A Deep Reinforcement Approach for Energy-Efficient Resource Assignment in Cooperative NOMA-Enhanced Cellular Networks
IEEE Internet of Things Journal(2023)
摘要
In this article, an energy efficiency (EE) maximization problem of cooperative nonorthogonal multiple access (CNOMA) network is proposed to jointly determine the user pairing, subchannel assignment, and power control scheme. We decompose it into two steps: in the first step, the optimal closed-form expressions of the power control problem are derived. Based on these, the EE optimization of whole system is formulated as a self-play Go game with the maximum EE as the winner, by constructing a virtual Go board with rows and columns representing indices of users and subchannels, respectively. Each move is to select a position on the Go board (i.e., select a user that has not been assigned to any channel and then assign a channel to it), until all users are assigned on the subchannels. Then, a deep Monte Carlo tree search (MCTS) model is proposed, where an MCTS guided by a neural network simulates multiple possible trajectories to search each move by evaluating its achievable EE reward, while the neural network is trained by the training data generated from the searching of MCTS to predict move selections and also the winner of games. The simulation results show that the proposed method is superior to a variety of conventional schemes in terms of EE in negligible computational time.
更多查看译文
关键词
Cooperative nonorthogonal multiple access (CNOMA),decode-and-forward (DF),deep neural network,deep reinforcement learning (DRL),energy efficiency (EE),full duplex (FD),half duplex (HD),Monte Carlo tree search (MCTS)
AI 理解论文
溯源树
样例
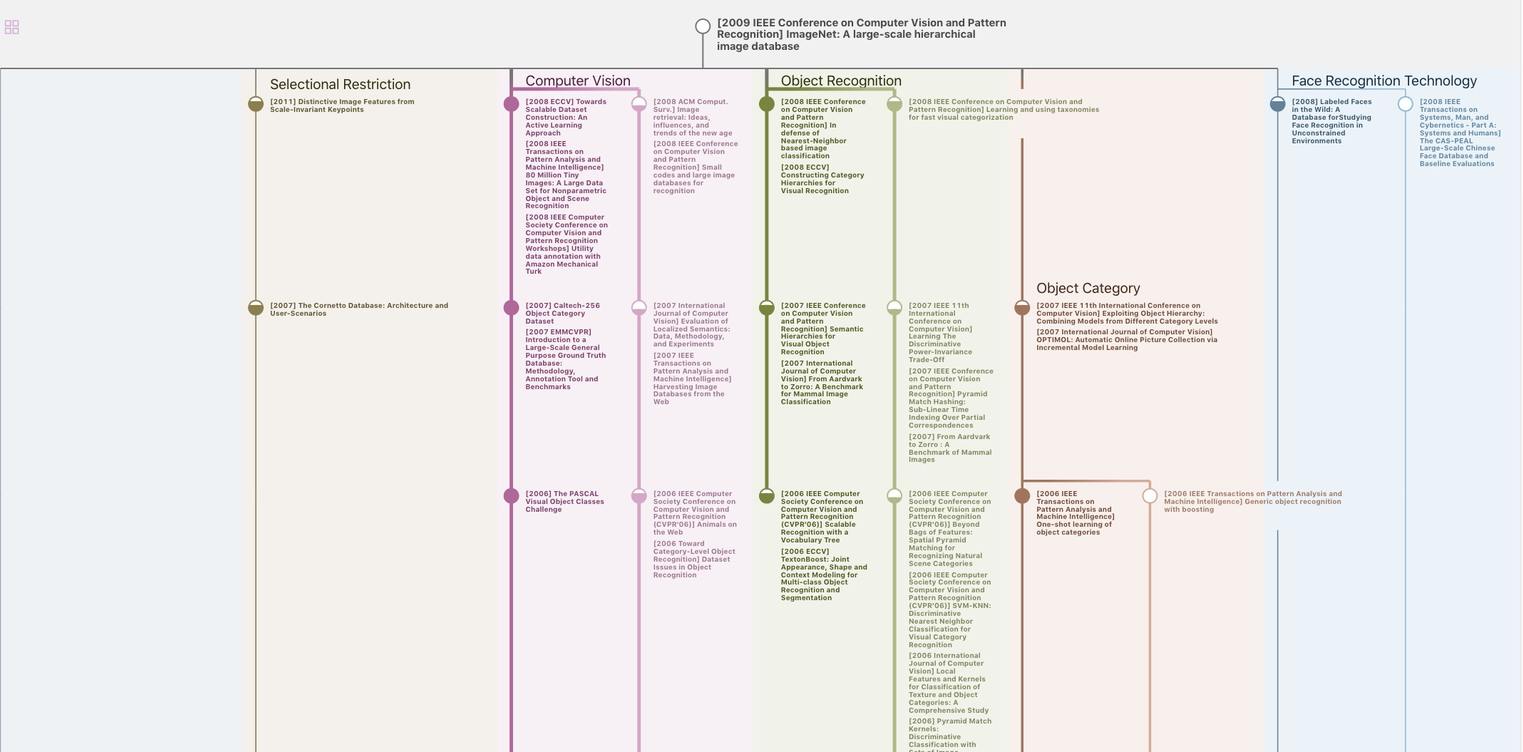
生成溯源树,研究论文发展脉络
Chat Paper
正在生成论文摘要