Identify causality by multi-scale structural complexity
PHYSICA A-STATISTICAL MECHANICS AND ITS APPLICATIONS(2024)
摘要
Identifying causality between variables is essential to reveal their interactions. In previous studies, causality between variables has been captured at specific scales. However, it was not taken into account the difference in causality between different scales. In other words, it remains an open question about which scale of causality contributes most to correct causality. In this paper, a multi-scale structural complexity method of bivariate time series was developed from the recent advances in measuring the complexity of visual images. This method can identify the direction and strength of causality between variables by total complexity. In addition, depending on the contribution from the local complexity of each scale to the total complexity, it determines which scaling scale(i.e., levels of resolution) is responsible for causality. Our results indicated that local complexity at the medium scale contributes the most to total complexity. More interestingly, this conclusion holds not just for synthetic data but also for empirical data. Consequently, our finding provides not only a novel perspective to reveal the causality between variables from the perspective of complexity, but also a reference to choose an appropriate scale to study the relationship between variables.
更多查看译文
关键词
Causality,Multi-scale structural complexity,Synthetic data,Empirical data
AI 理解论文
溯源树
样例
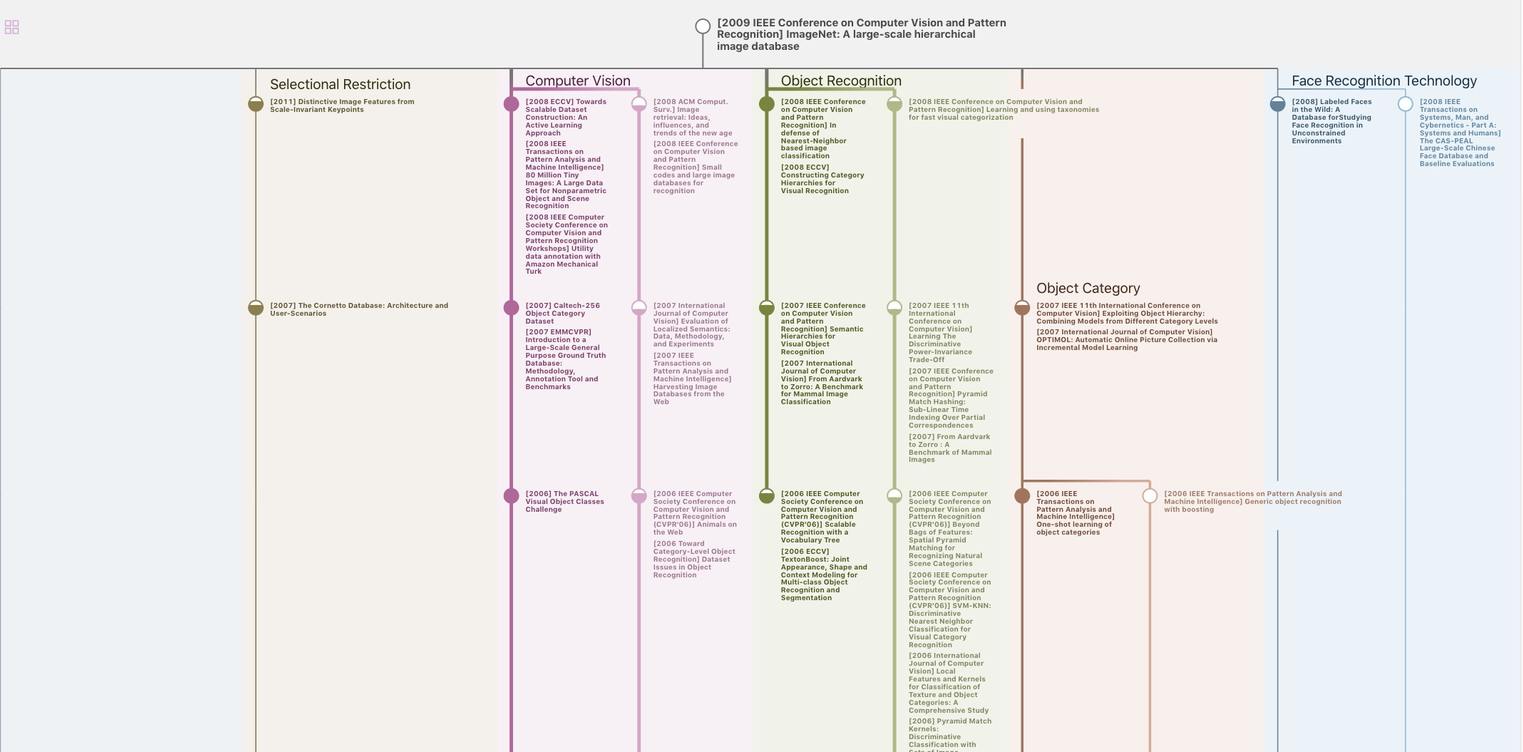
生成溯源树,研究论文发展脉络
Chat Paper
正在生成论文摘要