Adversarial Attacks and Batch Normalization: A Batch Statistics Perspective.
IEEE Access(2023)
摘要
Batch Normalization (BatchNorm) is an effective architectural component in deep learning models that helps to improve model performance and speed up training. However, it has also been found to increase the vulnerability of models to adversarial attacks. In this study, we investigate the mechanism behind this vulnerability and took first steps towards a solution called RobustNorm. We observed that adversarial inputs tend to shift the distributions of the output of the BatchNorm layer, leading to inaccurate train-time statistics and increased vulnerability. Through a series of experiments on various architectures and datasets, we confirm our hypothesis. We also demonstrate the effectiveness of RobustNorm in improving the robustness of models under adversarial perturbation while maintaining the benefits of BatchNorm.
更多查看译文
关键词
Batch normalization,adversarial robustness,transfer learning
AI 理解论文
溯源树
样例
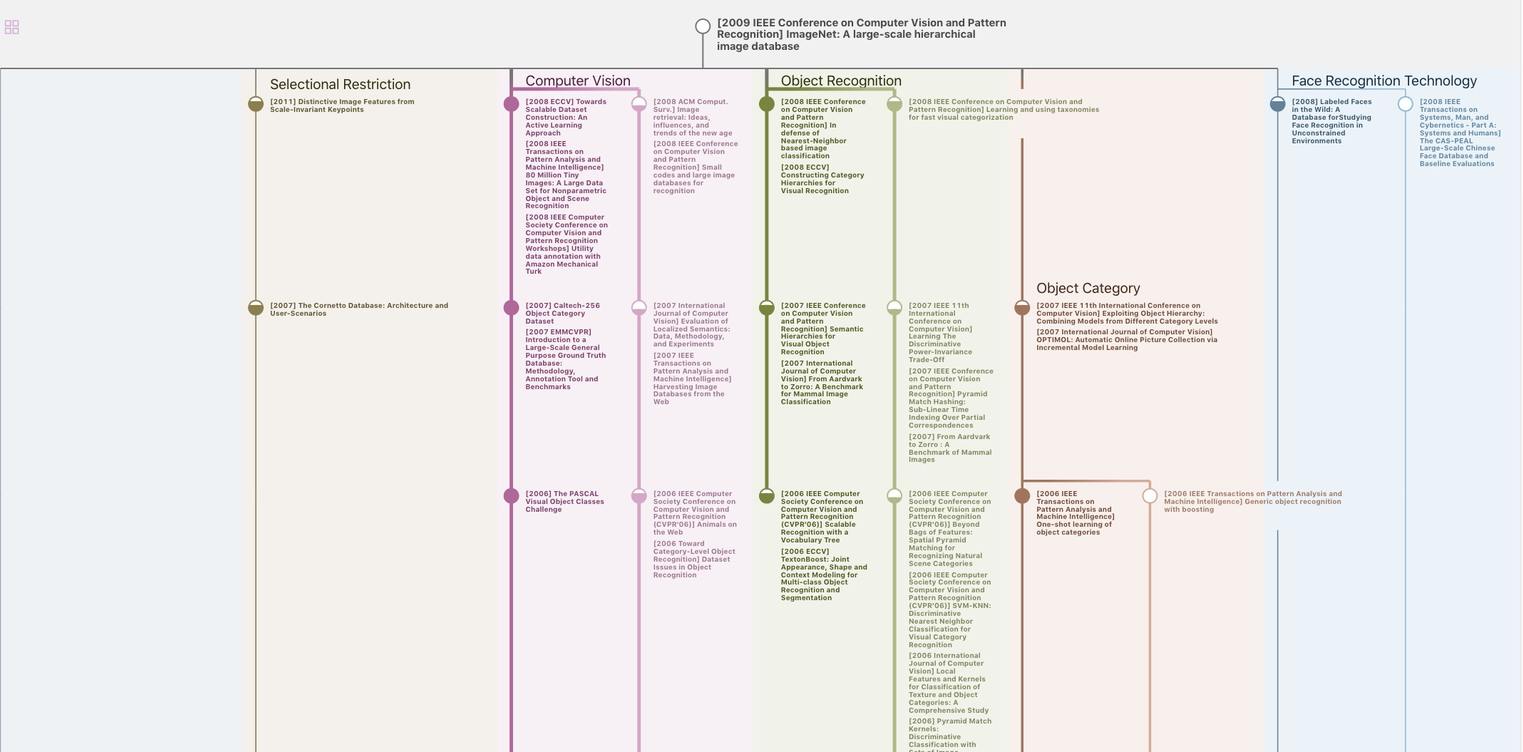
生成溯源树,研究论文发展脉络
Chat Paper
正在生成论文摘要