Dual-aware transformer network for single-image super-resolution
Journal of Electronic Imaging(2023)
摘要
The advent of deep learning has enabled image super-resolution (SR) technology to achieve tremendous success. The recent exploration between convolutional neural network (CNN) and transformer has produced an impressive performance. However, the huge model size and heavy computational cost occupied by most approaches cannot be ignored. Additionally, the global and local modeling of image features is not well utilized and explored, which is important for texture content reconstruction. In this study, we present an efficient and lightweight network based on CNN and transformer for image SR, dubbed dual-aware transformer network (DATN). Specifically, a dual-aware fusion module (DAFM), consisting of spatial-aware adaptive block (SAAB) and global-aware fusion block (GAFB), learns local and global features in a complementary manner for yielding abundant content details. SAAB delivers the captured spatial information to GAFB in parallel, whereas GAFB establishes long-range spatial dependencies among diverse features to boost the network's ability for recovering texture details. Further, in the upsampling stage, our proposed transformer-empowered upsampling module aggregates global information from the transformer module to generate location-wise reassembly kernels for content-aware upsampling. In such way, feature-rich information is highlighted adaptively to reconstruct high-quality images with maximum efficiency. Experimental results reveal that our proposed DATN achieves high qualitative performance while maintaining low computational demands, surpassing most state-of-the-art SR networks.
更多查看译文
关键词
dual-aware,single-image,super-resolution
AI 理解论文
溯源树
样例
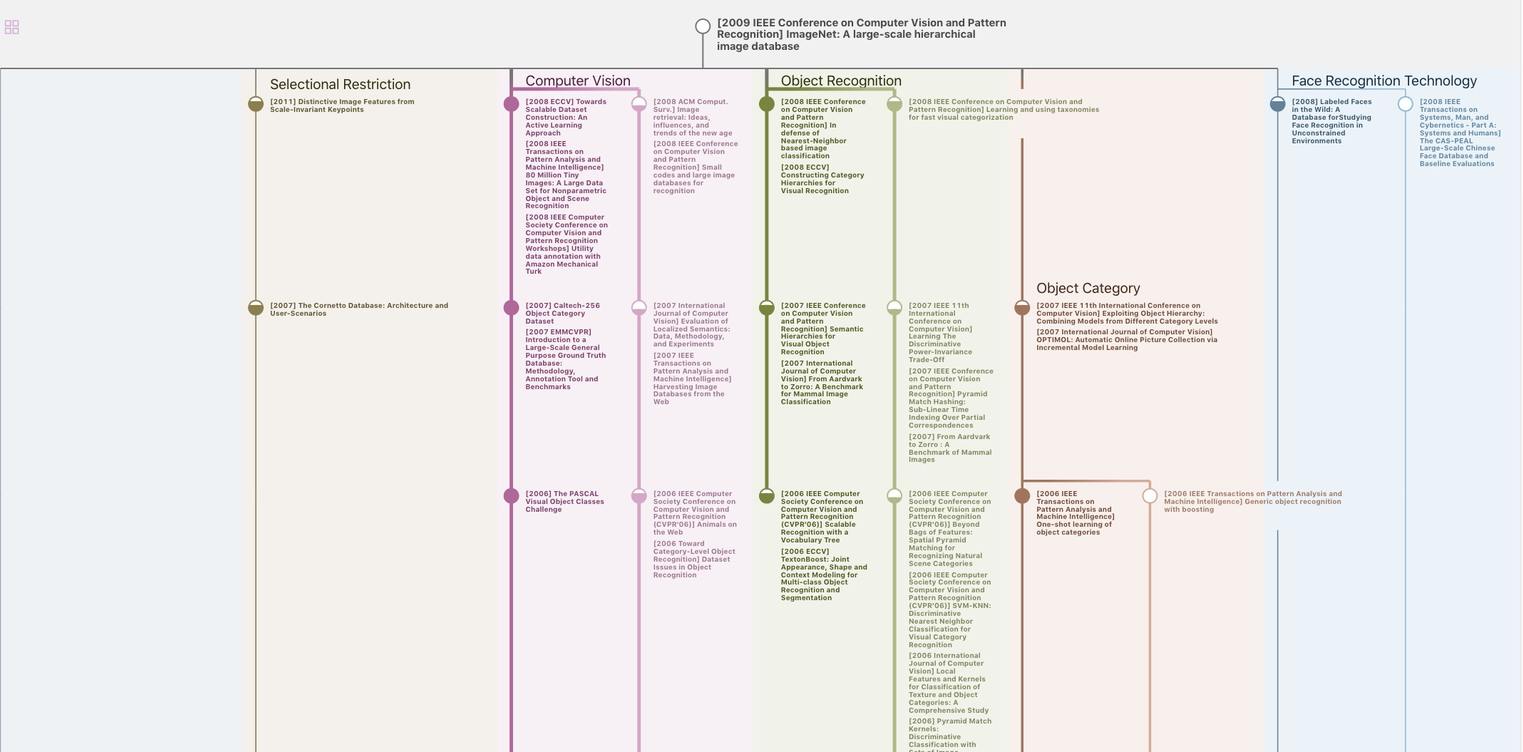
生成溯源树,研究论文发展脉络
Chat Paper
正在生成论文摘要