Deep Cross-Modal Hashing Based on Semantic Consistent Ranking
IEEE TRANSACTIONS ON MULTIMEDIA(2023)
摘要
The amount of multi-modal data available on the Internet is enormous. Cross-modal hash retrieval maps heterogeneous cross-modal data into a single Hamming space to offer fast and flexible retrieval services. However, existing cross-modal methods mainly rely on the feature-level similarity between multi-modal data and ignore the relationship between relative rankings and label-level fine-grained similarity of neighboring instances. To overcome these issues, we propose a novel Deep Cross-modal Hashing based on Semantic Consistent Ranking (DCH-SCR) that comprehensively investigates the intra-modal semantic similarity relationship. Firstly, to the best of our knowledge, it is an early attempt to preserve semantic similarity for cross-modal hashing retrieval by combining label-level and feature-level information. Secondly, the inherent gap between modalities is narrowed by developing a ranking alignment loss function. Thirdly, the compact and efficient hash codes are optimized based on the common semantic space. Finally, we use the gradient to specify the optimization direction and introduce the Normalized Discounted Cumulative Gain (NDCG) to achieve varying optimization strengths for data pairs with different similarities. Extensive experiments on three real-world image-text retrieval datasets demonstrate the superiority of DCH-SCR over several state-of-the-art cross-modal retrieval methods.
更多查看译文
关键词
Cross-modal hashing,rank learning,heterogeneous gap,intra-modal similarity
AI 理解论文
溯源树
样例
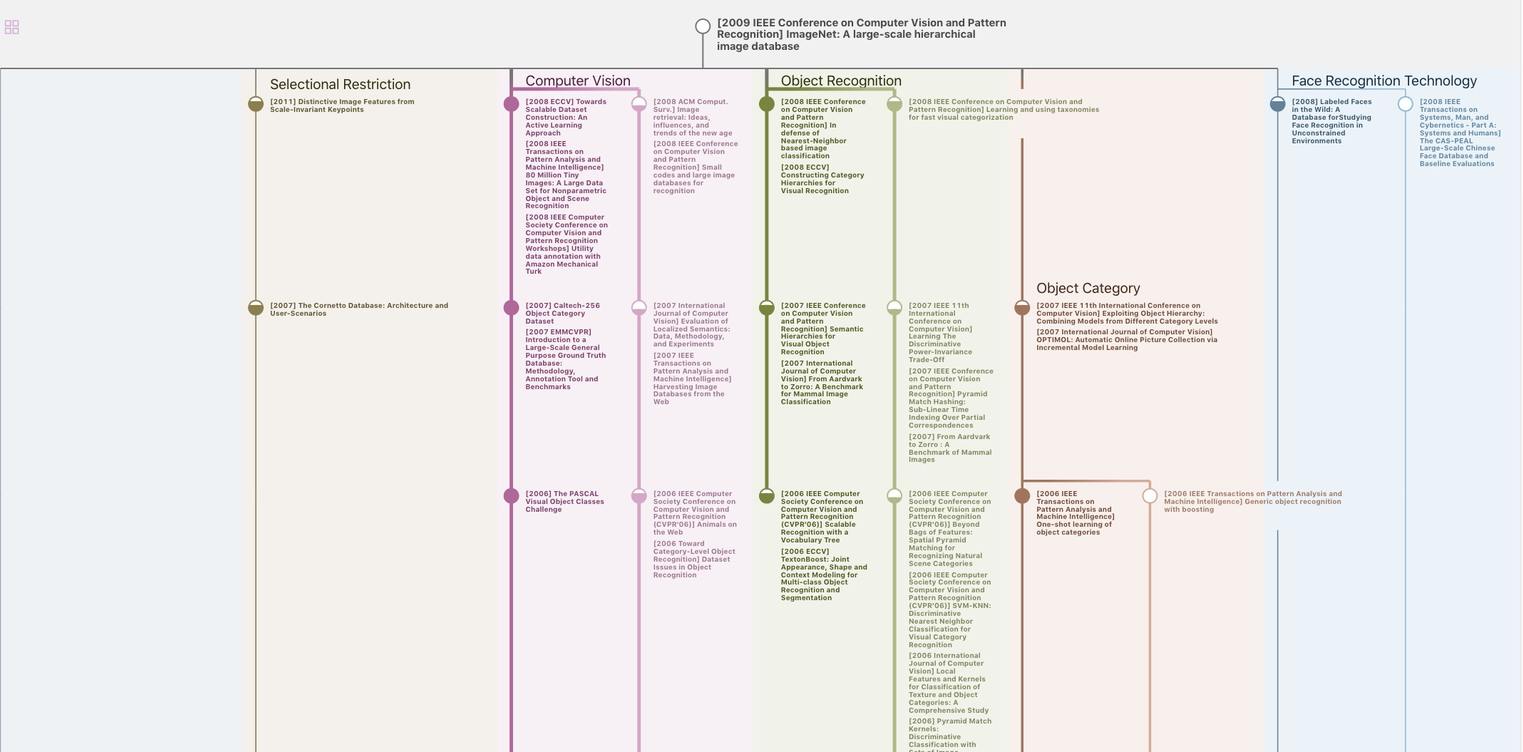
生成溯源树,研究论文发展脉络
Chat Paper
正在生成论文摘要