MAE-BG: Dual-stream Boundary Optimization for Remote Sensing Image Semantic Segmentation
Geocarto International(2023)
摘要
Deep learning has achieved remarkable performance in semantically segmenting remotely sensed images. However, the high-frequency detail loss caused by continuous convolution and pooling operations and the uncertainty introduced when annotating low-contrast objects with weak boundaries induce blurred object boundaries. Therefore, a dual-stream network MAE-BG, consisting of an edge detection (ED) branch and a smooth branch with boundary guidance (BG), is proposed. The ED branch is designed to enhance the weak edges that need to be preserved, simultaneously suppressing false responses caused by local texture. This mechanism is achieved by introducing improved multiple-attention edge detection blocks (MAE). Furthermore, two specific ED branches with MAE are designed to combine with typical deep convolutional (DC) and Codec infrastructures and result in two configurations of MAE-A and MAE-B. Meanwhile, multiscale edge information extracted by MAE networks is fed into the backbone networks to complement the detail loss caused by convolution and pooling operations. This results in smooth networks with BG. After that, the segmentation results with improved boundaries are obtained by stacking the output of the ED and smooth branches. The proposed algorithms were evaluated on the ISPRS Potsdam and Inria Aerial Image Labelling datasets. Comprehensive experiments show that the proposed method can precisely locate object boundaries and improve segmentation performance. The MAE-A branch leads to an overall accuracy (OA) of 89.16%, a mean intersection over union (MIOU) of 80.25% for Potsdam, and an OA of 96.61% and MIOU of 86.63% for Inria. Compared with the results without the proposed edge optimization blocks, the OAs from the Potsdam and Inria datasets increase by 5.49% and 7.64%, respectively.
更多查看译文
关键词
deep learning,boundary optimization,squeeze and excitation,semantic segmentation,remote sensing
AI 理解论文
溯源树
样例
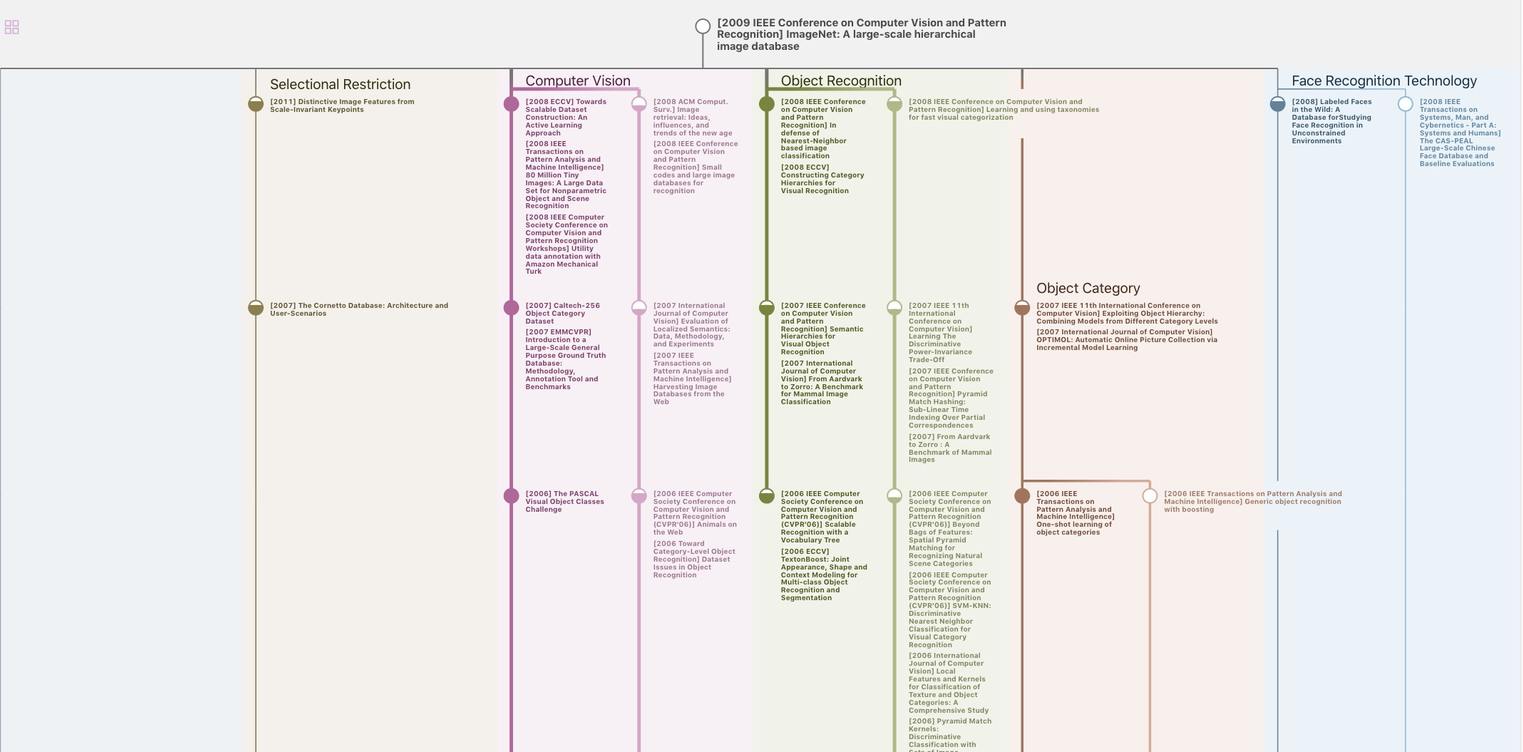
生成溯源树,研究论文发展脉络
Chat Paper
正在生成论文摘要