Multimodal contrastive learning for unsupervised video representation learning.
Electronic Imaging(2023)
摘要
In this paper, we propose a multimodal unsupervised video learning algorithm designed to incorporate information from any number of modalities present in the data. We cooperatively train a network corresponding to each modality: at each stage of training, one of these networks is selected to be trained using the output of the other networks. To verify our algorithm, we train a model using RGB, optical flow, and audio. We then evaluate the effectiveness of our unsupervised learning model by performing action classification and nearest neighbor retrieval on a supervised dataset. We compare this triple modality model to contrastive learning models using one or two modalities, and find that using all three modalities in tandem provides a 1.5% improvement in UCF101 classification accuracy, a 1.4% improvement in R@1 retrieval recall, a 3.5% improvement in R@5 retrieval recall, and a 2.4% improvement in R@10 retrieval recall as compared to using only RGB and optical flow, demonstrating the merit of utilizing as many modalities as possible in a cooperative learning model.
更多查看译文
关键词
multimodal contrastive learning,unsupervised video representation learning
AI 理解论文
溯源树
样例
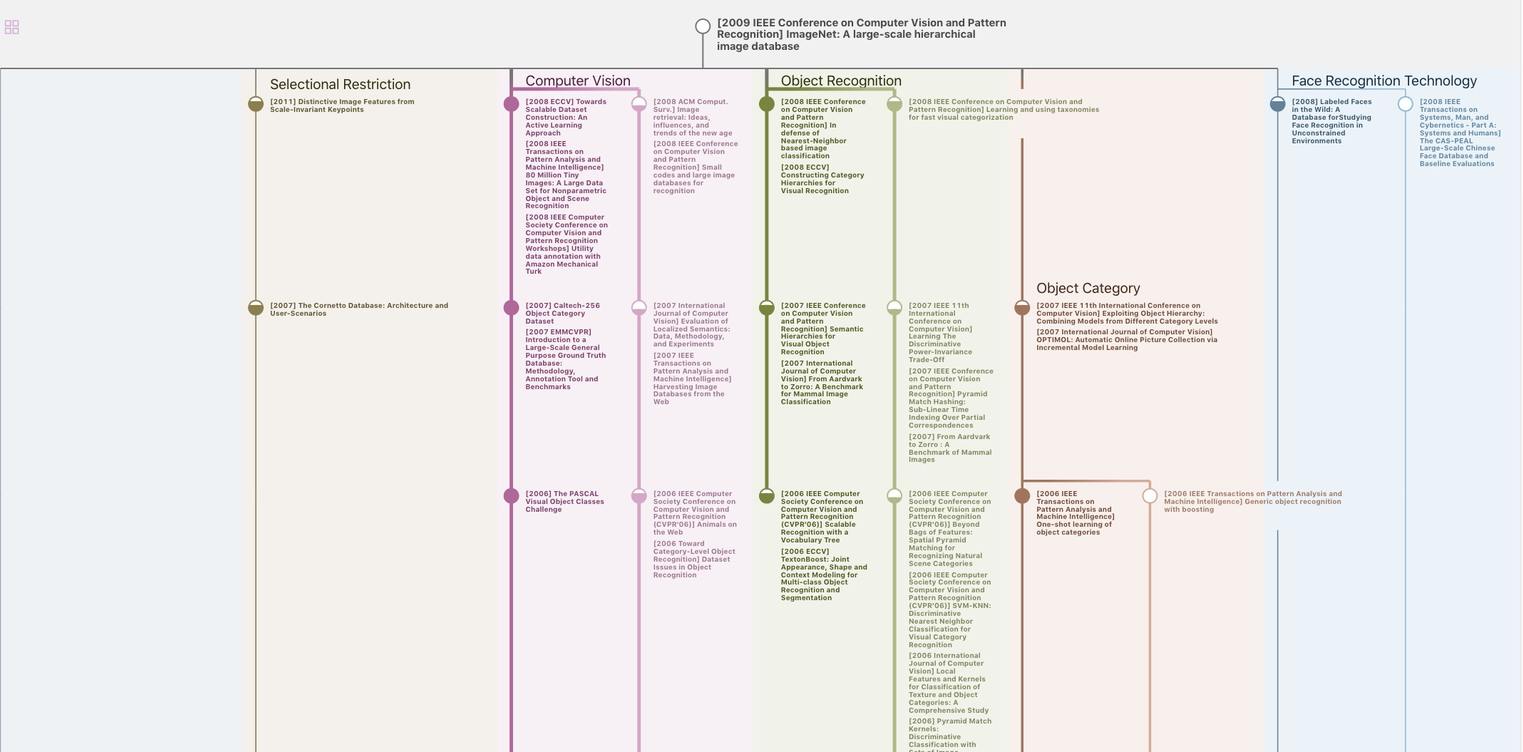
生成溯源树,研究论文发展脉络
Chat Paper
正在生成论文摘要