Efficient Index Learning via Model Reuse and Fine-tuning
2023 IEEE 39th International Conference on Data Engineering Workshops (ICDEW)(2023)
摘要
Learned indices using machine learning techniques have demonstrated potential as alternatives to traditional indices such as B-trees in both query time and memory. However, a well fitted learned index requires significant space consumption to train models and tune parameters. Furthermore, fast training methods—ones that train in one pass—may not learn the data distribution well. To consider both the fitness to data distribution and building efficiency, in this paper, we apply pre-trained models and fine-tuning to accelerate the building of learned indices by 30.4% and improve lookup efficiency by up to 24.4% on real datasets and 22.5% on skewed datasets.
更多查看译文
关键词
learned index, model reuse, fine-tuning
AI 理解论文
溯源树
样例
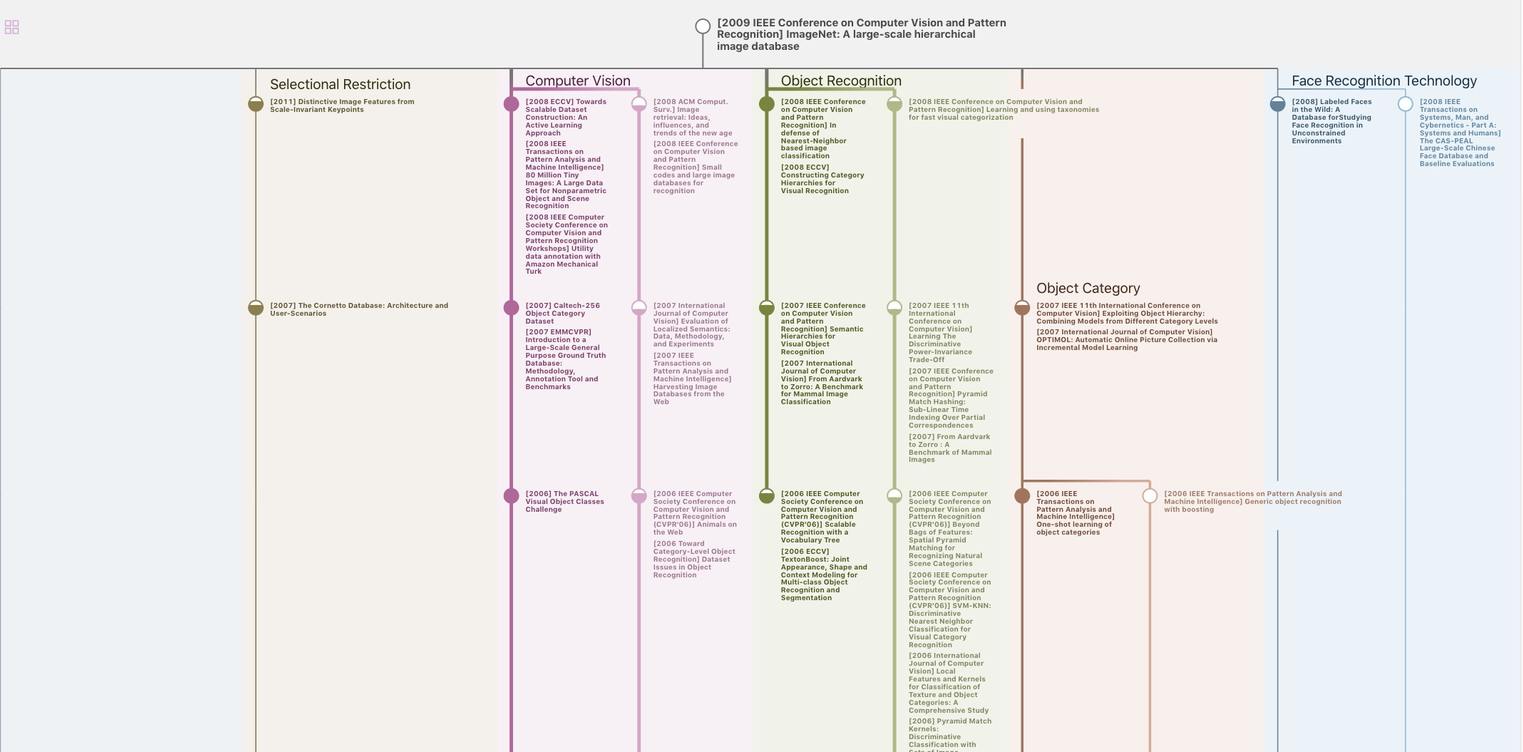
生成溯源树,研究论文发展脉络
Chat Paper
正在生成论文摘要