Self-labeling video prediction
Displays(2023)
摘要
Learning to predict future visual dynamics given input video sequences is a challenging but essential task. Although many stochastic video prediction models are proposed, they still suffer from “multi-modal entanglement”, which refers to the ambiguity of learned representations for multi-modal dynamics modeling. While most existing video prediction models are label-free, we propose a self-supervised labeling strategy to improve spatiotemporal prediction networks without extra supervision. Starting from a set of clustered pseudo-labels, our framework alternates between model optimization and label updating. The key insight of our method lies in that we exploit the reconstruction error from the optimized model itself as an indicator to progressively refine the label assignment on the training set. The two steps are interdependent, with the predictive model guiding the direction of label updates, and in turn, effective pseudo-labels further help the model learn better disentangled multi-modal representation. Experiments on two different video prediction datasets demonstrate the effectiveness of the proposed method.
更多查看译文
关键词
prediction,video,self-labeling
AI 理解论文
溯源树
样例
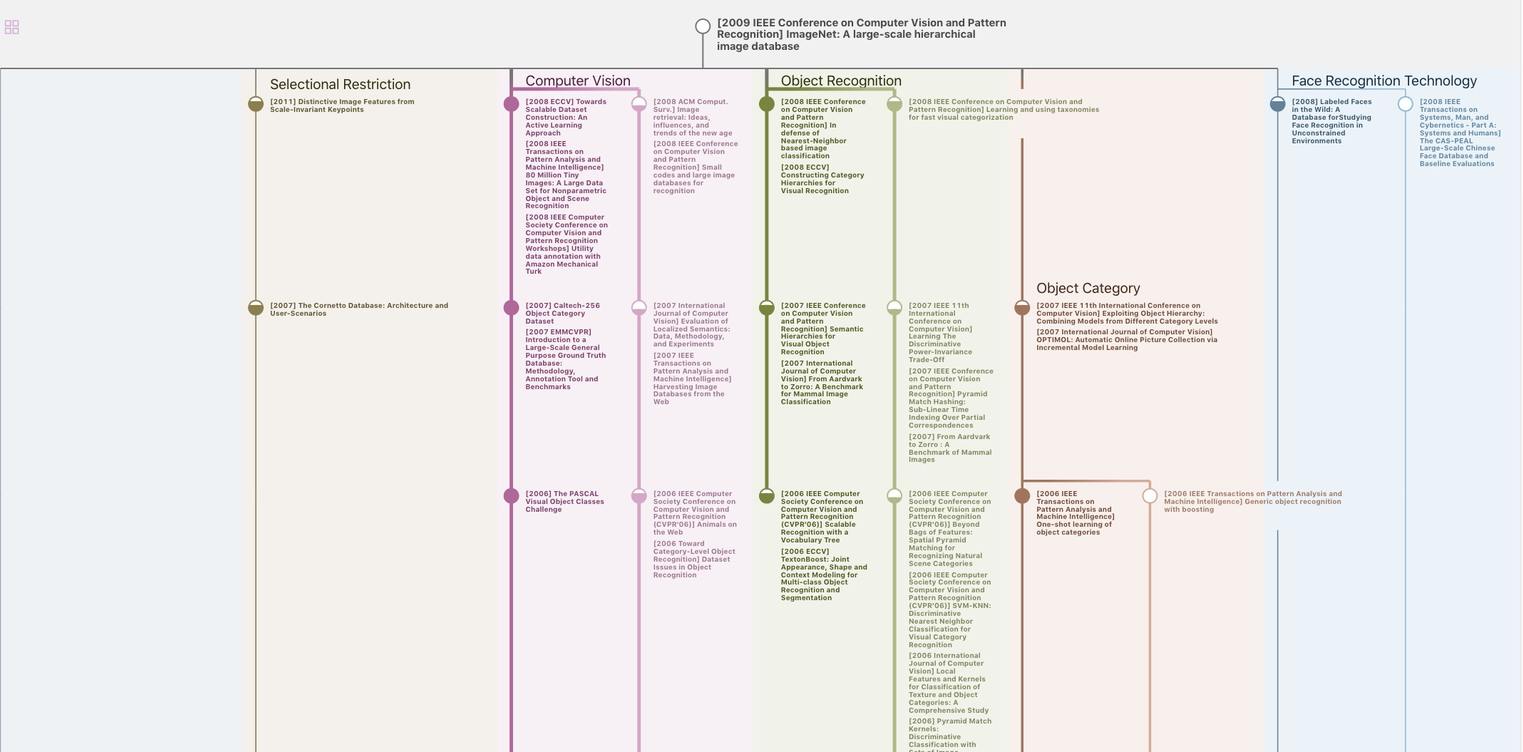
生成溯源树,研究论文发展脉络
Chat Paper
正在生成论文摘要