Large scale detection of plastic covered crops using multispectral and SAR satellite data
crossref(2023)
摘要
<p>The use of plastic in agriculture (plasticulture) started together with the rise of plastic in global markets, around 1950. Nowadays, plastic plays a key role in agriculture as an inexpensive, lightweight and resistant material. In European vegetable production, around 80% of plastic use is attributed to plastic films for crop covers. While meeting the needs of both producers and consumers (e.g., higher yields, early harvest, availability of out-of-season products), serious concerns have been raised about end-of-life management of plastic films. Different management practices, crop and film types are potentially controlling the amount of macro- and microplastic residues that remain in soil. While part of the scientific studies is improving our understanding of the environmental risks of plasticulture, a tool to monitor and quantify its extent at large scale (e.g., regions up to countries) is still lacking. The presence of publicly and freely available satellite data with increasing temporal and spatial resolution, coupled with increasing computing capabilities, qualify satellite remote sensing as a potential data source for large scale plasticulture monitoring. The challenge of detecting plastic covered crops by satellite data stimulated a growing number of papers. However, most of the literature focuses on local plasticulture hotspots, while a few authors recently tried to identify either greenhouses or plastic mulched farmlands (PMF) at larger scale. To the best of our knowledge, no attempts have been made for large scale plasticulture mapping in regions where different types of plastic covers are present (PMF, tunnels and greenhouses).</p> <p>In this research study we aim at identifying PMF, tunnels and greenhouses across Germany, one of the most active countries in the EU agricultural plastic film market. Google Earth Engine cloud computing capabilities were combined with Sentinel-1 and Sentinel-2 data coming from a whole year of acquisitions. In this context, time series analyses were supplied with a novel multi-image classification-based index (called Plastic Detection Frequency, PDF). The index was created by looping a random forest classifier over a yearly image collection to address the seasonality of temporary plastic covers (e.g., mulch foils, tunnels), which might not be caught by analysing time series with traditional methods alone. The results were evaluated in two German sub-regions (Cologne-Bonn region and Rhine-Valley south of Mainz), by combining ground truth sampling on Google Earth images and regional crop type databases. The overall accuracy assessed in the evaluation regions reaches 90% when plasticulture is distinguished between PMF, tunnels and greenhouses, while it exceeds 95% when the total area of plasticulture is mapped. Based on the feature rank, the PDF resulted to be the most important feature in the classification process. Moreover, the PDF showed higher values for crops typically associated with use of plastic films, which points at a strong relationship between the PDF and the presence of plastic covers. This study demonstrates the potential of an operational workflow for large scale plasticulture mapping and monitoring by synergising freely available optical and SAR satellite data.</p>
更多查看译文
AI 理解论文
溯源树
样例
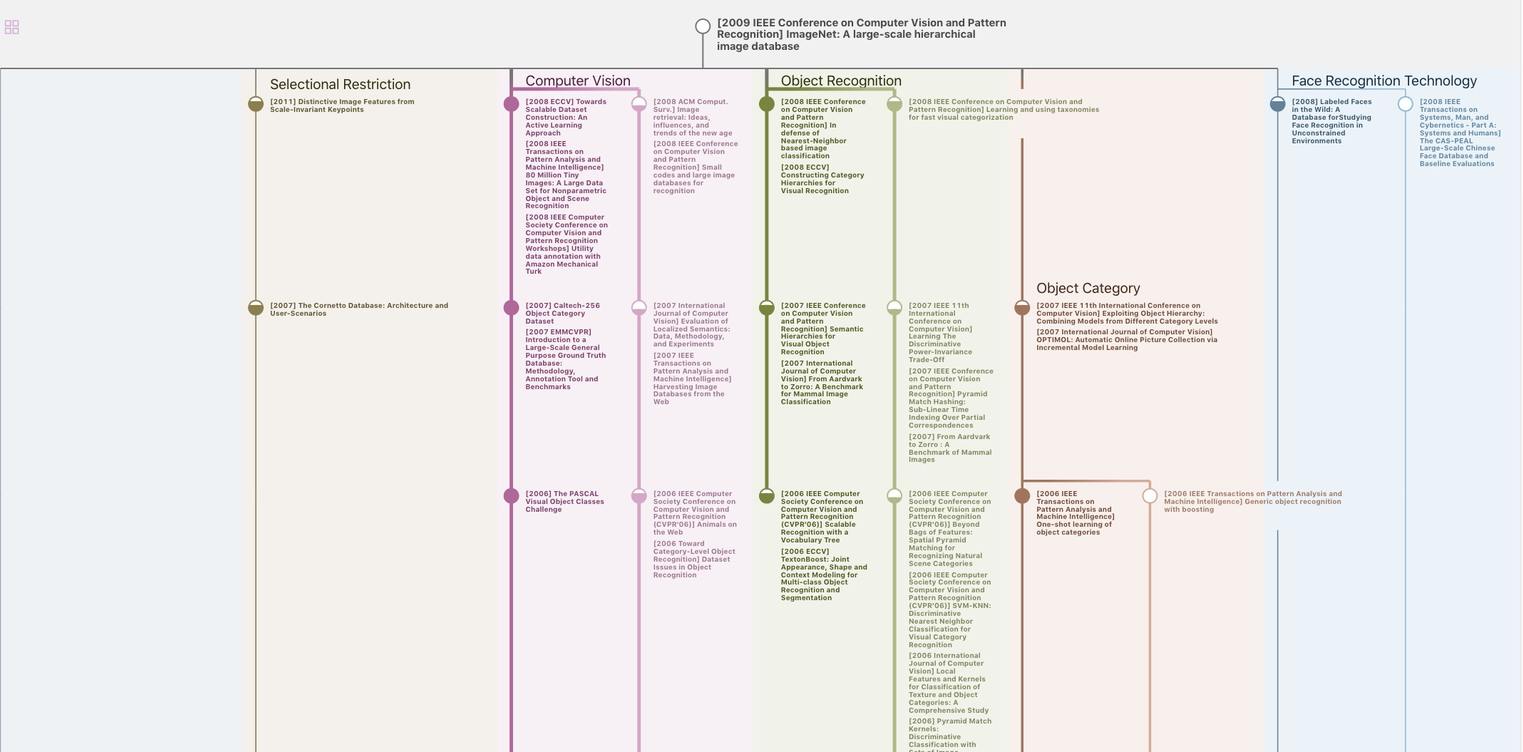
生成溯源树,研究论文发展脉络
Chat Paper
正在生成论文摘要