Rapid determination of the main components of corn based on near-infrared spectroscopy and a BiPLS-PCA-ELM model
Applied Optics(2023)
摘要
To evaluate corn quality quickly, the feasibility of near-infrared spectroscopy (NIRS) coupled with chemometrics was analyzed to detect the moisture, oil, protein, and starch content in corn. A backward interval partial least squares (BiPLS)-principal component analysis (PCA)-extreme learning machine (ELM) quantitative analysis model was constructed based on BiPLS in conjunction with PCA and the ELM. The selection of characteristic spectral intervals was accomplished by BiPLS. The best principal components were determined by the prediction residual error sum of squares of Monte Carlo cross validation. In addition, a genetic simulated annealing algorithm was utilized to optimize the parameters of the ELM regression model. The established regression models for moisture, oil, protein, and starch can meet the demand for corn component detection with the prediction determination coefficients of 0.996, 0.990, 0.974, and 0.976; the prediction root means square errors of 0.018, 0.016, 0.067, and 0.109; and the residual prediction deviations of 15.704, 9.741, 6.330, and 6.236, respectively. The results show that the NIRS rapid detection model has higher robustness and accuracy based on the selection of characteristic spectral intervals in conjunction with spectral data dimensionality reduction and nonlinear modeling and can be used as an alternative strategy to detect multiple components in corn rapidly.
更多查看译文
关键词
corn,spectroscopy,rapid determination,near-infrared,bipls-pca-elm
AI 理解论文
溯源树
样例
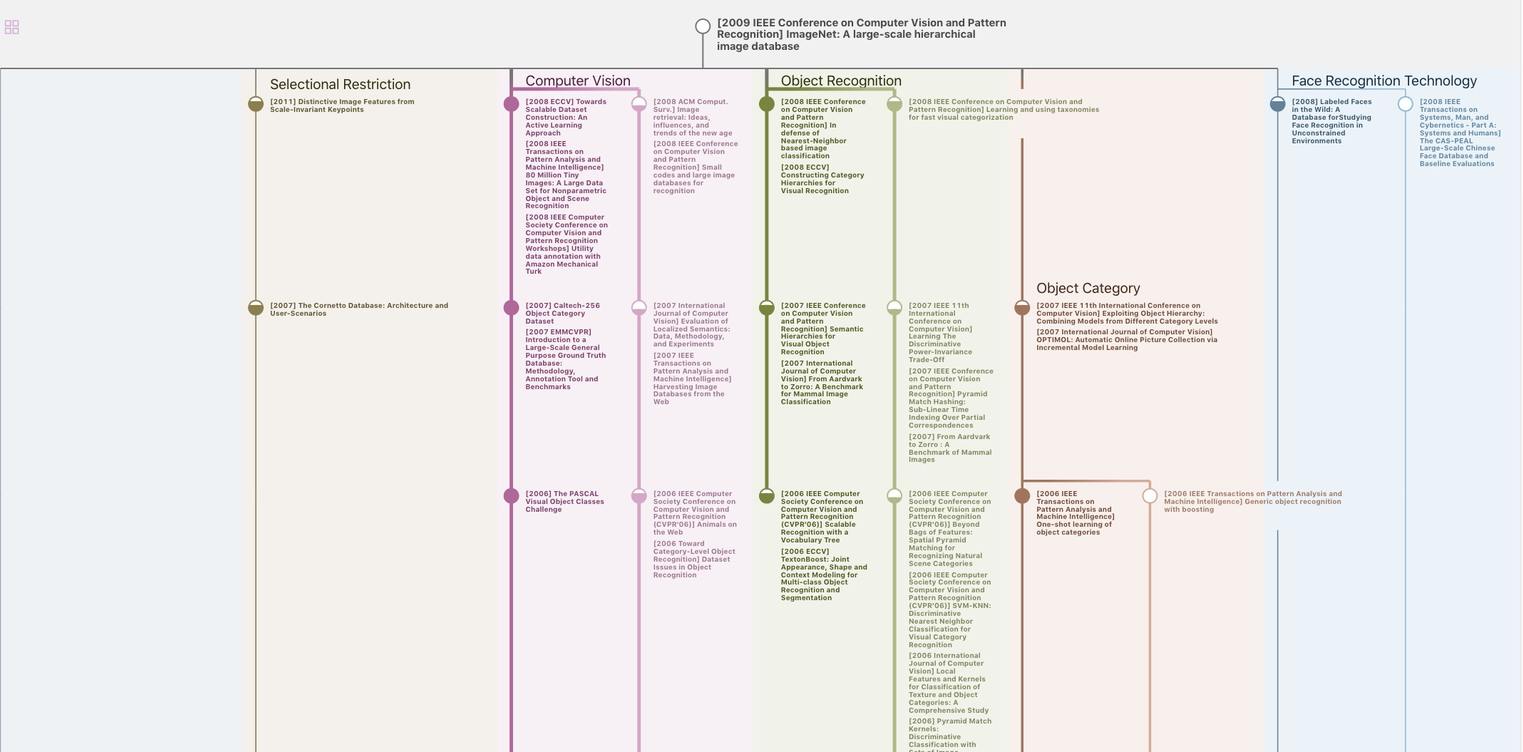
生成溯源树,研究论文发展脉络
Chat Paper
正在生成论文摘要