A Self-Adaptive Learning Approach for Uncertain Disassembly Planning Based on Extended Petri Net
IEEE TRANSACTIONS ON INDUSTRIAL INFORMATICS(2023)
摘要
Disassembly is the first phase to demanufacture end-of-life (EOL) products that are separated into parts/components for recovery. The quality conditions of EOL products are highly uncertain, which would result in some uncertain information during the disassembly process, e.g., the disassembly time and recovering revenue of each subassembly. It is quite challenging to determine the optimal/near-optimal disassembly solutions under uncertain information. This article studies uncertain disassembly planning (UDP) and proposes a self-adaptive learning approach to quickly identify the near-optimal disassembly solutions. First, we model the UDP by extending Petri nets, where not only disassembly operations but also EOL options of each subassembly are represented in the extended Petri Net. Based on the UDP model, we develop the self-adaptive learning approach, which integrates an approximation procedure for estimating uncertain disassembly information, a Q-learning algorithm for training disassembly samples, and a heuristic method for selecting the best disassembly solution. Finally, a hybrid Li-ion battery pack of Audi A3 Sportback e-tron is selected as the case study and applied to test the proposed self-adaptive learning approach. The experimental results demonstrate that our proposed method can efficiently find a better disassembly solution than the existing disassembly solution within 200 trainings in the case study.
更多查看译文
关键词
Petri nets,Costs,Training,Planning,Q-learning,Lithium-ion batteries,Informatics,Extended petri net,hybrid Li-ion battery pack,self-adaptive learning,uncertain disassembly planning (UDP)
AI 理解论文
溯源树
样例
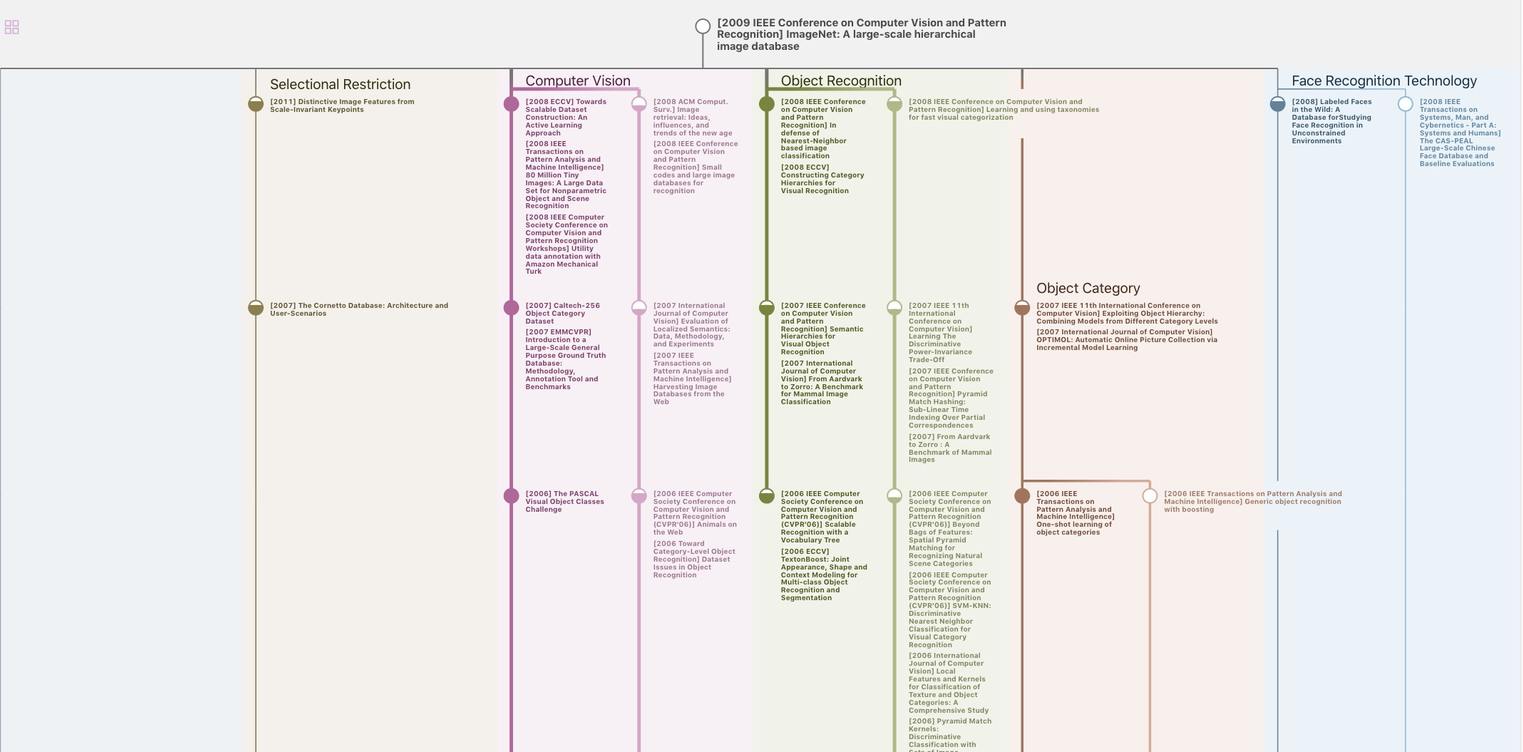
生成溯源树,研究论文发展脉络
Chat Paper
正在生成论文摘要