Selection of linear mixed‐effects models for clustered data
Scandinavian Journal of Statistics(2023)
摘要
We consider model selection for linear mixed-effects models with clustered structure, where conditional Kullback-Leibler (CKL) loss is applied to measure the efficiency of the selection. We estimate the CKL loss by substituting the empirical best linear unbiased predictors (EBLUPs) into random effects with model parameters estimated by maximum likelihood. Although the BLUP approach is commonly used in predicting random effects and future observations, selecting random effects to achieve asymptotic loss efficiency concerning CKL loss is challenging and has not been well studied. In this paper, we propose addressing this difficulty using a conditional generalized information criterion (CGIC) with two tuning parameters. We further consider a challenging but practically relevant situation where the number, m$$ m $$, of clusters does not go to infinity with the sample size. Hence the random-effects variances are not consistently estimable. We show that via a novel decomposition of the CKL risk, the CGIC achieves consistency and asymptotic loss efficiency, whether m$$ m $$ is fixed or increases to infinity with the sample size. We also conduct numerical experiments to illustrate the theoretical findings.
更多查看译文
关键词
asymptotic loss efficiency, conditional Akaike's information criterion, conditional Kullback-Leibler loss, inconsistent estimation, random effects, risk decomposition, unbalanced data
AI 理解论文
溯源树
样例
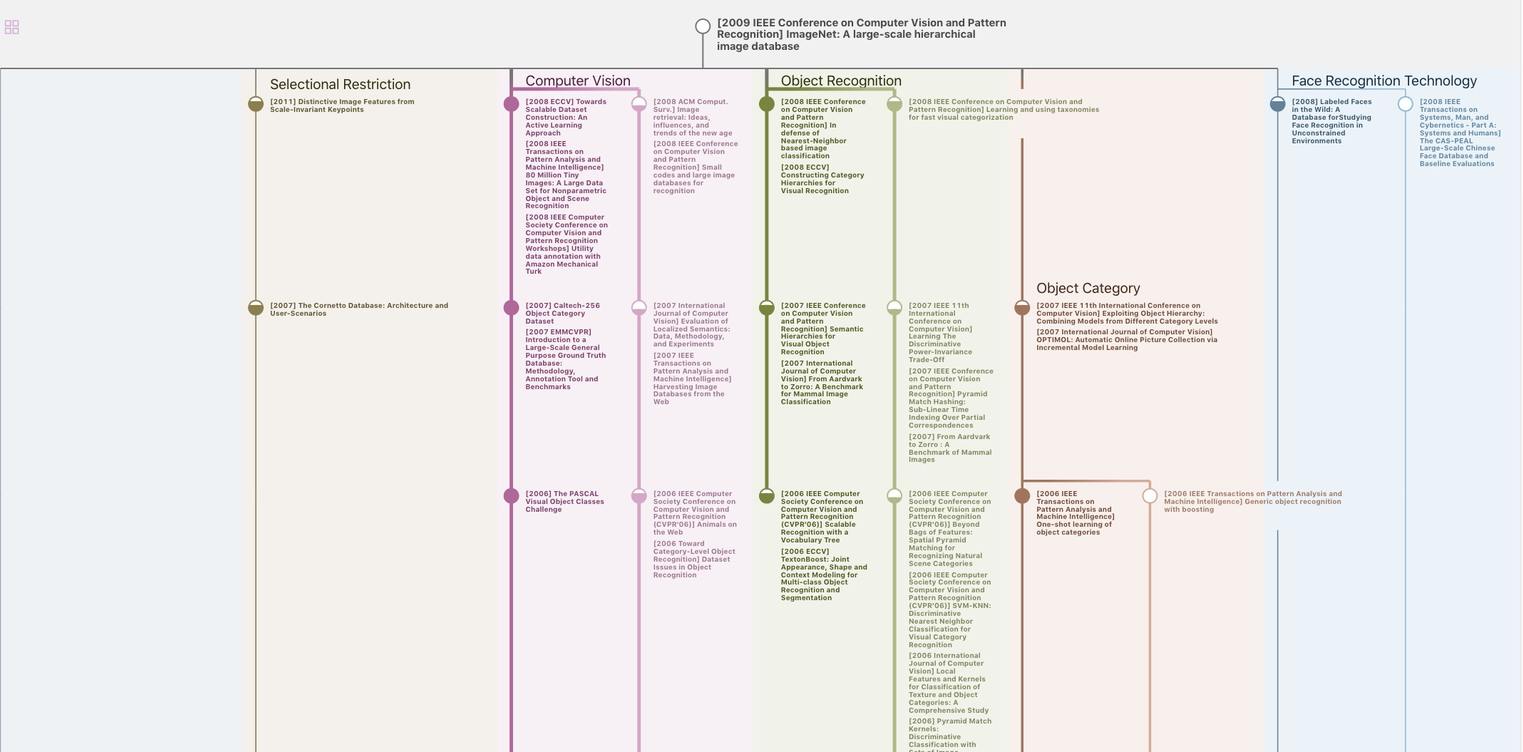
生成溯源树,研究论文发展脉络
Chat Paper
正在生成论文摘要