Dimensionality reduction for machine learning using statistical methods: A case study on predicting mechanical properties of steels
Materials Today Communications(2023)
摘要
Steel manufacturing is a long and complicated process including refining, casting, and rolling; hundreds of process parameters can potentially influence the mechanical properties of final products. This complexity results in significant challenges in correlating input parameters with final mechanical properties. Machine learning models, neural networks and XGBoost, have been used in the prediction of mechanical properties, however, interpretability remains an issue, especially in the case of neural networks. In this study, a statistical method - iGATE is utilised to reduce dimension of inputs in predicting mechanical properties of hot-rolled steel plates. It is found that iGATE can successfully extract the key features and reduce the dimension of inputs while maintaining a high prediction accuracy. With relative errors lower than 5 %, XGboost with full inputs has the best prediction performance. With reduced input dimensions, interference of irrelevant features diminishes, and the ranking of important key features is more reliable. The iGATE methodology offers industry opportunities to identify the key input parameters in terms of materials chemistry and process variables to optimise mechanical properties of rolled plates.
更多查看译文
关键词
dimensionality reduction,machine learning,statistical methods,mechanical properties
AI 理解论文
溯源树
样例
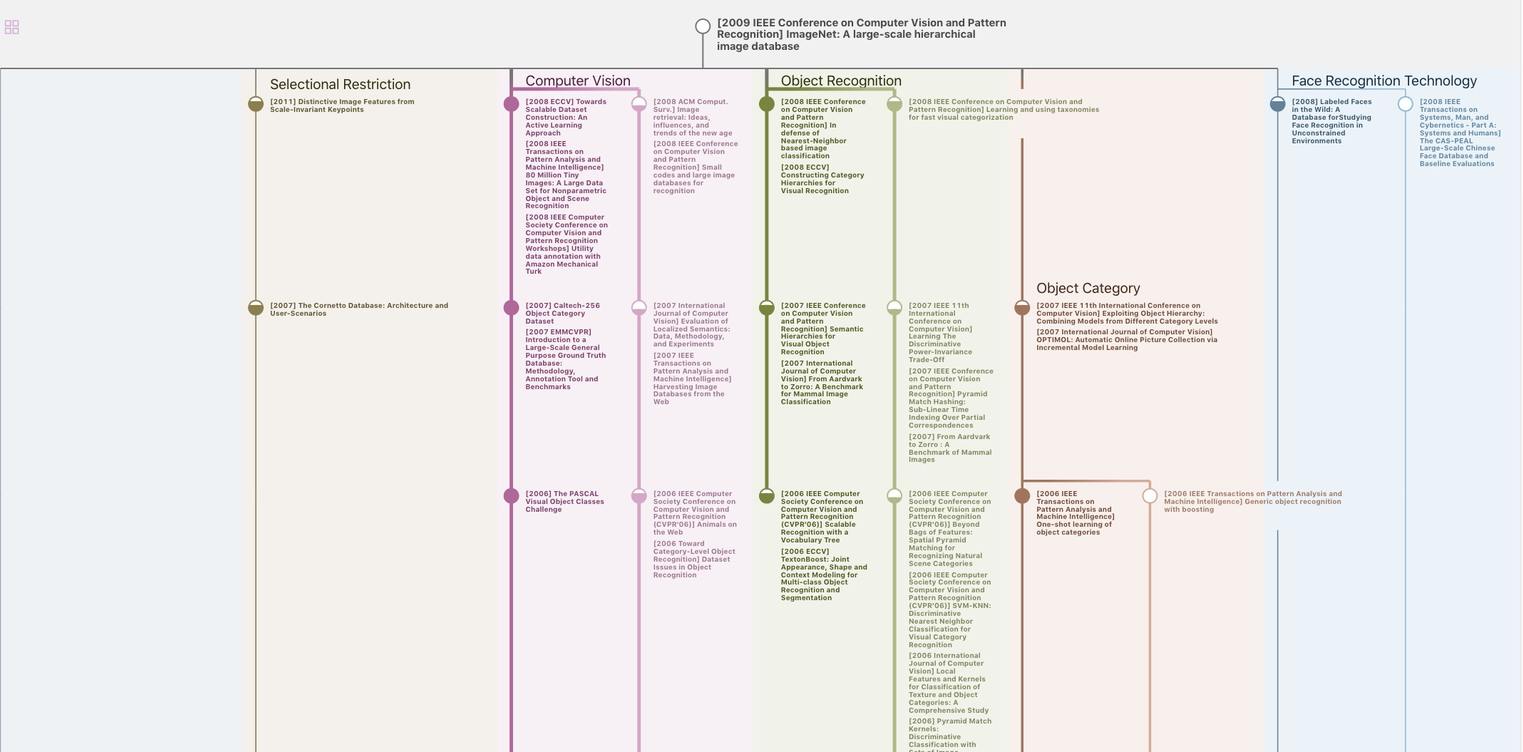
生成溯源树,研究论文发展脉络
Chat Paper
正在生成论文摘要