(ECOC 2022) A Flexible and Scalable ML-Based Diagnosis Module for Optical Networks: A Security Use Case
Journal of Optical Communications and Networking(2023)
摘要
To support the pervasive digital evolution, optical network infrastructures must be able to quickly and effectively adapt to changes arising from traffic dynamicity or external factors such as faults and attacks. Network automation is crucial for enabling dynamic, scalable, resource-efficient, and trustworthy network operations. Novel telemetry solutions enable optical network management systems to obtain fine-grained monitoring data from devices and channels as the first step toward the near-real-time diagnosis of anomalies such as security threats and soft failures. However, the collection of large amounts of data creates a scalability challenge related to processing the data within the desired monitoring cycle regardless of the number of optical services being analyzed. This paper proposes a module that leverages the cloud native software deployment approach to achieve near-real-time machine learning (ML)-assisted diagnosis of optical channels. The results obtained over an emulated physical-layer security scenario demonstrate that the architecture successfully scales the necessary components according to the computational load and consistently achieves the desired monitoring cycle duration over a varying number of monitored optical channels.
更多查看译文
关键词
Monitoring, Optical fiber networks, Optical feedback, Task analysis, Optical devices, Security, Scalability
AI 理解论文
溯源树
样例
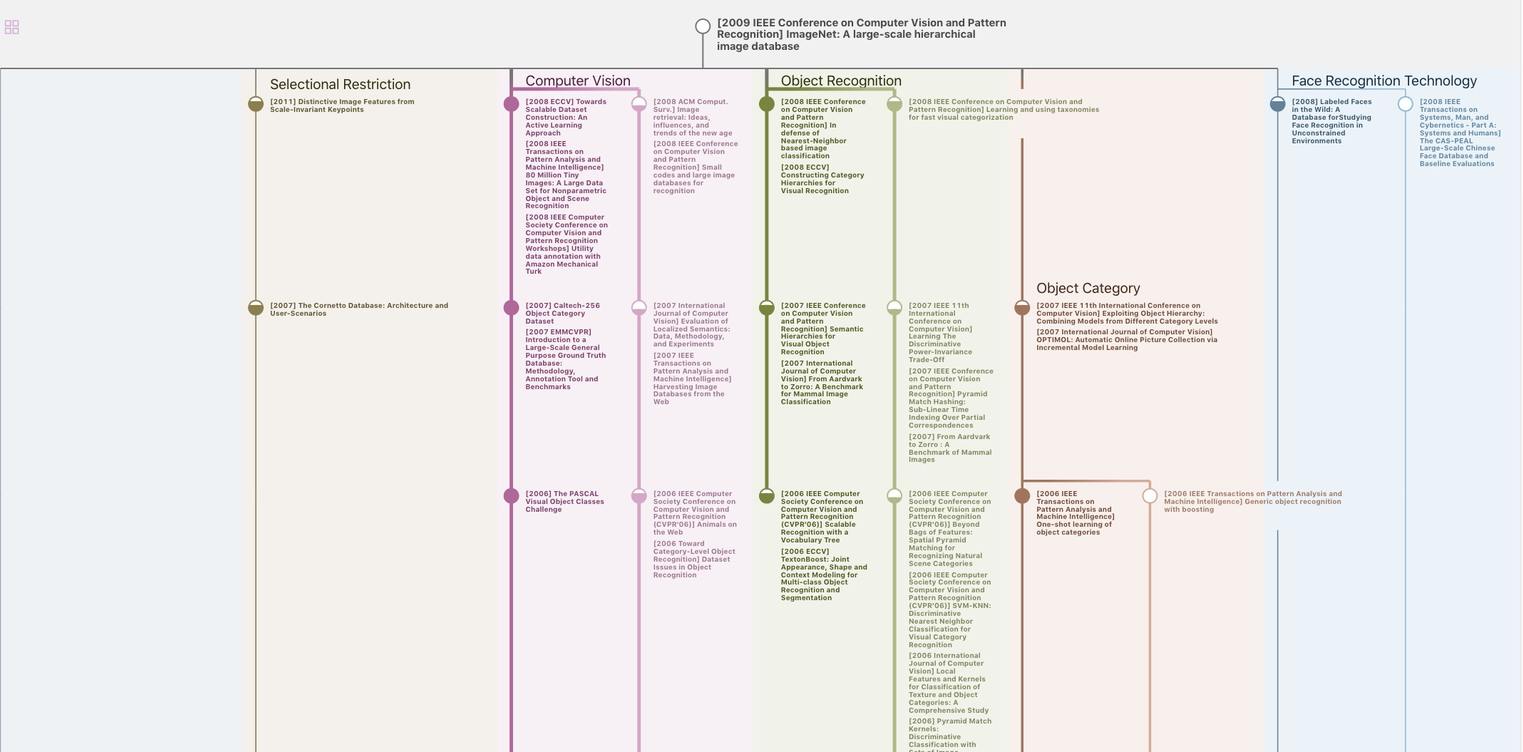
生成溯源树,研究论文发展脉络
Chat Paper
正在生成论文摘要