Why Seasonal Prediction of California Winter Precipitation Is Challenging
Bulletin of the American Meteorological Society(2022)
摘要
Abstract Despite an urgent demand for reliable seasonal prediction of precipitation in California (CA) due to the recent recurrent and severe drought conditions, our predictive skill for CA winter precipitation remains limited. October hindcasts by the coupled dynamical models typically show a correlation skill of about 0.3 for CA winter (November–March) precipitation. In this study, an attempt is made to understand the underlying processes that limit seasonal prediction skill for CA winter precipitation. It is found that only about 25% of interannual variability of CA winter precipitation can be attributed to influences by El Niño–Southern Oscillation (ENSO). Instead, the year-to-year CA winter precipitation variability is primarily due to circulation anomalies independent from ENSO, featuring a circulation center over the west coast United States as a portion of a short Rossby wave train pattern over the North Pacific. Analyses suggest that dynamical models show nearly no skill in predicting these ENSO-independent circulation anomalies, thus leading to limited predictive skill for CA winter precipitation. Low predictability of these ENSO-independent circulation anomalies is further demonstrated by a large ensemble of atmospheric-only climate model simulations. While low predictability of the ENSO-independent circulation anomalies could be due to chaotic internal atmospheric processes over the mid- to high latitudes, possible underexploited predictability sources for CA precipitation in models are also discussed. This study pinpoints an urgent need for improved understanding of the formation mechanisms of ENSO-independent circulation anomalies over the U.S. West Coast for a breakthrough in seasonal prediction of CA winter precipitation.
更多查看译文
关键词
california winter precipitation,seasonal prediction
AI 理解论文
溯源树
样例
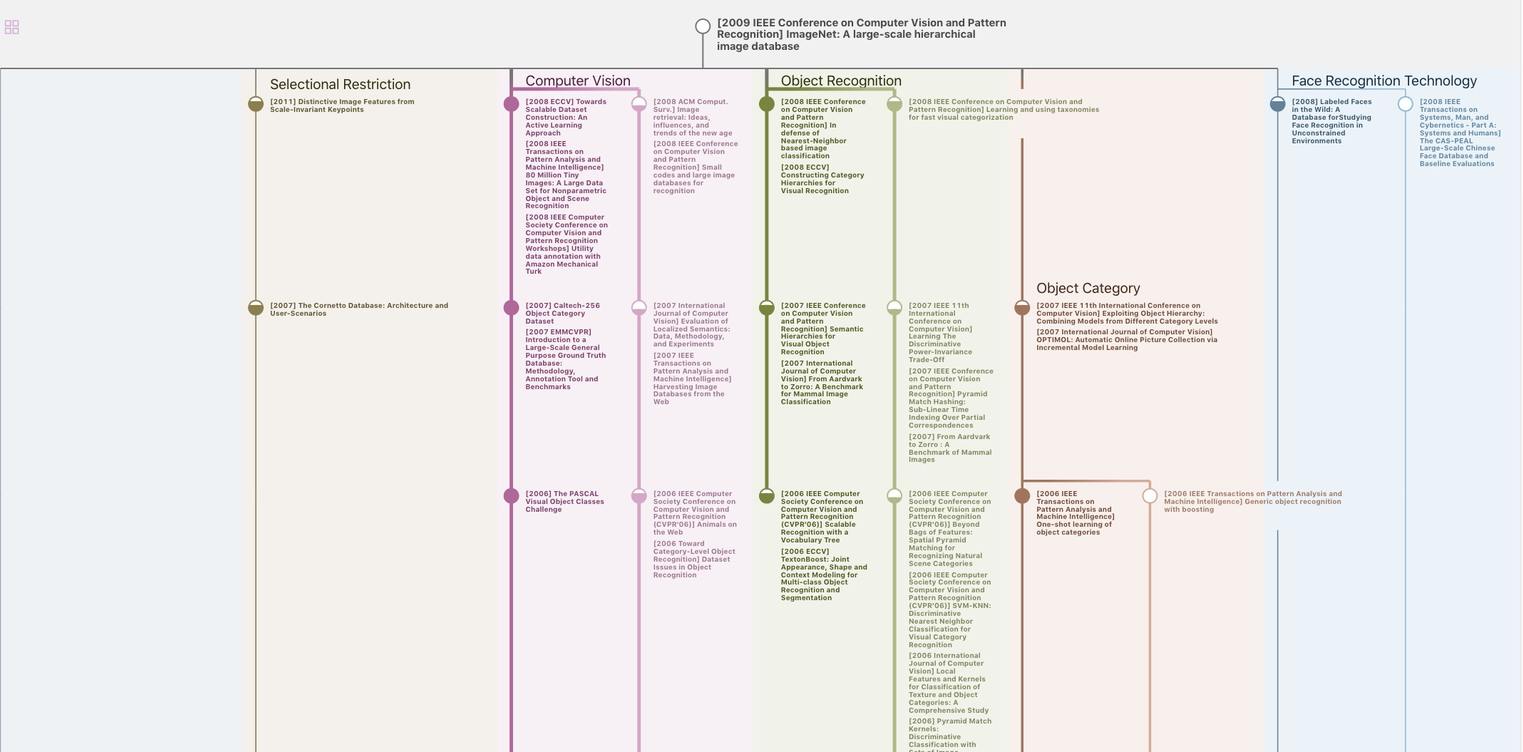
生成溯源树,研究论文发展脉络
Chat Paper
正在生成论文摘要