SOHO-FL: A Fast Reconvergent Intra-domain Routing Scheme Using Federated Learning
IEEE Network(2023)
摘要
The development of machine learning provides a new paradigm for network optimizations, e.g., reinforcement learning (RL) has brought great improvements in many fields, such as adaptive video streaming, congestion control of TCP. The fundamental mechanism of such RL-based architectures is that the neural network decision model converges to a stable state by continuously interacting with network environment. However, for network routing problem, such RL-based strategies do not work well due to topology change. This is because topological changes would require the existing RL models to be retrained, while these models may stop making routing decisions or provide non-optimal decisions during the slow reconverging process of retraining, seriously affected transmission performance. To solve this problem, we proposed a fast convergent RL-model (SOHOFL), which can alleviate the performance degradation caused by the slow retraining process by federated learning. The experimental results based on real-world network topologies demonstrate that SOHO-FL outperforms the state-of-the-art algorithms in reconvergence time by 22.3% on average.
更多查看译文
AI 理解论文
溯源树
样例
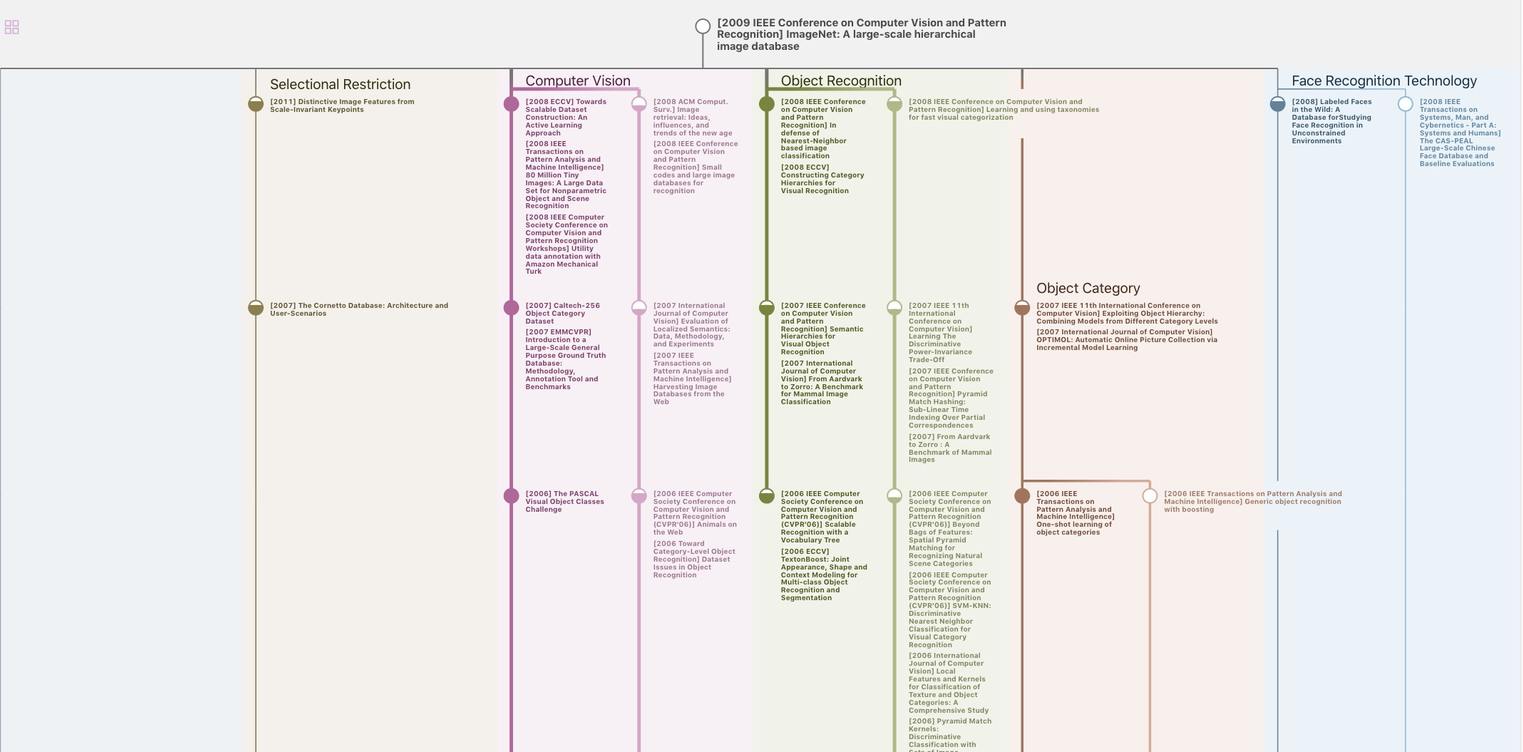
生成溯源树,研究论文发展脉络
Chat Paper
正在生成论文摘要