MCNet: Magnitude consistency network for domain adaptive object detection under inclement environments
PATTERN RECOGNITION(2024)
摘要
Deep learning-based object detection methods have achieved promising results in normal scenarios, while such methods often fail to locate objects from the disturbed images captured in inclement environments. Most existing methods utilized denoise modules to assist the detection network or exploit prior knowledge to reduce the environment interference remaining in the features, which ignores the essential role of the frequency element. From a novel perspective, we observe that the inclement environment alters the frequency content in the features, which in turn crushes the detection. To tickle this problem, we present the Magnitude Consistency Network (MCNet) to distill the irrelevant contents in the frequency domain. The MCNet is composed of the detection network and the magnitude corrector. The detection network is able to locate and classify objects. However, in inclement environments, the crucial information about the objects of interest is disrupted by the nuisance noise introduced from the inclement environments. The magnitude corrector can recover relevant information about the objects in the frequency domain by distilling the irrelevant factors and refining the affected features. Alternately optimizing the magnitude corrector and the detection network gradually makes the frequency content between the disturbed image and the clear image to be consistent. By distilling the irrelevant noise in the feature, the detection network can learn domain-invariant representations. Extensive experiments prove that the proposed method is effective and outperforms existing methods by a clear margin on four datasets.
更多查看译文
关键词
Object detection,Inclement environments,Frequency domain,Magnitude spectrum
AI 理解论文
溯源树
样例
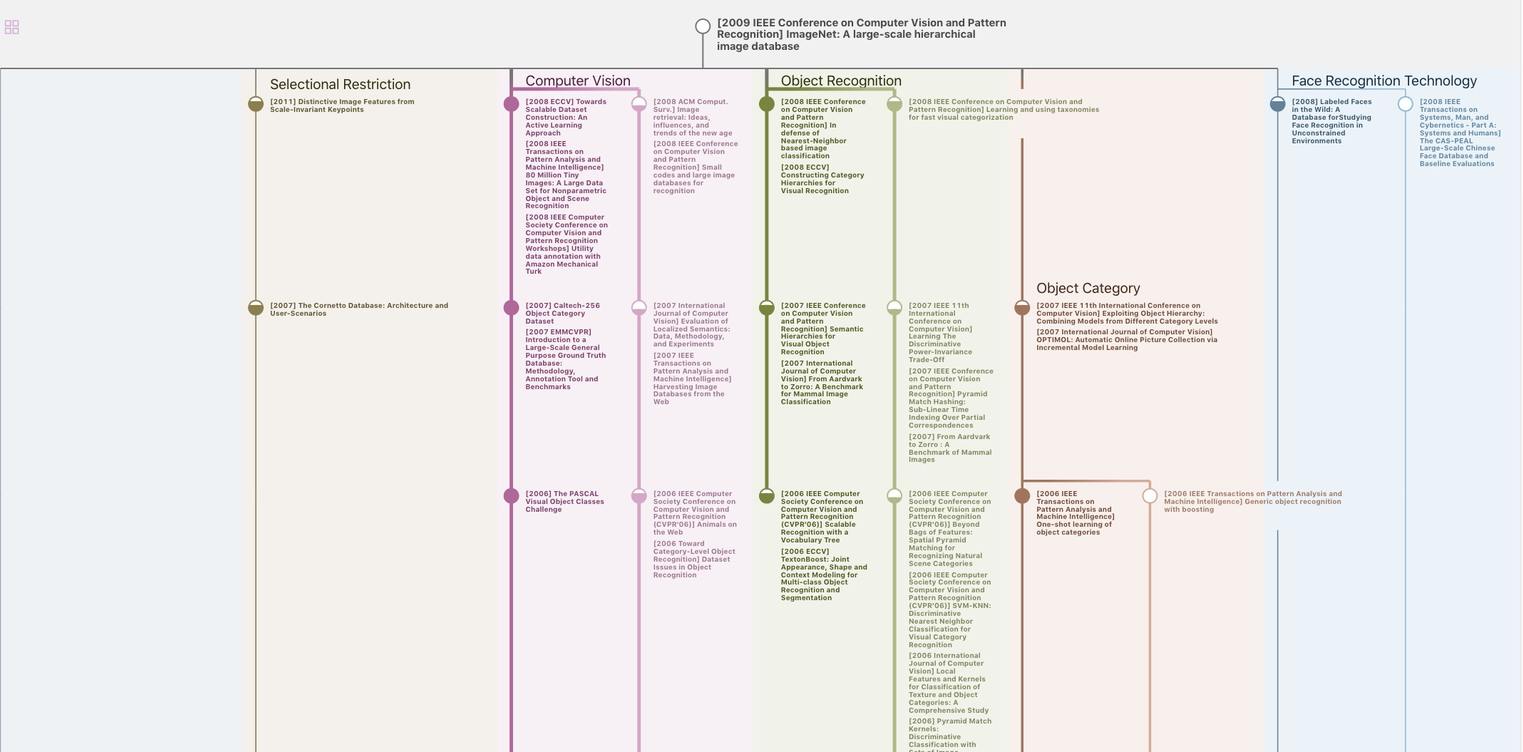
生成溯源树,研究论文发展脉络
Chat Paper
正在生成论文摘要