Power system transient voltage vulnerability assessment based on knowledge visualization of CNN
INTERNATIONAL JOURNAL OF ELECTRICAL POWER & ENERGY SYSTEMS(2024)
摘要
Because of the increasing proportion of grid-connected converters, the transient voltage problem has increased the risk of the secure and stable operation of power systems. As the extension of transient voltage stability assessment (TVSA), transient voltage vulnerability assessment is the key technique to detect the crucial elements which can cause the insecurity risk of power system transient voltage. Although deep learning-based TVSA models have demonstrated the high accuracy, it is more important to display the knowledge learnt by the models and utilize it to recognize the weakness of transient voltage. This paper presents a transient voltage vulnerability assessment method by knowledge visualization of convolutional neural network (CNN). First, the image-form sample construction method is proposed to characterize the power system operating condition, which prepares for training CNN-based TVSA model. Then, the knowledge in TVSA model is visualized based on Gradientweighted Class Activation Mapping (Grad-CAM) and Activation Maximization (AM) algorithms, which is analyzed from the perspective of power system stability. Finally, a comprehensive vulnerability indicator is proposed for identify the weakness of transient voltage, which combines the knowledge inside the TVSA model and the indicators with clear physical meaning. Simulation results of the New England 39-bus test system and the real Shandong provincial power grid in China demonstrate that the visualization makes the model more reliable and the proposed method can accurately identify the vulnerable branches.
更多查看译文
关键词
Power system,Transient voltage vulnerability assessment,Knowledge visualization,Grad-CAM,Convolutional neural network
AI 理解论文
溯源树
样例
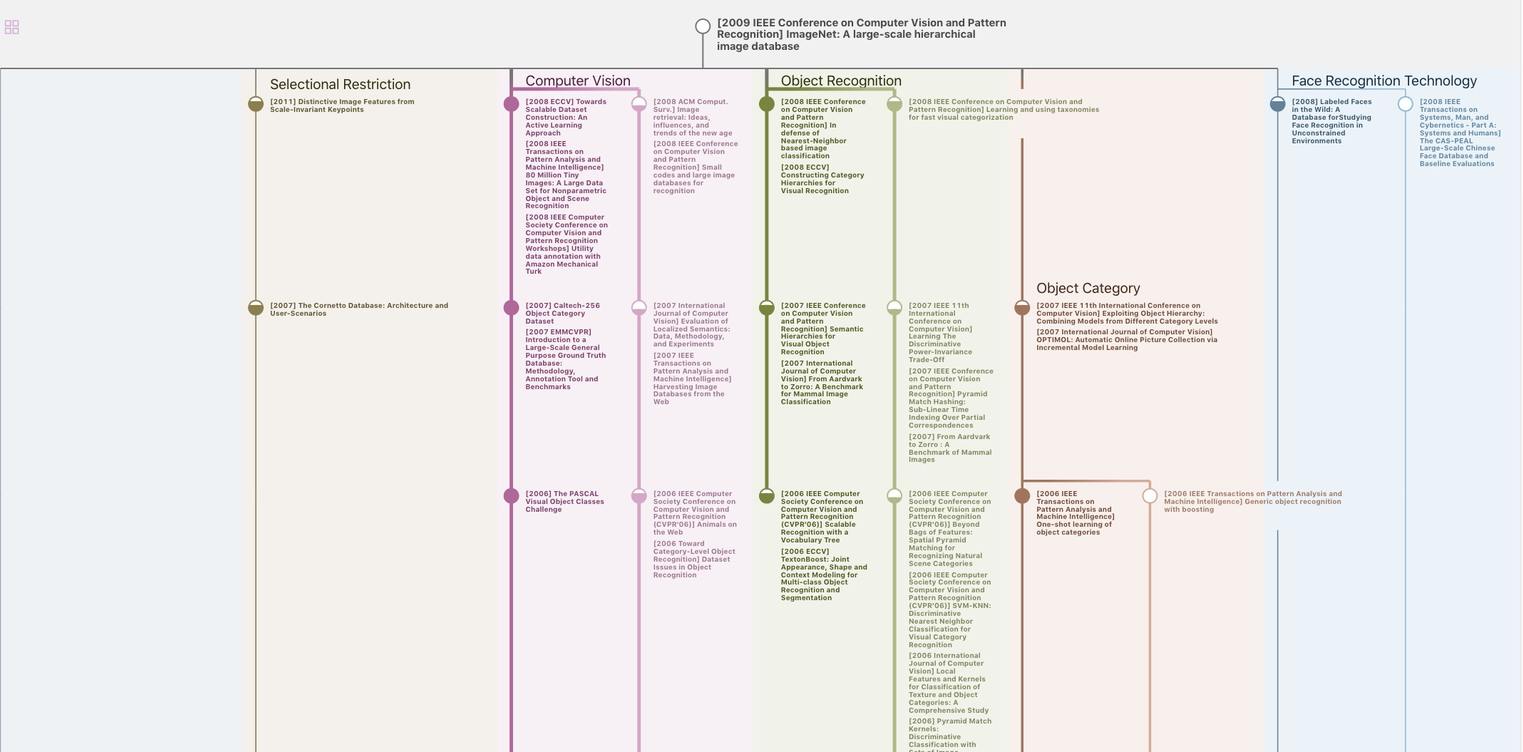
生成溯源树,研究论文发展脉络
Chat Paper
正在生成论文摘要