Convolutional neural network for normal fault scarp characterization :  application to the Trans-Mexican Volcanic Belt
crossref(2023)
摘要
<p>Normal fault markers in the landscape such as scarp are records of fault activity. The scarp morphology is used for exemple to estimate slip rates or rupture behaviors. The scarp morphology varies along strike, and needs to be estimated to assess this variation. Currently this is often a time-consuming step with expert-dependent results, often qualitative and with uncertainties that are difficult to estimate. To overcome those issues, we are developing a bayesian supervised machine learning method using convolutional neural networks (CNN) trained on a database of simulated profiles, called ScarpLearn. We train the CNN to use high resolution data (< 5m). From a 2D topographic profile across normal fault scarps, ScarpLearn is able to automatically give the scarp height with an uncertainty and to illuminate the area of the profile containing the scarp. We apply ScarpLearn for the characterization of normal active faults in the Trans-Mexican Volcanic Belt. This region is a slow deforming area (~0.2±0.05 mm/yr), which extends over more than 800km, crossed  by more than 600 potentially active faults but less than 5% of those have been correctly characterized by paleoseismological studies. In this context an automatic method to characterize the escarpments in a global, reproductible, robust (not expert-dependent) quantitative way will be highly valuable and a great step towards a better characterization of the seismic hazard of the region. In particular we tested our approach across the Ameca-Ahuisculco fault system, and by comparing ScarpLearn with with other methods based on profile analisis (not based on deep learning), we explore the advantages (computation time, accuracy, uncertainties) that deep learning methods bring, as well as the current limits (such as bias and dependance of the resolution).</p>
更多查看译文
AI 理解论文
溯源树
样例
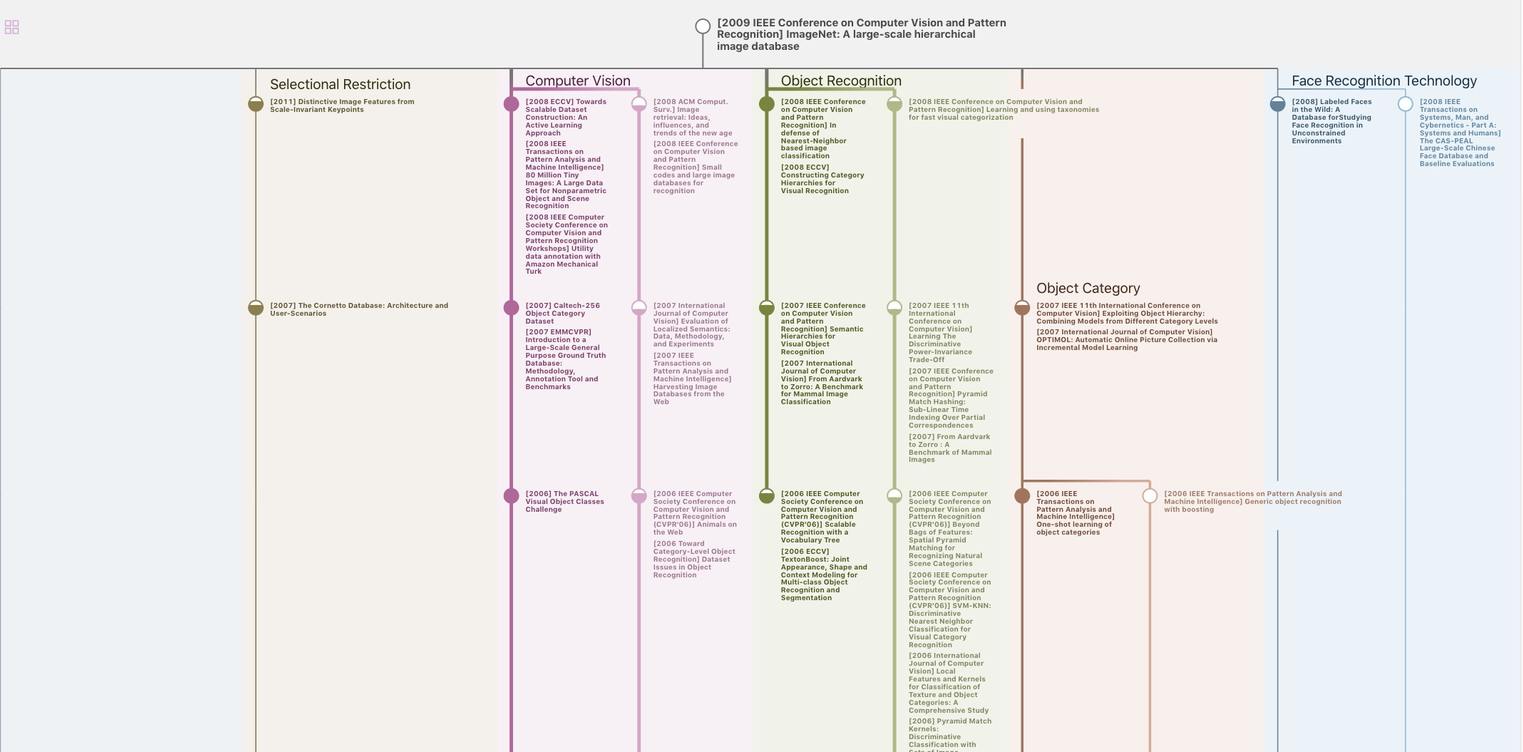
生成溯源树,研究论文发展脉络
Chat Paper
正在生成论文摘要