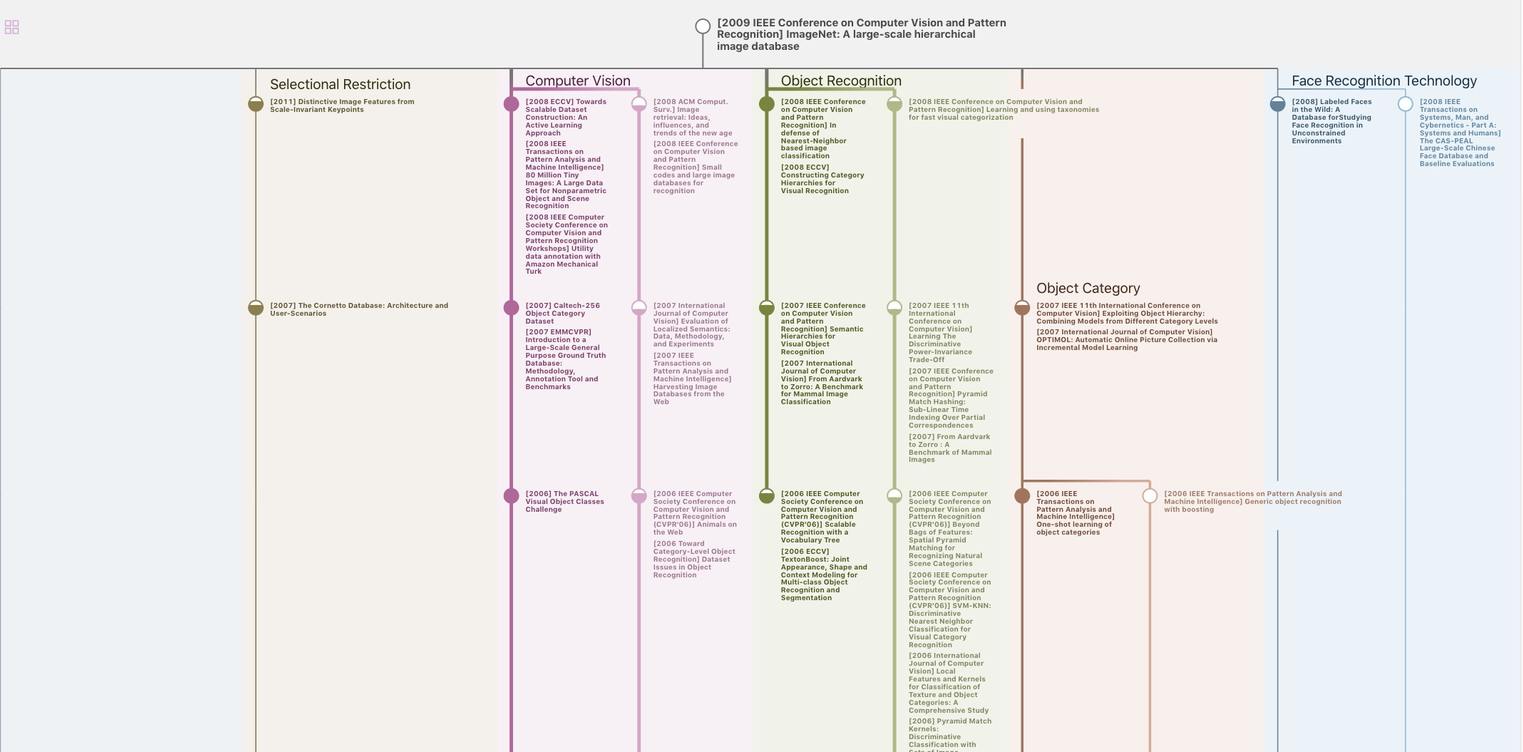
生成溯源树,研究论文发展脉络
Chat Paper
正在生成论文摘要