Average Gradient-Based Adversarial Attack
IEEE TRANSACTIONS ON MULTIMEDIA(2023)
摘要
Deep neural networks (DNNs) are vulnerable to adversarial attacks which can fool the classifiers by adding small perturbations to the original example. The added perturbations in most existing attacks are mainly determined by the gradient of the loss function with respect to the current example. In this paper, a new average gradient-based adversarial attack is proposed. In our proposed method, via utilizing the gradient of each iteration in the past, a dynamic set of adversarial examples is constructed first in each iteration. Then, according to the gradient of the loss function with respect to all the examples in the constructed dynamic set and the current adversarial example, the average gradient can be calculated, which is used to determine the added perturbations. Different from the existing adversarial attacks, the proposed average gradient-based attack optimizes the added perturbations through a dynamic set of adversarial examples, where the size of the dynamic set increases with the number of iterations. Our proposed method possesses good extensibility and can be integrated into most existing gradient-based attacks. Extensive experiments demonstrate that, compared with the state-of-the-art gradient-based adversarial attacks, the proposed attack can achieve higher attack success rates and exhibit better transferability, which is helpful to evaluate the robustness of the network and the effectiveness of the defense method.
更多查看译文
关键词
Adversarial attack,black-box attack,dynamic set of adversarial examples,transferability
AI 理解论文
溯源树
样例
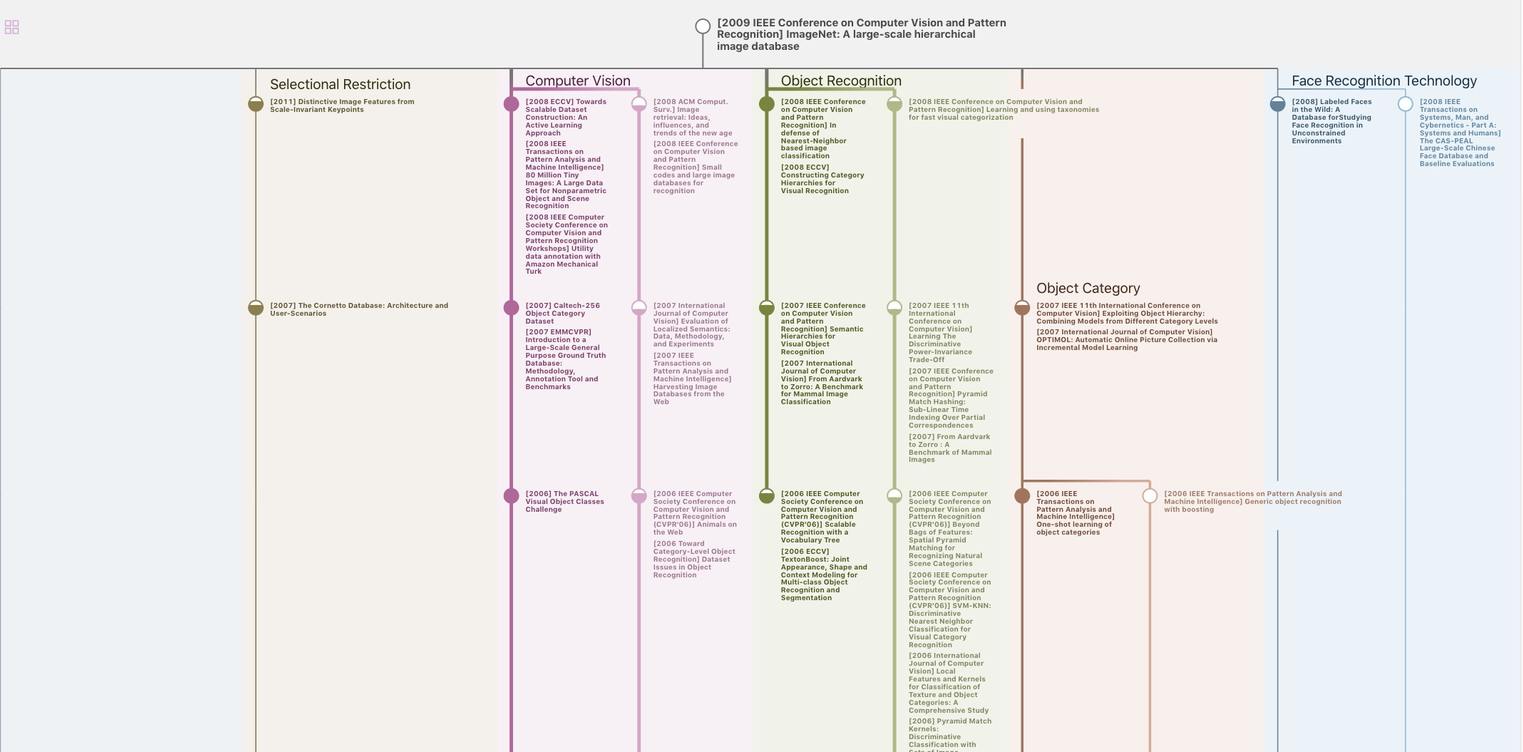
生成溯源树,研究论文发展脉络
Chat Paper
正在生成论文摘要