Refined Knowledge Transfer for Language-Based Person Search
IEEE TRANSACTIONS ON MULTIMEDIA(2023)
摘要
This paper proposes a novel method, named Refined Knowledge Transfer (RKT), for language-based person search. Existing state-of-the-art methods do not deal with knowledge imbalance between image and text. In detail, textual identity knowledge is limited, but the image contains more identity knowledge. We propose Cross-Modal Knowledge Transfer (CMKT) to enhance textual identity knowledge by image to address this problem. Besides, multiple texts of one image include more identity knowledge than a single text. Thus, we propose Intra-Modal Knowledge Transfer (IMKT) to enhance textual identity knowledge by other texts. These two types of knowledge transfer will enhance the identity knowledge in text. Additionally, by considering that identity-irrelevant knowledge is transferred to text, we propose Knowledge Refiner (KR) to refine the knowledge in text. KR is capable of preserving identity knowledge and discarding identity-irrelevant knowledge. By combining CMKT, IMKT, and KR, RKT makes textual identity knowledge more salient. Extensive experiments show the state-of-the-art performance of RKT on the CUHK-PEDES and our proposed PRW-PEDES-CN datasets. In addition, the decent generalization ability of RKT is also validated on the Flickr30K, CUB, and Flowers datasets.
更多查看译文
关键词
Language-based person search,knowledge enhancement,knowledge enhancement,cross-modal knowledge transfer,intra-modal knowledge transfer,intra-modal knowledge transfer,knowledge refiner
AI 理解论文
溯源树
样例
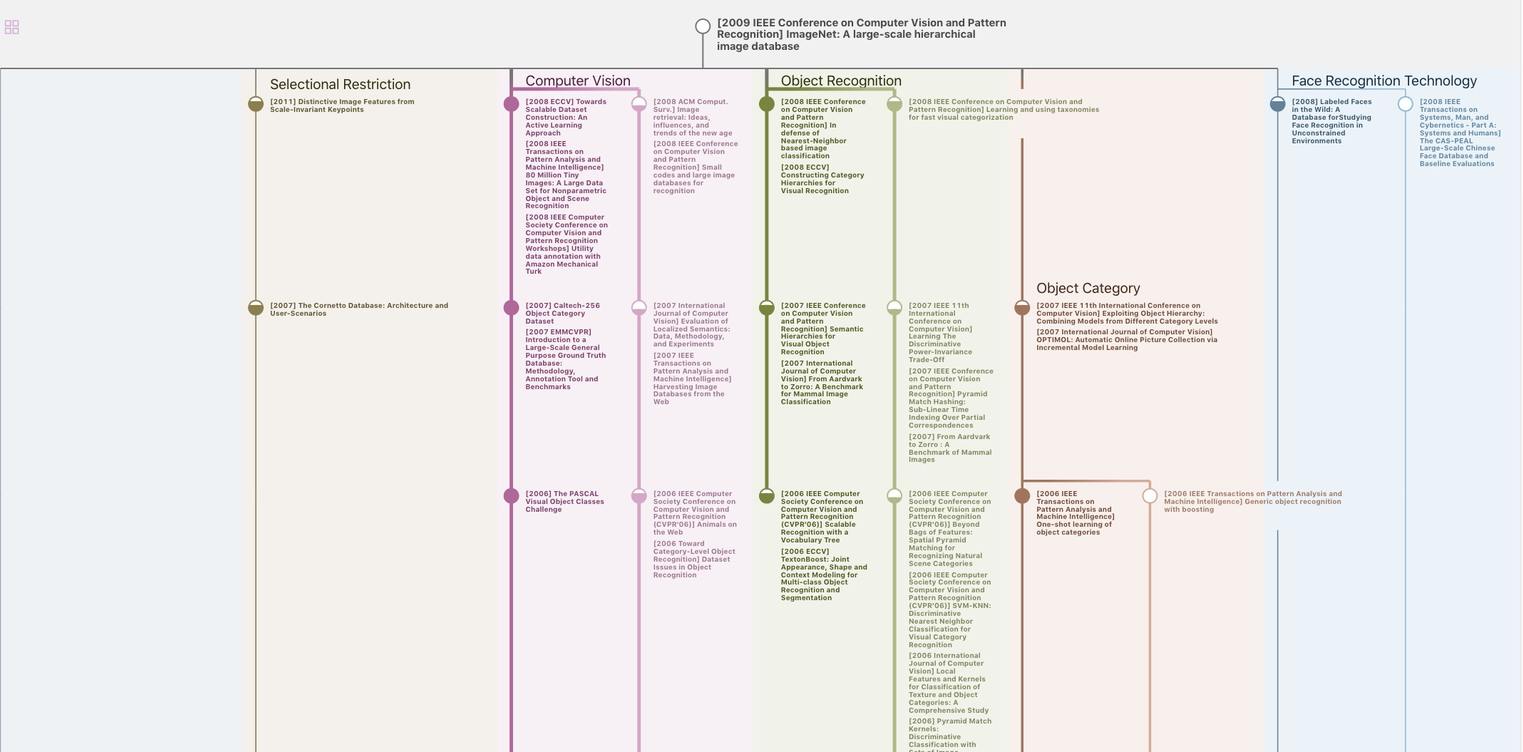
生成溯源树,研究论文发展脉络
Chat Paper
正在生成论文摘要