Time-sensitive prediction of NO2 concentration in China using an ensemble machine learning model from multi-source data
JOURNAL OF ENVIRONMENTAL SCIENCES(2024)
摘要
Nitrogen dioxide (NO2) poses a critical potential risk to environmental quality and pub-lic health. A reliable machine learning (ML) forecasting framework will be useful to pro -vide valuable information to support government decision-making. Based on the data from 1609 air quality monitors across China from 2014-2020, this study designed an ensemble ML model by integrating multiple types of spatial-temporal variables and three sub-models for time-sensitive prediction over a wide range. The ensemble ML model incorporates a residual connection to the gated recurrent unit (GRU) network and adopts the advantage of Trans-former, extreme gradient boosting (XGBoost) and GRU with residual connection network, resulting in a 4.1% +/- 1.0% lower root mean square error over XGBoost for the test results. The ensemble model shows great prediction performance, with coefficient of determina-tion of 0.91, 0.86, and 0.77 for 1-hr, 3-hr, and 24-hr averages for the test results, respectively. In particular, this model has achieved excellent performance with low spatial uncertainty in Central, East, and North China, the major site-dense zones. Through the interpretability analysis based on the Shapley value for different temporal resolutions, we found that the contribution of atmospheric chemical processes is more important for hourly predictions compared with the daily scale predictions, while the impact of meteorological conditions would be ever-prominent for the latter. Compared with existing models for different spa-tiotemporal scales, the present model can be implemented at any air quality monitoring station across China to facilitate achieving rapid and dependable forecast of NO2, which will help developing effective control policies. (c) 2023 The Research Center for Eco-Environmental Sciences, Chinese Academy of Sciences. Published by Elsevier B.V.
更多查看译文
关键词
Air quality prediction,Deep learning,Ensemble method,Nitrogen dioxide,Spatiotemporal covariates
AI 理解论文
溯源树
样例
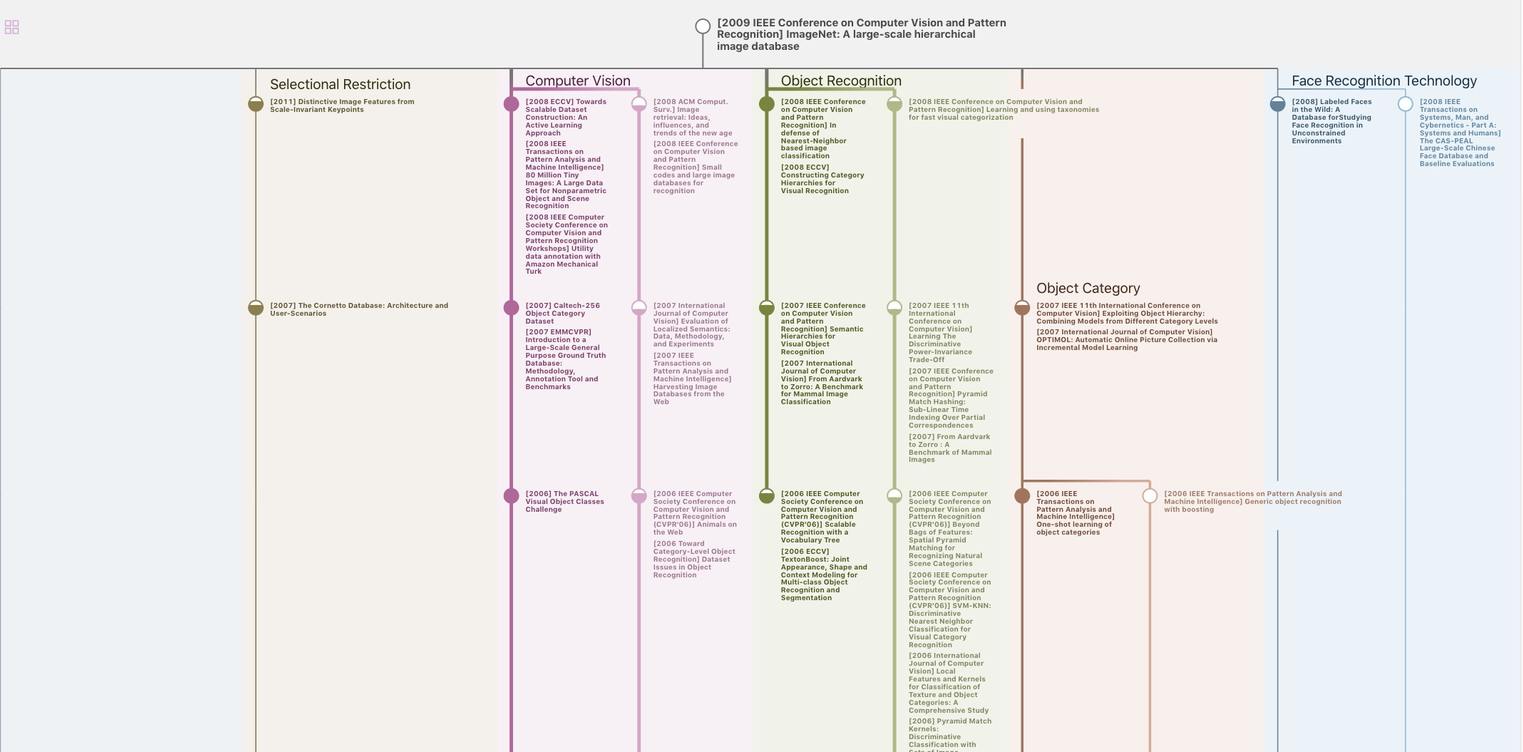
生成溯源树,研究论文发展脉络
Chat Paper
正在生成论文摘要