UIESC: An Underwater Image Enhancement Framework via Self-Attention and Contrastive Learning
IEEE TRANSACTIONS ON INDUSTRIAL INFORMATICS(2023)
摘要
Low contrast, color distortion, and blurred details are common problems that perplex vision-guided underwater robots. To this end, we propose an underwater image enhancement framework via self-attention and contrastive learning (UIESC) to solve these problems. In this article, local features and global dependencies are constructed through space and channel dual attention, and criss-cross attention is used to solve the high computational complexity of self-attention. Moreover, contrastive learning is introduced into network training as a loss function, and contrastive regularization ensures that the enhanced images are closer to clear positive samples and away from source negative samples. Finally, smoothed-histogram equalization is adopted for further optimization to accommodate complex and variable underwater scenes. Extensive experiments have shown that our framework outperforms state-of-the-art methods in underwater image enhancement tasks.
更多查看译文
关键词
Image enhancement,Image color analysis,Training,Informatics,Task analysis,Histograms,Feature extraction,Underwater tracking,Attention mechanism,contrastive learning,underwater image enhancement
AI 理解论文
溯源树
样例
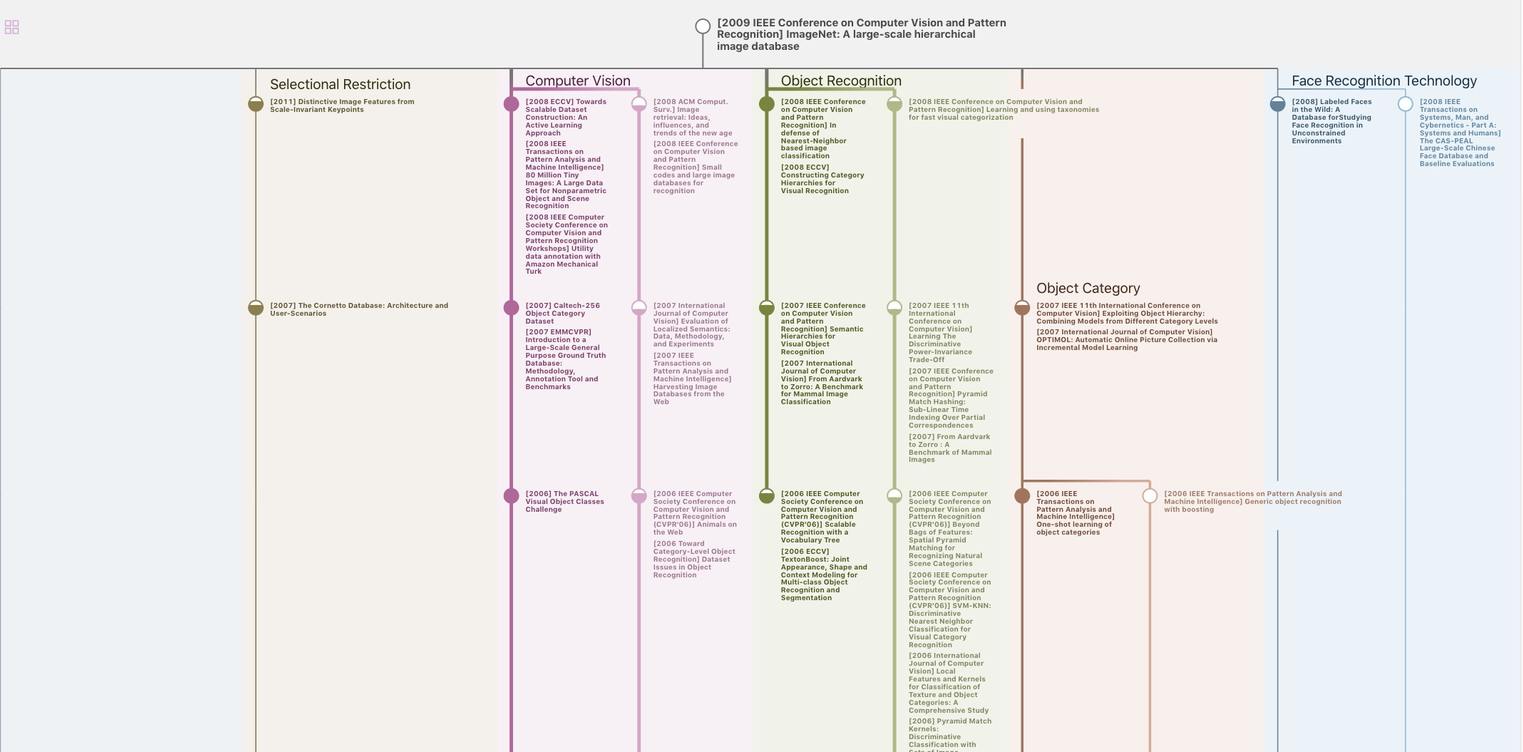
生成溯源树,研究论文发展脉络
Chat Paper
正在生成论文摘要