Optimum Design for Ill-Conditioned Models: K--Optimality and Stable Parameterizations
SSRN Electronic Journal(2023)
摘要
Nonlinear regression is frequently used to fit nonlinear relations between response variables and regressors, for process data. The procedure involves the minimization of the square norm of the residuals with respect to the model parameters. Nonlinear least squares may lead to parametric collinearity, multiple optima and computational inefficiency. One of the strategies to handle collinearity is model reparameterization, i.e. the replacement of the original set of parameters by another with increased orthogonality properties. In this paper we propose a systematic strategy for model reparameterization based on the response surface generated from a carefully chosen set of points. This is illustrated with the support points of locally K-optimal experimental designs, to generate a set of analytical equations that allow the construction of a transformation to a set of parameters with better orthogonality properties. Recognizing the difficulties in the generalization of the technique to complex models, we propose a related alternative approach based on first-order Taylor approximation of the model. Our approach is tested both with linear and nonlinear models. The Variance Inflation Factor and the condition number as well as the orientation and eccentricity of the parametric confidence region are used for comparisons.
更多查看译文
关键词
K-optimal design of experiments, Semidefinite programming, Nonlinear regression, Model reparameterization, Support points
AI 理解论文
溯源树
样例
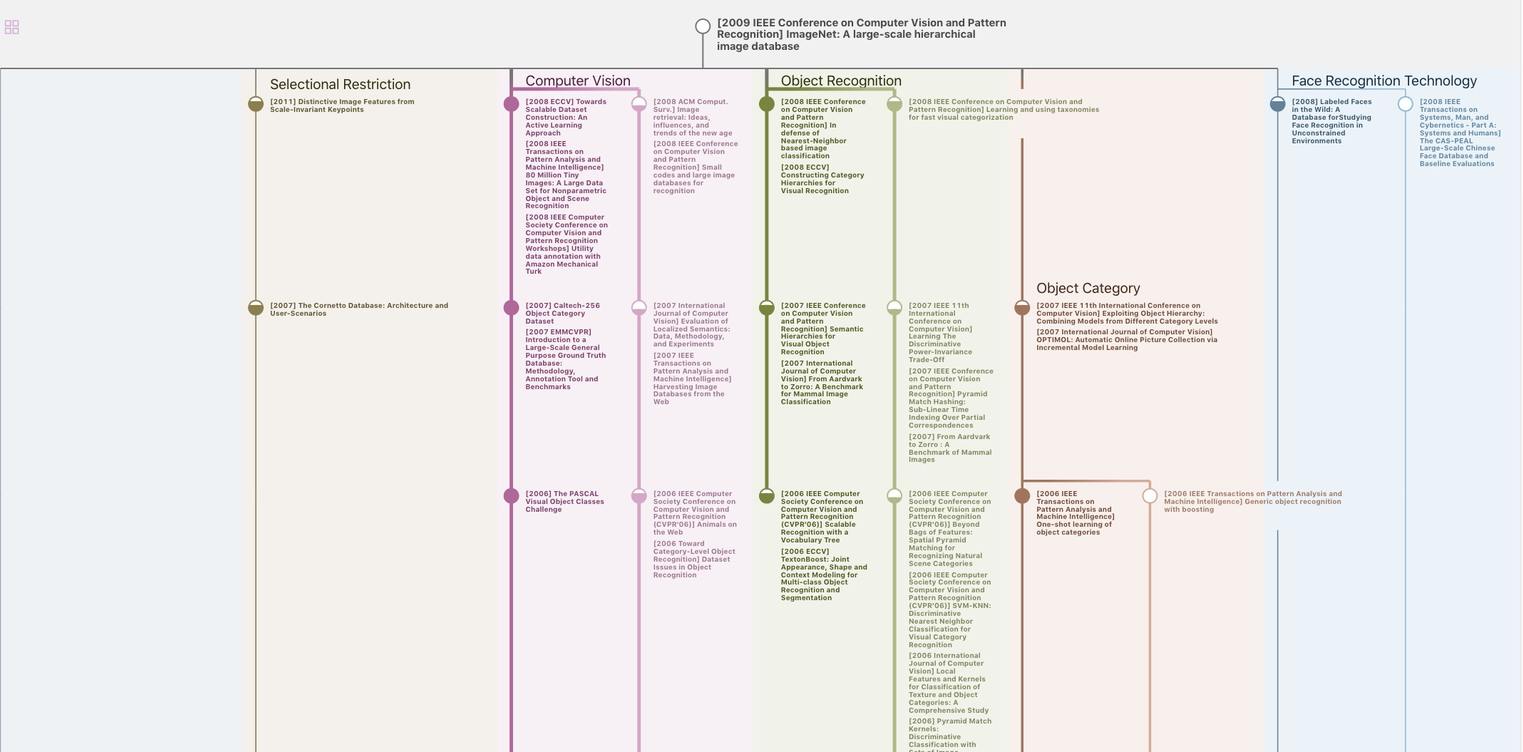
生成溯源树,研究论文发展脉络
Chat Paper
正在生成论文摘要